4M Tokens? MiniMax-Text-01 Outperforms DeepSeek V3
Chinese AI is making significant strides, challenging leading models like GPT-4, Claude, and Grok with cost-effective, open-source alternatives such as DeepSeek-V3 and Qwen 2.5. These models excel due to their efficiency, accessibility, and strong performance. Many operate under permissive commercial licenses, broadening their appeal to developers and businesses.
MiniMax-Text-01, the newest addition to this group, sets a new standard with its unprecedented 4 million token context length—vastly surpassing the typical 128K-256K token limit. This extended context capability, combined with a Hybrid Attention architecture for efficiency and an open-source, commercially permissive license, fosters innovation without high costs.
Let's delve into MiniMax-Text-01's features:
Table of Contents
- Hybrid Architecture
- Mixture-of-Experts (MoE) Strategy
- Training and Scaling Strategies
- Post-Training Optimization
- Key Innovations
- Core Academic Benchmarks
- General Tasks Benchmarks
- Reasoning Tasks Benchmarks
- Mathematics & Coding Tasks Benchmarks
- Getting Started with MiniMax-Text-01
- Important Links
- Conclusion
Hybrid Architecture
MiniMax-Text-01 cleverly balances efficiency and performance by integrating Lightning Attention, Softmax Attention, and Mixture-of-Experts (MoE).
- 7/8 Linear Attention (Lightning Attention-2): This linear attention mechanism drastically reduces computational complexity from O(n²d) to O(d²n), ideal for long-context processing. It uses SiLU activation for input transformation, matrix operations for attention score calculation, and RMSNorm and sigmoid for normalization and scaling.
- 1/8 Softmax Attention: A traditional attention mechanism, incorporating RoPE (Rotary Position Embedding) on half the attention head dimension, enabling length extrapolation without sacrificing performance.
Mixture-of-Experts (MoE) Strategy
MiniMax-Text-01's unique MoE architecture distinguishes it from models like DeepSeek-V3:
- Token Drop Strategy: Employs an auxiliary loss to maintain balanced token distribution across experts, unlike DeepSeek's dropless approach.
- Global Router: Optimizes token allocation for even workload distribution among expert groups.
- Top-k Routing: Selects the top-2 experts per token (compared to DeepSeek's top-8 1 shared expert).
- Expert Configuration: Utilizes 32 experts (vs. DeepSeek's 256 1 shared), with an expert hidden dimension of 9216 (vs. DeepSeek's 2048). The total activated parameters per layer remain the same as DeepSeek (18,432).
Training and Scaling Strategies
- Training Infrastructure: Leveraged approximately 2000 H100 GPUs, employing advanced parallelism techniques like Expert Tensor Parallelism (ETP) and Linear Attention Sequence Parallelism Plus (LASP ). Optimized for 8-bit quantization for efficient inference on 8x80GB H100 nodes.
- Training Data: Trained on roughly 12 trillion tokens using a WSD-like learning rate schedule. The data comprised a blend of high- and low-quality sources, with global deduplication and 4x repetition for high-quality data.
- Long-Context Training: A three-phased approach: Phase 1 (128k context), Phase 2 (512k context), and Phase 3 (1M context), using linear interpolation to manage distribution shifts during context length scaling.
Post-Training Optimization
- Iterative Fine-Tuning: Cycles of Supervised Fine-Tuning (SFT) and Reinforcement Learning (RL), using Offline DPO and Online GRPO for alignment.
- Long-Context Fine-Tuning: A phased approach: Short-Context SFT → Long-Context SFT → Short-Context RL → Long-Context RL, crucial for superior long-context performance.
Key Innovations
- DeepNorm: A post-norm architecture enhancing residual connection scaling and training stability.
- Batch Size Warmup: Gradually increases batch size from 16M to 128M tokens for optimal training dynamics.
- Efficient Parallelism: Utilizes Ring Attention to minimize memory overhead for long sequences and padding optimization to reduce wasted computation.
Core Academic Benchmarks
(Tables showing benchmark results for General Tasks, Reasoning Tasks, and Mathematics & Coding Tasks are included here, mirroring the original input's tables.)
(Additional evaluation parameters link remains)
Getting Started with MiniMax-Text-01
(Code example for using MiniMax-Text-01 with Hugging Face transformers remains the same.)
Important Links
- Chatbot
- Online API
- Documentation
Conclusion
MiniMax-Text-01 demonstrates impressive capabilities, achieving state-of-the-art performance in long-context and general-purpose tasks. While areas for improvement exist, its open-source nature, cost-effectiveness, and innovative architecture make it a significant player in the AI field. It's particularly suitable for memory-intensive and complex reasoning applications, though further refinement for coding tasks may be beneficial.
The above is the detailed content of 4M Tokens? MiniMax-Text-01 Outperforms DeepSeek V3. For more information, please follow other related articles on the PHP Chinese website!

Hot AI Tools

Undresser.AI Undress
AI-powered app for creating realistic nude photos

AI Clothes Remover
Online AI tool for removing clothes from photos.

Undress AI Tool
Undress images for free

Clothoff.io
AI clothes remover

Video Face Swap
Swap faces in any video effortlessly with our completely free AI face swap tool!

Hot Article

Hot Tools

Notepad++7.3.1
Easy-to-use and free code editor

SublimeText3 Chinese version
Chinese version, very easy to use

Zend Studio 13.0.1
Powerful PHP integrated development environment

Dreamweaver CS6
Visual web development tools

SublimeText3 Mac version
God-level code editing software (SublimeText3)

Hot Topics










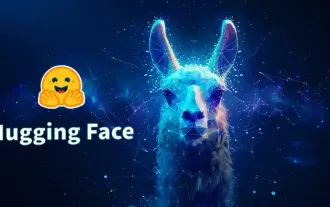
Meta's Llama 3.2: A Leap Forward in Multimodal and Mobile AI Meta recently unveiled Llama 3.2, a significant advancement in AI featuring powerful vision capabilities and lightweight text models optimized for mobile devices. Building on the success o
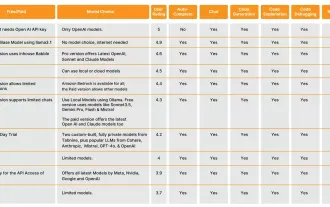
Hey there, Coding ninja! What coding-related tasks do you have planned for the day? Before you dive further into this blog, I want you to think about all your coding-related woes—better list those down. Done? – Let’
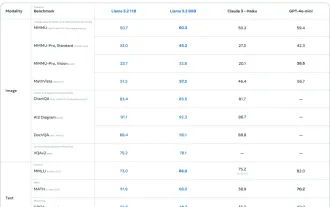
This week's AI landscape: A whirlwind of advancements, ethical considerations, and regulatory debates. Major players like OpenAI, Google, Meta, and Microsoft have unleashed a torrent of updates, from groundbreaking new models to crucial shifts in le
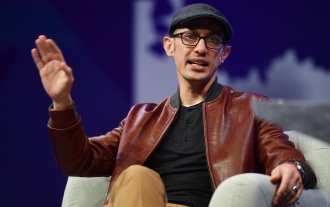
Shopify CEO Tobi Lütke's recent memo boldly declares AI proficiency a fundamental expectation for every employee, marking a significant cultural shift within the company. This isn't a fleeting trend; it's a new operational paradigm integrated into p
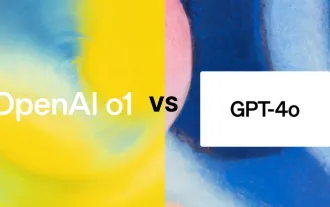
Introduction OpenAI has released its new model based on the much-anticipated “strawberry” architecture. This innovative model, known as o1, enhances reasoning capabilities, allowing it to think through problems mor
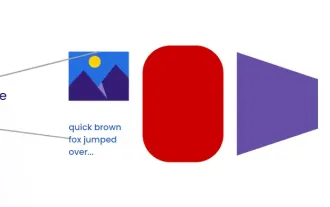
Introduction Imagine walking through an art gallery, surrounded by vivid paintings and sculptures. Now, what if you could ask each piece a question and get a meaningful answer? You might ask, “What story are you telling?
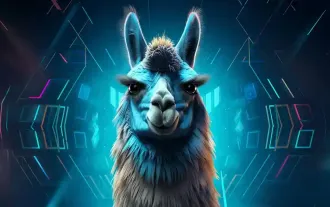
Meta's Llama 3.2: A Multimodal AI Powerhouse Meta's latest multimodal model, Llama 3.2, represents a significant advancement in AI, boasting enhanced language comprehension, improved accuracy, and superior text generation capabilities. Its ability t
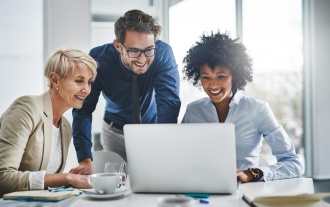
For those of you who might be new to my column, I broadly explore the latest advances in AI across the board, including topics such as embodied AI, AI reasoning, high-tech breakthroughs in AI, prompt engineering, training of AI, fielding of AI, AI re
