Bitcoin Price Prediction Using MLops
Don’t know much about Bitcoin or its price fluctuations but want to make investment decisions to make profits? This machine learning model has your back. It can predict the prices way better than an astrologer. In this article, we will build an ML model for forecasting and predicting Bitcoin price, using ZenML and MLflow. So let’s start our journey to understand how anyone can use ML and MLOps tools to predict the future.
Learning Objectives
- Learn to fetch live data using API efficiently.
- Understand what ZenML is, why we use MLflow, and how you can integrate it with ZenML.
- Explore the deployment process for machine learning models, from idea to production.
- Discover how to create a user-friendly Streamlit app for interactive machine-learning model predictions.
This article was published as a part of theData Science Blogathon.
Table of Contents
- Problem Statement
- Project Implementation
- Step 1: Accessing the API
- Step 2: Connecting to Database Using MongoDB
- Step 3: Integration of MLflow with ZenML
- Step 4: Data Ingestion
- Step 5: Data Cleaning
- Step 6: Feature Engineering
- Step 7: Data Splitting
- Step 8: Model Training
- Step 9: Model Evaluation
- Step 10: Model Deployment
- Step 11: Building the Streamlit App
- Frequently Asked Questions
Problem Statement
Bitcoin prices are highly volatile, and making predictions is next to impossible. In our project, we are usingMLOps’ best practicesto build an LSTM model to forecast Bitcoinprices and trends.
Before implementing the project let’s look at the project architecture.
Project Implementation
Let’s begin by accessing the API.
Why are we doing this? You can get historical Bitcoin price data from different datasets, but with an API, we can have access to live market data.
Step 1: Accessing the API
-
Sign up for API Access:
Once you sign up on theCCData API page. You can get the free API key from this pagehttps://developers.cryptocompare.com/documentation/data-api/index_cc_v1_historical_days
-
Fetch Bitcoin Price Data:
With the code below, you can fetch Bitcoin price data from the CCData API and convert it into a pandas DataFrame. Also, keep the API key in the .env file.
import requests import pandas as pd from dotenv import load_dotenv import os # Load the .env file load_dotenv() def fetch_crypto_data(api_uri): response = requests.get( api_uri, params={ "market": "cadli", "instrument": "BTC-USD", "limit": 5000, "aggregate": 1, "fill": "true", "apply_mapping": "true", "response_format": "JSON" }, headers={"Content-type": "application/json; charset=UTF-8"} ) if response.status_code == 200: print('API Connection Successful! \nFetching the data...') data = response.json() data_list = data.get('Data', []) df = pd.DataFrame(data_list) df['DATE'] = pd.to_datetime(df['TIMESTAMP'], unit='s') return df # Return the DataFrame else: raise Exception(f"API Error: {response.status_code} - {response.text}")
Step 2: Connecting to Database Using MongoDB
MongoDB is a NoSQL database known for its adaptability, expandability, and ability to store unstructured data in a JSON-like format.
import requests import pandas as pd from dotenv import load_dotenv import os # Load the .env file load_dotenv() def fetch_crypto_data(api_uri): response = requests.get( api_uri, params={ "market": "cadli", "instrument": "BTC-USD", "limit": 5000, "aggregate": 1, "fill": "true", "apply_mapping": "true", "response_format": "JSON" }, headers={"Content-type": "application/json; charset=UTF-8"} ) if response.status_code == 200: print('API Connection Successful! \nFetching the data...') data = response.json() data_list = data.get('Data', []) df = pd.DataFrame(data_list) df['DATE'] = pd.to_datetime(df['TIMESTAMP'], unit='s') return df # Return the DataFrame else: raise Exception(f"API Error: {response.status_code} - {response.text}")
This code connects to MongoDB, retrieves Bitcoin price data through an API, and updates the database with all new entries after the latest logged date.
Introducing ZenML
ZenMLis an open-source platform tailored for machine learning operations, supporting the creation of flexible and production-ready pipelines. Additionally, ZenML integrates with multiple machine learning tools likeMLflow, BentoML, etc., to create seamless ML pipelines.
⚠️ If you are a Windows user, try to install wsl on your system. Zenml does not support Windows.
In this project, we will implement a traditional pipeline, which uses ZenML, and we will be integrating MLflow with ZenML, for experiment tracking.
Pre-requisites and Basic ZenML Commands
- Python 3.12 or higher: You can get it from here:https://www.python.org/downloads/
- Activate your Virtual Environment:
import os from pymongo import MongoClient from dotenv import load_dotenv from data.management.api import fetch_crypto_data # Import the API function import pandas as pd load_dotenv() MONGO_URI = os.getenv("MONGO_URI") API_URI = os.getenv("API_URI") client = MongoClient(MONGO_URI, ssl=True, ssl_certfile=None, ssl_ca_certs=None) db = client['crypto_data'] collection = db['historical_data'] try: latest_entry = collection.find_one(sort=[("DATE", -1)]) # Find the latest date if latest_entry: last_date = pd.to_datetime(latest_entry['DATE']).strftime('%Y-%m-%d') else: last_date = '2011-03-27' # Default start date if MongoDB is empty print(f"Fetching data starting from {last_date}...") new_data_df = fetch_crypto_data(API_URI) if latest_entry: new_data_df = new_data_df[new_data_df['DATE'] > last_date] if not new_data_df.empty: data_to_insert = new_data_df.to_dict(orient='records') result = collection.insert_many(data_to_insert) print(f"Inserted {len(result.inserted_ids)} new records into MongoDB.") else: print("No new data to insert.") except Exception as e: print(f"An error occurred: {e}")
- ZenML Commands:
All the core ZenML Commands along with their functionalities are provided below:
#create a virtual environment python3 -m venv venv #Activate your virtual environmnent in your project folder source venv/bin/activate
Step 3: Integration of MLflow with ZenML
We are using MLflow for experiment tracking, to track our model, artifacts, metrics, and hyperparameter values. We are registering MLflow for experiment tracking and model deployer here:
#Install zenml pip install zenml #To Launch zenml server and dashboard locally pip install "zenml[server]" #To check the zenml Version: zenml version #To initiate a new repository zenml init #To run the dashboard locally: zenml login --local #To know the status of our zenml Pipelines zenml show #To shutdown the zenml server zenml clean
ZenML Stack List
Project Structure
Here, you can see the layout of the project. Now let’s discuss it one by one in great detail.
#Integrating mlflow with ZenML zenml integration install mlflow -y #Register the experiment tracker zenml experiment-tracker register mlflow_tracker --flavor=mlflow #Registering the model deployer zenml model-deployer register mlflow --flavor=mlflow #Registering the stack zenml stack register local-mlflow-stack-new -a default -o default -d mlflow -e mlflow_tracker --set #To view the stack list zenml stack --list
Step 4: Data Ingestion
We first ingest data from API to MongoDB and convert it into pandas DataFrame.
bitcoin_price_prediction_mlops/ # Project directory ├── data/ │ └── management/ │ ├── api_to_mongodb.py # Code to fetch data and save it to MongoDB │ └── api.py # API-related utility functions │ ├── pipelines/ │ ├── deployment_pipeline.py # Deployment pipeline │ └── training_pipeline.py # Training pipeline │ ├── saved_models/ # Directory for storing trained models ├── saved_scalers/ # Directory for storing scalers used in data preprocessing │ ├── src/ # Source code │ ├── data_cleaning.py # Data cleaning and preprocessing │ ├── data_ingestion.py # Data ingestion │ ├── data_splitter.py # Data splitting │ ├── feature_engineering.py # Feature engineering │ ├── model_evaluation.py # Model evaluation │ └── model_training.py # Model training │ ├── steps/ # ZenML steps │ ├── clean_data.py # ZenML step for cleaning data │ ├── data_splitter.py # ZenML step for data splitting │ ├── dynamic_importer.py # ZenML step for importing dynamic data │ ├── feature_engineering.py # ZenML step for feature engineering │ ├── ingest_data.py # ZenML step for data ingestion │ ├── model_evaluation.py # ZenML step for model evaluation │ ├── model_training.py # ZenML step for training the model │ ├── prediction_service_loader.py # ZenML step for loading prediction services │ ├── predictor.py # ZenML step for prediction │ └── utils.py # Utility functions for steps │ ├── .env # Environment variables file ├── .gitignore # Git ignore file │ ├── app.py # Streamlit user interface app │ ├── README.md # Project documentation ├── requirements.txt # List of required packages ├── run_deployment.py # Code for running deployment and prediction pipeline ├── run_pipeline.py # Code for running training pipeline └── .zen/ # ZenML directory (created automatically after ZenML initialization)
we add @step as a decorator to the ingest_data() function to declare it as a step of our training pipeline. In the same way, we will write code for each step in the project architecture and create the pipeline.
To view how I have used the @step decorator, check out the GitHub link below (steps folder) to go through the code for other steps of the pipeline i.e. data cleaning, feature engineering, data splitting, model training, and model evaluation.
Step 5: Data Cleaning
In this step, we will create different strategies for cleaning the ingested data. We will drop the unwanted columns and missing values in the data.
import requests import pandas as pd from dotenv import load_dotenv import os # Load the .env file load_dotenv() def fetch_crypto_data(api_uri): response = requests.get( api_uri, params={ "market": "cadli", "instrument": "BTC-USD", "limit": 5000, "aggregate": 1, "fill": "true", "apply_mapping": "true", "response_format": "JSON" }, headers={"Content-type": "application/json; charset=UTF-8"} ) if response.status_code == 200: print('API Connection Successful! \nFetching the data...') data = response.json() data_list = data.get('Data', []) df = pd.DataFrame(data_list) df['DATE'] = pd.to_datetime(df['TIMESTAMP'], unit='s') return df # Return the DataFrame else: raise Exception(f"API Error: {response.status_code} - {response.text}")
Step 6: Feature Engineering
This step takes the cleaned data from the earlier data_cleaning step. We are creating new features like Simple Moving Average (SMA), Exponential Moving Average (EMA), and lagged and rolling statistics to capture trends, reduce noise, and make more reliable predictions from time-series data. Additionally, we scale the features and target variables using Minmax scaling.
import os from pymongo import MongoClient from dotenv import load_dotenv from data.management.api import fetch_crypto_data # Import the API function import pandas as pd load_dotenv() MONGO_URI = os.getenv("MONGO_URI") API_URI = os.getenv("API_URI") client = MongoClient(MONGO_URI, ssl=True, ssl_certfile=None, ssl_ca_certs=None) db = client['crypto_data'] collection = db['historical_data'] try: latest_entry = collection.find_one(sort=[("DATE", -1)]) # Find the latest date if latest_entry: last_date = pd.to_datetime(latest_entry['DATE']).strftime('%Y-%m-%d') else: last_date = '2011-03-27' # Default start date if MongoDB is empty print(f"Fetching data starting from {last_date}...") new_data_df = fetch_crypto_data(API_URI) if latest_entry: new_data_df = new_data_df[new_data_df['DATE'] > last_date] if not new_data_df.empty: data_to_insert = new_data_df.to_dict(orient='records') result = collection.insert_many(data_to_insert) print(f"Inserted {len(result.inserted_ids)} new records into MongoDB.") else: print("No new data to insert.") except Exception as e: print(f"An error occurred: {e}")
Step 7: Data Splitting
Now, we split the processed data into training and testing datasets in the ratio of 80:20.
#create a virtual environment python3 -m venv venv #Activate your virtual environmnent in your project folder source venv/bin/activate
Step 8: Model Training
In this step, we train theLSTM model with early stopping to prevent overfitting, and by using MLflow’s automated logging to track our model and experiments and save the trained model as lstm_model.keras.
#Install zenml pip install zenml #To Launch zenml server and dashboard locally pip install "zenml[server]" #To check the zenml Version: zenml version #To initiate a new repository zenml init #To run the dashboard locally: zenml login --local #To know the status of our zenml Pipelines zenml show #To shutdown the zenml server zenml clean
Step 9: Model Evaluation
As this is a regression problem, we are using evaluation metrics like Mean Squared Error (MSE), Root Mean Squared Error (MSE), Mean Absolute Error (MAE), and R-squared.
#Integrating mlflow with ZenML zenml integration install mlflow -y #Register the experiment tracker zenml experiment-tracker register mlflow_tracker --flavor=mlflow #Registering the model deployer zenml model-deployer register mlflow --flavor=mlflow #Registering the stack zenml stack register local-mlflow-stack-new -a default -o default -d mlflow -e mlflow_tracker --set #To view the stack list zenml stack --list
Now we shall organize all the above steps into a pipeline. Let’s create a new file training_pipeline.py.
bitcoin_price_prediction_mlops/ # Project directory ├── data/ │ └── management/ │ ├── api_to_mongodb.py # Code to fetch data and save it to MongoDB │ └── api.py # API-related utility functions │ ├── pipelines/ │ ├── deployment_pipeline.py # Deployment pipeline │ └── training_pipeline.py # Training pipeline │ ├── saved_models/ # Directory for storing trained models ├── saved_scalers/ # Directory for storing scalers used in data preprocessing │ ├── src/ # Source code │ ├── data_cleaning.py # Data cleaning and preprocessing │ ├── data_ingestion.py # Data ingestion │ ├── data_splitter.py # Data splitting │ ├── feature_engineering.py # Feature engineering │ ├── model_evaluation.py # Model evaluation │ └── model_training.py # Model training │ ├── steps/ # ZenML steps │ ├── clean_data.py # ZenML step for cleaning data │ ├── data_splitter.py # ZenML step for data splitting │ ├── dynamic_importer.py # ZenML step for importing dynamic data │ ├── feature_engineering.py # ZenML step for feature engineering │ ├── ingest_data.py # ZenML step for data ingestion │ ├── model_evaluation.py # ZenML step for model evaluation │ ├── model_training.py # ZenML step for training the model │ ├── prediction_service_loader.py # ZenML step for loading prediction services │ ├── predictor.py # ZenML step for prediction │ └── utils.py # Utility functions for steps │ ├── .env # Environment variables file ├── .gitignore # Git ignore file │ ├── app.py # Streamlit user interface app │ ├── README.md # Project documentation ├── requirements.txt # List of required packages ├── run_deployment.py # Code for running deployment and prediction pipeline ├── run_pipeline.py # Code for running training pipeline └── .zen/ # ZenML directory (created automatically after ZenML initialization)
Here,@pipelinedecorator is used to define the functionml_pipeline() as a pipeline in ZenML.
To view the dashboard for the training pipeline, simply run the run_pipeline.py script. Let’s create a run_pipeline.py file.
import os import logging from pymongo import MongoClient from dotenv import load_dotenv from zenml import step import pandas as pd # Load the .env file load_dotenv() # Get MongoDB URI from environment variables MONGO_URI = os.getenv("MONGO_URI") def fetch_data_from_mongodb(collection_name:str, database_name:str): """ Fetches data from MongoDB and converts it into a pandas DataFrame. collection_name: Name of the MongoDB collection to fetch data. database_name: Name of the MongoDB database. return: A pandas DataFrame containing the data """ # Connect to the MongoDB client client = MongoClient(MONGO_URI) db = client[database_name] # Select the database collection = db[collection_name] # Select the collection # Fetch all documents from the collection try: logging.info(f"Fetching data from MongoDB collection: {collection_name}...") data = list(collection.find()) # Convert cursor to a list of dictionaries if not data: logging.info("No data found in the MongoDB collection.") # Convert the list of dictionaries into a pandas DataFrame df = pd.DataFrame(data) # Drop the MongoDB ObjectId field if it exists (optional) if '_id' in df.columns: df = df.drop(columns=['_id']) logging.info("Data successfully fetched and converted to a DataFrame!") return df except Exception as e: logging.error(f"An error occurred while fetching data: {e}") raise e @step(enable_cache=False) def ingest_data(collection_name: str = "historical_data", database_name: str = "crypto_data") -> pd.DataFrame: logging.info("Started data ingestion process from MongoDB.") try: # Use the fetch_data_from_mongodb function to fetch data df = fetch_data_from_mongodb(collection_name=collection_name, database_name=database_name) if df.empty: logging.warning("No data was loaded. Check the collection name or the database content.") else: logging.info(f"Data ingestion completed. Number of records loaded: {len(df)}.") return df except Exception as e: logging.error(f"Error while reading data from {collection_name} in {database_name}: {e}") raise e
Now we have completed creating the pipeline. Run the command below to view the pipeline dashboard.
class DataPreprocessor: def __init__(self, data: pd.DataFrame): self.data = data logging.info("DataPreprocessor initialized with data of shape: %s", data.shape) def clean_data(self) -> pd.DataFrame: """ Performs data cleaning by removing unnecessary columns, dropping columns with missing values, and returning the cleaned DataFrame. Returns: pd.DataFrame: The cleaned DataFrame with unnecessary and missing-value columns removed. """ logging.info("Starting data cleaning process.") # Drop unnecessary columns, including '_id' if it exists columns_to_drop = [ 'UNIT', 'TYPE', 'MARKET', 'INSTRUMENT', 'FIRST_MESSAGE_TIMESTAMP', 'LAST_MESSAGE_TIMESTAMP', 'FIRST_MESSAGE_VALUE', 'HIGH_MESSAGE_VALUE', 'HIGH_MESSAGE_TIMESTAMP', 'LOW_MESSAGE_VALUE', 'LOW_MESSAGE_TIMESTAMP', 'LAST_MESSAGE_VALUE', 'TOTAL_INDEX_UPDATES', 'VOLUME_TOP_TIER', 'QUOTE_VOLUME_TOP_TIER', 'VOLUME_DIRECT', 'QUOTE_VOLUME_DIRECT', 'VOLUME_TOP_TIER_DIRECT', 'QUOTE_VOLUME_TOP_TIER_DIRECT', '_id' # Adding '_id' to the list ] logging.info("Dropping columns: %s") self.data = self.drop_columns(self.data, columns_to_drop) # Drop columns where the number of missing values is greater than 0 logging.info("Dropping columns with missing values.") self.data = self.drop_columns_with_missing_values(self.data) logging.info("Data cleaning completed. Data shape after cleaning: %s", self.data.shape) return self.data def drop_columns(self, data: pd.DataFrame, columns: list) -> pd.DataFrame: """ Drops specified columns from the DataFrame. Returns: pd.DataFrame: The DataFrame with the specified columns removed. """ logging.info("Dropping columns: %s", columns) return data.drop(columns=columns, errors='ignore') def drop_columns_with_missing_values(self, data: pd.DataFrame) -> pd.DataFrame: """ Drops columns with any missing values from the DataFrame. Parameters: data: pd.DataFrame The DataFrame from which columns with missing values will be removed. Returns: pd.DataFrame: The DataFrame with columns containing missing values removed. """ missing_columns = data.columns[data.isnull().sum() > 0] if not missing_columns.empty: logging.info("Columns with missing values: %s", missing_columns.tolist()) else: logging.info("No columns with missing values found.") return data.loc[:, data.isnull().sum() == 0]
After running the above command it will return the tracking dashboard URL, which looks like this.
The training pipeline looks like this in the dashboard, given below:
Step 10: Model Deployment
Till now we have built the model and the pipelines. Now let’s push the pipeline into production where users can make predictions.
Continuous Deployment Pipeline
import joblib import pandas as pd from abc import ABC, abstractmethod from sklearn.preprocessing import MinMaxScaler # Abstract class for Feature Engineering strategy class FeatureEngineeringStrategy(ABC): @abstractmethod def generate_features(self, df: pd.DataFrame) -> pd.DataFrame: pass # Concrete class for calculating SMA, EMA, RSI, and other features class TechnicalIndicators(FeatureEngineeringStrategy): def generate_features(self, df: pd.DataFrame) -> pd.DataFrame: # Calculate SMA, EMA, and RSI df['SMA_20'] = df['CLOSE'].rolling(window=20).mean() df['SMA_50'] = df['CLOSE'].rolling(window=50).mean() df['EMA_20'] = df['CLOSE'].ewm(span=20, adjust=False).mean() # Price difference features df['OPEN_CLOSE_diff'] = df['OPEN'] - df['CLOSE'] df['HIGH_LOW_diff'] = df['HIGH'] - df['LOW'] df['HIGH_OPEN_diff'] = df['HIGH'] - df['OPEN'] df['CLOSE_LOW_diff'] = df['CLOSE'] - df['LOW'] # Lagged features df['OPEN_lag1'] = df['OPEN'].shift(1) df['CLOSE_lag1'] = df['CLOSE'].shift(1) df['HIGH_lag1'] = df['HIGH'].shift(1) df['LOW_lag1'] = df['LOW'].shift(1) # Rolling statistics df['CLOSE_roll_mean_14'] = df['CLOSE'].rolling(window=14).mean() df['CLOSE_roll_std_14'] = df['CLOSE'].rolling(window=14).std() # Drop rows with missing values (due to rolling windows, shifts) df.dropna(inplace=True) return df # Abstract class for Scaling strategy class ScalingStrategy(ABC): @abstractmethod def scale(self, df: pd.DataFrame, features: list, target: str): pass # Concrete class for MinMax Scaling class MinMaxScaling(ScalingStrategy): def scale(self, df: pd.DataFrame, features: list, target: str): """ Scales the features and target using MinMaxScaler. Parameters: df: pd.DataFrame The DataFrame containing the features and target. features: list List of feature column names. target: str The target column name. Returns: pd.DataFrame, pd.DataFrame: Scaled features and target """ scaler_X = MinMaxScaler(feature_range=(0, 1)) scaler_y = MinMaxScaler(feature_range=(0, 1)) X_scaled = scaler_X.fit_transform(df[features].values) y_scaled = scaler_y.fit_transform(df[[target]].values) joblib.dump(scaler_X, 'saved_scalers/scaler_X.pkl') joblib.dump(scaler_y, 'saved_scalers/scaler_y.pkl') return X_scaled, y_scaled, scaler_y # FeatureEngineeringContext: This will use the Strategy Pattern class FeatureEngineering: def __init__(self, feature_strategy: FeatureEngineeringStrategy, scaling_strategy: ScalingStrategy): self.feature_strategy = feature_strategy self.scaling_strategy = scaling_strategy def process_features(self, df: pd.DataFrame, features: list, target: str): # Generate features using the provided strategy df_with_features = self.feature_strategy.generate_features(df) # Scale features and target using the provided strategy X_scaled, y_scaled, scaler_y = self.scaling_strategy.scale(df_with_features, features, target) return df_with_features, X_scaled, y_scaled, scaler_y
This pipeline is responsible for continuously deploying trained models. It first runs the ml_pipeline()from thetraining_pipeline.py file to train the model, then uses the Mlflow Model Deployerto deploy the trained model using the continuous_deployment_pipeline().
Inference Pipeline
We use an inference pipeline to make predictions on the new data, using the deployed model. Let’s take a look at how we implemented this pipeline in our project.
import requests import pandas as pd from dotenv import load_dotenv import os # Load the .env file load_dotenv() def fetch_crypto_data(api_uri): response = requests.get( api_uri, params={ "market": "cadli", "instrument": "BTC-USD", "limit": 5000, "aggregate": 1, "fill": "true", "apply_mapping": "true", "response_format": "JSON" }, headers={"Content-type": "application/json; charset=UTF-8"} ) if response.status_code == 200: print('API Connection Successful! \nFetching the data...') data = response.json() data_list = data.get('Data', []) df = pd.DataFrame(data_list) df['DATE'] = pd.to_datetime(df['TIMESTAMP'], unit='s') return df # Return the DataFrame else: raise Exception(f"API Error: {response.status_code} - {response.text}")
Let us see about each of the functions called in the inference pipeline below:
dynamic_importer()
This function loads the new data, performs data processing, and returns the data.
import os from pymongo import MongoClient from dotenv import load_dotenv from data.management.api import fetch_crypto_data # Import the API function import pandas as pd load_dotenv() MONGO_URI = os.getenv("MONGO_URI") API_URI = os.getenv("API_URI") client = MongoClient(MONGO_URI, ssl=True, ssl_certfile=None, ssl_ca_certs=None) db = client['crypto_data'] collection = db['historical_data'] try: latest_entry = collection.find_one(sort=[("DATE", -1)]) # Find the latest date if latest_entry: last_date = pd.to_datetime(latest_entry['DATE']).strftime('%Y-%m-%d') else: last_date = '2011-03-27' # Default start date if MongoDB is empty print(f"Fetching data starting from {last_date}...") new_data_df = fetch_crypto_data(API_URI) if latest_entry: new_data_df = new_data_df[new_data_df['DATE'] > last_date] if not new_data_df.empty: data_to_insert = new_data_df.to_dict(orient='records') result = collection.insert_many(data_to_insert) print(f"Inserted {len(result.inserted_ids)} new records into MongoDB.") else: print("No new data to insert.") except Exception as e: print(f"An error occurred: {e}")
prediction_service_loader()
This function is decorated with @step. We load the deployment service w.r.t the deployed model based on the pipeline_name, and step_name, where our deployed model is ready to process prediction queries for the new data.
The lineexisting_services=mlflow_model_deployer_component.find_model_server()searches for an available deployment service based on the given parameters like pipeline name and pipeline step name. If no services are available, it indicates that the deployment pipeline has either not been carried out or encountered a problem with the deployment pipeline, so it throws a RuntimeError.
#create a virtual environment python3 -m venv venv #Activate your virtual environmnent in your project folder source venv/bin/activate
predictor()
The function takes in the MLFlow-deployed model through the MLFlowDeploymentService and the new data. The data is processed further to match the expected format of the model to make real-time inferences.
#Install zenml pip install zenml #To Launch zenml server and dashboard locally pip install "zenml[server]" #To check the zenml Version: zenml version #To initiate a new repository zenml init #To run the dashboard locally: zenml login --local #To know the status of our zenml Pipelines zenml show #To shutdown the zenml server zenml clean
To visualize the continuous deployment and inference pipeline, we need to run the run_deployment.py script, where the deployment and prediction configurations will be defined. (Please check the run_deployment.py code in the GitHub given below).
#Integrating mlflow with ZenML zenml integration install mlflow -y #Register the experiment tracker zenml experiment-tracker register mlflow_tracker --flavor=mlflow #Registering the model deployer zenml model-deployer register mlflow --flavor=mlflow #Registering the stack zenml stack register local-mlflow-stack-new -a default -o default -d mlflow -e mlflow_tracker --set #To view the stack list zenml stack --list
Now let’s run the run_deployment.py file to see the dashboard of the continuous deployment pipeline and inference pipeline.
bitcoin_price_prediction_mlops/ # Project directory ├── data/ │ └── management/ │ ├── api_to_mongodb.py # Code to fetch data and save it to MongoDB │ └── api.py # API-related utility functions │ ├── pipelines/ │ ├── deployment_pipeline.py # Deployment pipeline │ └── training_pipeline.py # Training pipeline │ ├── saved_models/ # Directory for storing trained models ├── saved_scalers/ # Directory for storing scalers used in data preprocessing │ ├── src/ # Source code │ ├── data_cleaning.py # Data cleaning and preprocessing │ ├── data_ingestion.py # Data ingestion │ ├── data_splitter.py # Data splitting │ ├── feature_engineering.py # Feature engineering │ ├── model_evaluation.py # Model evaluation │ └── model_training.py # Model training │ ├── steps/ # ZenML steps │ ├── clean_data.py # ZenML step for cleaning data │ ├── data_splitter.py # ZenML step for data splitting │ ├── dynamic_importer.py # ZenML step for importing dynamic data │ ├── feature_engineering.py # ZenML step for feature engineering │ ├── ingest_data.py # ZenML step for data ingestion │ ├── model_evaluation.py # ZenML step for model evaluation │ ├── model_training.py # ZenML step for training the model │ ├── prediction_service_loader.py # ZenML step for loading prediction services │ ├── predictor.py # ZenML step for prediction │ └── utils.py # Utility functions for steps │ ├── .env # Environment variables file ├── .gitignore # Git ignore file │ ├── app.py # Streamlit user interface app │ ├── README.md # Project documentation ├── requirements.txt # List of required packages ├── run_deployment.py # Code for running deployment and prediction pipeline ├── run_pipeline.py # Code for running training pipeline └── .zen/ # ZenML directory (created automatically after ZenML initialization)
Continuous Deployment Pipeline – Output
Inference Pipeline – Output
After running the run_deployment.py file you can see the MLflow dashboard link which looks like this.
import os import logging from pymongo import MongoClient from dotenv import load_dotenv from zenml import step import pandas as pd # Load the .env file load_dotenv() # Get MongoDB URI from environment variables MONGO_URI = os.getenv("MONGO_URI") def fetch_data_from_mongodb(collection_name:str, database_name:str): """ Fetches data from MongoDB and converts it into a pandas DataFrame. collection_name: Name of the MongoDB collection to fetch data. database_name: Name of the MongoDB database. return: A pandas DataFrame containing the data """ # Connect to the MongoDB client client = MongoClient(MONGO_URI) db = client[database_name] # Select the database collection = db[collection_name] # Select the collection # Fetch all documents from the collection try: logging.info(f"Fetching data from MongoDB collection: {collection_name}...") data = list(collection.find()) # Convert cursor to a list of dictionaries if not data: logging.info("No data found in the MongoDB collection.") # Convert the list of dictionaries into a pandas DataFrame df = pd.DataFrame(data) # Drop the MongoDB ObjectId field if it exists (optional) if '_id' in df.columns: df = df.drop(columns=['_id']) logging.info("Data successfully fetched and converted to a DataFrame!") return df except Exception as e: logging.error(f"An error occurred while fetching data: {e}") raise e @step(enable_cache=False) def ingest_data(collection_name: str = "historical_data", database_name: str = "crypto_data") -> pd.DataFrame: logging.info("Started data ingestion process from MongoDB.") try: # Use the fetch_data_from_mongodb function to fetch data df = fetch_data_from_mongodb(collection_name=collection_name, database_name=database_name) if df.empty: logging.warning("No data was loaded. Check the collection name or the database content.") else: logging.info(f"Data ingestion completed. Number of records loaded: {len(df)}.") return df except Exception as e: logging.error(f"Error while reading data from {collection_name} in {database_name}: {e}") raise e
Now you need to copy and paste the above MLflow UI link in your command line and run it.
Here isthe MLflow dashboard, where you can see the evaluation metrics and model parameters:
Step 11: Building the Streamlit App
Streamlit is an amazing open-source, Python-based framework, used to create interactive UI’s, we can use Streamlit to build web apps quickly, without knowing backend or frontend development. First, we need to install Streamlit on our system.
class DataPreprocessor: def __init__(self, data: pd.DataFrame): self.data = data logging.info("DataPreprocessor initialized with data of shape: %s", data.shape) def clean_data(self) -> pd.DataFrame: """ Performs data cleaning by removing unnecessary columns, dropping columns with missing values, and returning the cleaned DataFrame. Returns: pd.DataFrame: The cleaned DataFrame with unnecessary and missing-value columns removed. """ logging.info("Starting data cleaning process.") # Drop unnecessary columns, including '_id' if it exists columns_to_drop = [ 'UNIT', 'TYPE', 'MARKET', 'INSTRUMENT', 'FIRST_MESSAGE_TIMESTAMP', 'LAST_MESSAGE_TIMESTAMP', 'FIRST_MESSAGE_VALUE', 'HIGH_MESSAGE_VALUE', 'HIGH_MESSAGE_TIMESTAMP', 'LOW_MESSAGE_VALUE', 'LOW_MESSAGE_TIMESTAMP', 'LAST_MESSAGE_VALUE', 'TOTAL_INDEX_UPDATES', 'VOLUME_TOP_TIER', 'QUOTE_VOLUME_TOP_TIER', 'VOLUME_DIRECT', 'QUOTE_VOLUME_DIRECT', 'VOLUME_TOP_TIER_DIRECT', 'QUOTE_VOLUME_TOP_TIER_DIRECT', '_id' # Adding '_id' to the list ] logging.info("Dropping columns: %s") self.data = self.drop_columns(self.data, columns_to_drop) # Drop columns where the number of missing values is greater than 0 logging.info("Dropping columns with missing values.") self.data = self.drop_columns_with_missing_values(self.data) logging.info("Data cleaning completed. Data shape after cleaning: %s", self.data.shape) return self.data def drop_columns(self, data: pd.DataFrame, columns: list) -> pd.DataFrame: """ Drops specified columns from the DataFrame. Returns: pd.DataFrame: The DataFrame with the specified columns removed. """ logging.info("Dropping columns: %s", columns) return data.drop(columns=columns, errors='ignore') def drop_columns_with_missing_values(self, data: pd.DataFrame) -> pd.DataFrame: """ Drops columns with any missing values from the DataFrame. Parameters: data: pd.DataFrame The DataFrame from which columns with missing values will be removed. Returns: pd.DataFrame: The DataFrame with columns containing missing values removed. """ missing_columns = data.columns[data.isnull().sum() > 0] if not missing_columns.empty: logging.info("Columns with missing values: %s", missing_columns.tolist()) else: logging.info("No columns with missing values found.") return data.loc[:, data.isnull().sum() == 0]
Again, you can find the code on GitHub for the Streamlit app.
Here’s the GitHub Code and Video Explanation of the Project for your better understanding.
Conclusion
In this article, we have successfully built an end-to-end, production-ready Bitcoin Price Prediction MLOps project. From acquiring data through an API and preprocessing it to model training, evaluation, and deployment, our project highlights the critical role of MLOps in connecting development with production. We’re one step closer to shaping the future of predicting Bitcoin prices in real time. APIs provide smooth access to external data, like Bitcoin price data from the CCData API, eliminating the need for a pre-existing dataset.
Key Takeaways
- APIs enable seamless access to external data, like Bitcoin price data from CCData API, eliminating the need for a pre-existing dataset.
- ZenML and MLflow are robust tools that facilitate the development, tracking, and deployment of machine learning models in real-world applications.
- We have followed best practices by properly performing data ingestion, cleaning, feature engineering, model training, and evaluation.
- Continuous deployment and inference pipelines are essential for ensuring that models remain efficient and available in production environments.
Frequently Asked Questions
Q1. Is ZenML free to use?A. Yes, ZenML is a fully open-source MLOps framework that makes the transition from local development to production pipelines as easy as 1 line of code.
Q2. What is MLflow used for?A. MLflow makes machine learning development easier by offering tools for tracking experiments, versioning models, and deploying them.
Q3. How to debug the server daemon is not running error?A. This is a common error you will face in the project. Just run `zenml logout –local` then `zenml clean`, and then `zenml login –local`, again run the pipeline. It will be resolved.
The above is the detailed content of Bitcoin Price Prediction Using MLops. For more information, please follow other related articles on the PHP Chinese website!

Hot AI Tools

Undresser.AI Undress
AI-powered app for creating realistic nude photos

AI Clothes Remover
Online AI tool for removing clothes from photos.

Undress AI Tool
Undress images for free

Clothoff.io
AI clothes remover

Video Face Swap
Swap faces in any video effortlessly with our completely free AI face swap tool!

Hot Article

Hot Tools

Notepad++7.3.1
Easy-to-use and free code editor

SublimeText3 Chinese version
Chinese version, very easy to use

Zend Studio 13.0.1
Powerful PHP integrated development environment

Dreamweaver CS6
Visual web development tools

SublimeText3 Mac version
God-level code editing software (SublimeText3)

Hot Topics
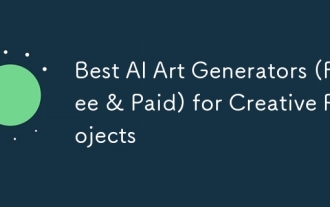
The article reviews top AI art generators, discussing their features, suitability for creative projects, and value. It highlights Midjourney as the best value for professionals and recommends DALL-E 2 for high-quality, customizable art.
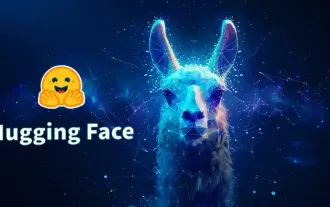
Meta's Llama 3.2: A Leap Forward in Multimodal and Mobile AI Meta recently unveiled Llama 3.2, a significant advancement in AI featuring powerful vision capabilities and lightweight text models optimized for mobile devices. Building on the success o
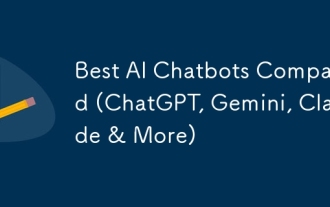
The article compares top AI chatbots like ChatGPT, Gemini, and Claude, focusing on their unique features, customization options, and performance in natural language processing and reliability.
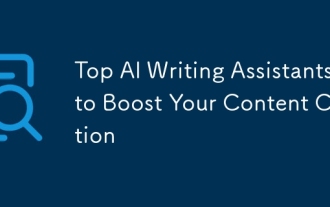
The article discusses top AI writing assistants like Grammarly, Jasper, Copy.ai, Writesonic, and Rytr, focusing on their unique features for content creation. It argues that Jasper excels in SEO optimization, while AI tools help maintain tone consist
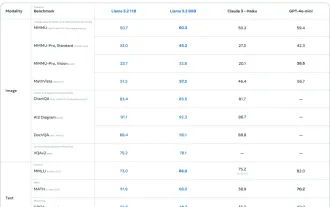
This week's AI landscape: A whirlwind of advancements, ethical considerations, and regulatory debates. Major players like OpenAI, Google, Meta, and Microsoft have unleashed a torrent of updates, from groundbreaking new models to crucial shifts in le
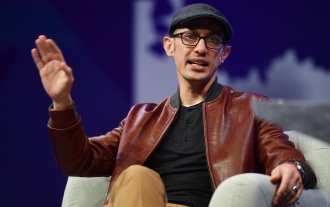
Shopify CEO Tobi Lütke's recent memo boldly declares AI proficiency a fundamental expectation for every employee, marking a significant cultural shift within the company. This isn't a fleeting trend; it's a new operational paradigm integrated into p
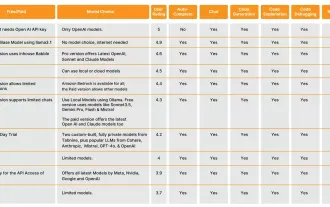
Hey there, Coding ninja! What coding-related tasks do you have planned for the day? Before you dive further into this blog, I want you to think about all your coding-related woes—better list those down. Done? – Let’
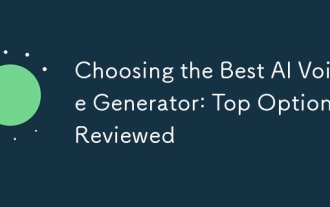
The article reviews top AI voice generators like Google Cloud, Amazon Polly, Microsoft Azure, IBM Watson, and Descript, focusing on their features, voice quality, and suitability for different needs.
