


Fine-Tuning LLaMA 2: A Step-by-Step Guide to Customizing the Large Language Model
Meta's LLaMA sparked a surge in Large Language Model (LLM) development, aiming to rival models like GPT-3.5. The open-source community rapidly produced increasingly powerful models, but these advancements weren't without challenges. Many open-source LLMs had restrictive licenses (research use only), required substantial budgets for fine-tuning, and were expensive to deploy.
LLaMA's new iteration addresses these issues with a commercial license and new methods enabling fine-tuning on consumer-grade GPUs with limited memory. This democratizes AI, allowing even smaller organizations to create tailored models.
This guide demonstrates fine-tuning Llama-2 on Google Colab, utilizing efficient techniques to overcome resource constraints. We'll explore methodologies that minimize memory usage and accelerate training.
Image generated by Author using DALL-E 3
Fine-Tuning Llama-2: A Step-by-Step Guide
This tutorial fine-tunes the 7-billion parameter Llama-2 model on a T4 GPU (available on Google Colab or Kaggle). The T4's 16GB VRAM necessitates parameter-efficient fine-tuning, specifically using QLoRA (4-bit precision). We'll utilize the Hugging Face ecosystem (transformers, accelerate, peft, trl, bitsandbytes).
1. Setup:
Install necessary libraries:
<code>%%capture %pip install accelerate peft bitsandbytes transformers trl</code>
Import modules:
<code>import os import torch from datasets import load_dataset from transformers import ( AutoModelForCausalLM, AutoTokenizer, BitsAndBytesConfig, TrainingArguments, pipeline, logging, ) from peft import LoraConfig from trl import SFTTrainer</code>
2. Model & Dataset Selection:
We'll use NousResearch/Llama-2-7b-chat-hf
(a readily accessible equivalent to the official Llama-2) as the base model and mlabonne/guanaco-llama2-1k
as our smaller training dataset.
<code>base_model = "NousResearch/Llama-2-7b-chat-hf" guanaco_dataset = "mlabonne/guanaco-llama2-1k" new_model = "llama-2-7b-chat-guanaco"</code>
Images illustrating the Hugging Face model and dataset are included here, same as original.
3. Loading Data & Model:
Load the dataset:
<code>dataset = load_dataset(guanaco_dataset, split="train")</code>
Configure 4-bit quantization using QLoRA:
<code>compute_dtype = getattr(torch, "float16") quant_config = BitsAndBytesConfig( load_in_4bit=True, bnb_4bit_quant_type="nf4", bnb_4bit_compute_dtype=compute_dtype, bnb_4bit_use_double_quant=False, )</code>
Load the Llama-2 model with 4-bit quantization:
<code>model = AutoModelForCausalLM.from_pretrained( base_model, quantization_config=quant_config, device_map={"": 0} ) model.config.use_cache = False model.config.pretraining_tp = 1</code>
Load the tokenizer:
<code>tokenizer = AutoTokenizer.from_pretrained(base_model, trust_remote_code=True) tokenizer.pad_token = tokenizer.eos_token tokenizer.padding_side = "right"</code>
Image illustrating QLoRA is included here, same as original.
4. PEFT Configuration:
Define PEFT parameters for efficient fine-tuning:
<code>peft_params = LoraConfig( lora_alpha=16, lora_dropout=0.1, r=64, bias="none", task_type="CAUSAL_LM", )</code>
5. Training Parameters:
Set training hyperparameters (output directory, epochs, batch sizes, learning rate, etc.). Details are the same as the original.
6. Fine-tuning with SFT:
Use the SFTTrainer
from the TRL library for supervised fine-tuning:
<code>trainer = SFTTrainer( model=model, train_dataset=dataset, peft_config=peft_params, dataset_text_field="text", max_seq_length=None, tokenizer=tokenizer, args=training_params, packing=False, ) trainer.train() trainer.model.save_pretrained(new_model) trainer.tokenizer.save_pretrained(new_model)</code>
Screenshots showing training progress and model saving are included here, same as original.
7. Evaluation:
Use the transformers
pipeline to test the fine-tuned model. Examples are provided, same as original.
8. Tensorboard Visualization:
Launch Tensorboard to monitor training metrics.
<code>%%capture %pip install accelerate peft bitsandbytes transformers trl</code>
Screenshot of Tensorboard is included here, same as original.
Conclusion:
This guide showcases efficient Llama-2 fine-tuning on limited hardware. The use of QLoRA and other techniques makes advanced LLMs accessible to a wider audience. Further resources and learning paths are mentioned at the end, similar to the original, but without the marketing calls to action.
The above is the detailed content of Fine-Tuning LLaMA 2: A Step-by-Step Guide to Customizing the Large Language Model. For more information, please follow other related articles on the PHP Chinese website!

Hot AI Tools

Undresser.AI Undress
AI-powered app for creating realistic nude photos

AI Clothes Remover
Online AI tool for removing clothes from photos.

Undress AI Tool
Undress images for free

Clothoff.io
AI clothes remover

Video Face Swap
Swap faces in any video effortlessly with our completely free AI face swap tool!

Hot Article

Hot Tools

Notepad++7.3.1
Easy-to-use and free code editor

SublimeText3 Chinese version
Chinese version, very easy to use

Zend Studio 13.0.1
Powerful PHP integrated development environment

Dreamweaver CS6
Visual web development tools

SublimeText3 Mac version
God-level code editing software (SublimeText3)

Hot Topics
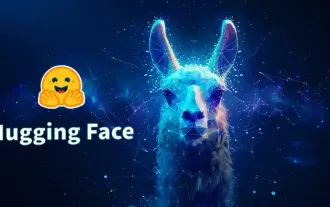
Meta's Llama 3.2: A Leap Forward in Multimodal and Mobile AI Meta recently unveiled Llama 3.2, a significant advancement in AI featuring powerful vision capabilities and lightweight text models optimized for mobile devices. Building on the success o
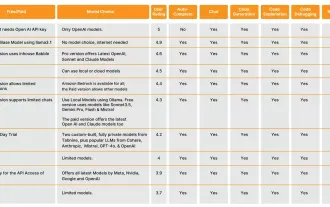
Hey there, Coding ninja! What coding-related tasks do you have planned for the day? Before you dive further into this blog, I want you to think about all your coding-related woes—better list those down. Done? – Let’
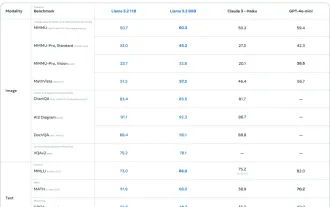
This week's AI landscape: A whirlwind of advancements, ethical considerations, and regulatory debates. Major players like OpenAI, Google, Meta, and Microsoft have unleashed a torrent of updates, from groundbreaking new models to crucial shifts in le
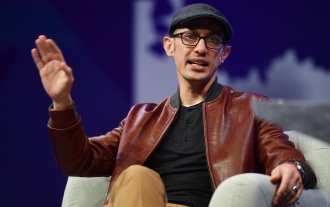
Shopify CEO Tobi Lütke's recent memo boldly declares AI proficiency a fundamental expectation for every employee, marking a significant cultural shift within the company. This isn't a fleeting trend; it's a new operational paradigm integrated into p
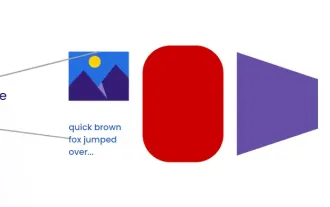
Introduction Imagine walking through an art gallery, surrounded by vivid paintings and sculptures. Now, what if you could ask each piece a question and get a meaningful answer? You might ask, “What story are you telling?
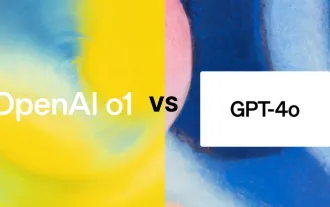
Introduction OpenAI has released its new model based on the much-anticipated “strawberry” architecture. This innovative model, known as o1, enhances reasoning capabilities, allowing it to think through problems mor
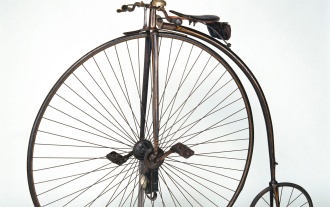
The 2025 Artificial Intelligence Index Report released by the Stanford University Institute for Human-Oriented Artificial Intelligence provides a good overview of the ongoing artificial intelligence revolution. Let’s interpret it in four simple concepts: cognition (understand what is happening), appreciation (seeing benefits), acceptance (face challenges), and responsibility (find our responsibilities). Cognition: Artificial intelligence is everywhere and is developing rapidly We need to be keenly aware of how quickly artificial intelligence is developing and spreading. Artificial intelligence systems are constantly improving, achieving excellent results in math and complex thinking tests, and just a year ago they failed miserably in these tests. Imagine AI solving complex coding problems or graduate-level scientific problems – since 2023
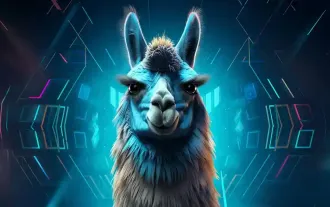
Meta's Llama 3.2: A Multimodal AI Powerhouse Meta's latest multimodal model, Llama 3.2, represents a significant advancement in AI, boasting enhanced language comprehension, improved accuracy, and superior text generation capabilities. Its ability t
