Using GPT-4 for Personal Styling
I've always loved fashion, collecting unique pieces and styling them in my own way. However, my closet was more of a disorganized mess than a curated collection. Adding new items risked toppling precariously balanced piles.
The Challenge: A cluttered closet is a common problem, especially for style-conscious individuals. This article details how I transformed my chaotic wardrobe into outfits I love, using a multi-step GPT setup. This showcases how a complex real-world task—managing hundreds of clothing items, accessories, and even makeup—can be handled effectively with AI, without system overload.
My initial idea: Could ChatGPT help manage my wardrobe? I experimented, creating a custom GPT-based fashion advisor, initially called "Glitter" (requires a paid OpenAI account). Through numerous iterations, I refined it into a smarter version, "Pico Glitter." Each step helped me conquer closet chaos and boost my confidence in daily outfit choices.
Here are some outfits Pico Glitter helped create:
(See Section B in Technical Notes for details on handling token limits and document truncation.)
- Early Experiments: I started with simple questions like, "What can I wear with a black leather jacket?" ChatGPT offered decent suggestions, but lacked knowledge of my personal style (e.g., "no black navy") and my specific wardrobe.
I then realized I could show ChatGPT my wardrobe—photographing items, providing brief descriptions, and letting it recommend outfits. The first iteration (Glitter) struggled with memory, but proved the concept was viable.
GPT-4's leather jacket styling advice
Pico Glitter's improved advice for the same jacket.
(See Section A.1 in Technical Notes for multi-model pipeline details.)
- Building a Smarter Stylist: I photographed and summarized each garment concisely (e.g., "Black V-neck T-shirt, short sleeves"). I organized items by category (shoes, tops, etc.) for easier GPT referencing. (I used o1 to organize this initially chaotic data.)
The improvement was dramatic. Pico Glitter referenced items accurately and suggested outfits I'd actually wear.
Sample inventory category (Belts).
(See Section A.2 for details on summarization vs. chunking.)
- Addressing Memory Limitations: Like all LLMs, ChatGPT has memory limitations. Pico Glitter sometimes forgot items or invented outfits. To solve this, I periodically reminded it to re-check the full wardrobe list, often restarting the session.
A hallucinated outfit: turquoise cargo pants with lavender clogs?!
4. Evolving GPT Personalities: I experimented with different GPT personalities:
- Mini-Glitter: Very rule-oriented, but lacked creativity.
- Micro-Glitter: Too creative, suggesting outlandish combinations.
- Nano-Glitter: Overly complex and repetitive due to a self-modification feedback loop.
Pico Glitter found the optimal balance—respecting my style while offering inspiration. I refined prompts and showed examples of outfits I liked (or disliked).
Pico Glitter's self-portrait.
5. Transforming My Wardrobe: Pico Glitter's suggestions revealed which clothes I wore frequently and which I neglected. I donated over 50 bags of unworn items, even discovering duplicates!
Before Glitter, getting dressed was a 30-60 minute process. Now, using saved "recipes" (outfit descriptions), it takes 3-4 minutes. Creating new looks rarely exceeds 15-20 minutes. Pico Glitter eliminates guesswork.
Outfit "Recipes"
I ask Pico Glitter to create ensembles, iterating with image uploads and feedback. I save "recipes" (name and item list) in my Notes app with tags (#casual, #business, etc.) and a photo.
### High-Low Combinations
I love mixing high-end and budget-friendly pieces—Pico Glitter seamlessly pairs them based on color, silhouette, and overall style.
- Key Takeaways:
- Start small: Test with a few items.
- Stay organized: Use concise summaries.
- Regularly refresh: Remind the GPT to re-check the wardrobe list.
- Learn from suggestions: Identify frequently suggested items and those rarely used.
- Experiment: Unexpected pairings can lead to great looks.
7. Conclusion: My closet is still evolving, but Pico Glitter transformed it from chaos to wearable style. The collaboration between me and the GPT is key—I provide style rules and items; it provides combinations, and together, we refine until we achieve outfits that feel authentically me.
Call to action:
- Get my config: A starter config for your own GPT-based stylist.
- Share your results: Tag @GlitterGPT (Instagram, TikTok, X).
*(See Technical Notes for technical details.)*
Technical Notes: (Detailed technical explanation of multi-model pipelines, handling truncation, context windows, prompt engineering, and lessons learned.) (This section remains largely unchanged from the original, as it provides valuable technical insight.)
The above is the detailed content of Using GPT-4 for Personal Styling. For more information, please follow other related articles on the PHP Chinese website!

Hot AI Tools

Undresser.AI Undress
AI-powered app for creating realistic nude photos

AI Clothes Remover
Online AI tool for removing clothes from photos.

Undress AI Tool
Undress images for free

Clothoff.io
AI clothes remover

Video Face Swap
Swap faces in any video effortlessly with our completely free AI face swap tool!

Hot Article

Hot Tools

Notepad++7.3.1
Easy-to-use and free code editor

SublimeText3 Chinese version
Chinese version, very easy to use

Zend Studio 13.0.1
Powerful PHP integrated development environment

Dreamweaver CS6
Visual web development tools

SublimeText3 Mac version
God-level code editing software (SublimeText3)

Hot Topics










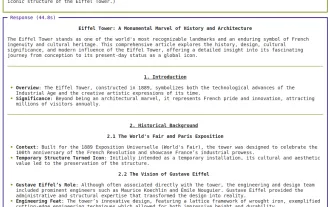
While working on Agentic AI, developers often find themselves navigating the trade-offs between speed, flexibility, and resource efficiency. I have been exploring the Agentic AI framework and came across Agno (earlier it was Phi-
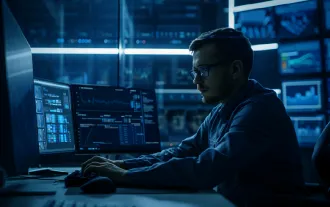
SQL's ALTER TABLE Statement: Dynamically Adding Columns to Your Database In data management, SQL's adaptability is crucial. Need to adjust your database structure on the fly? The ALTER TABLE statement is your solution. This guide details adding colu
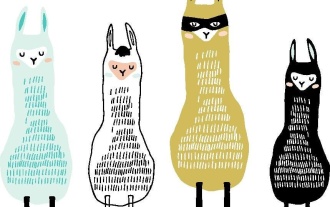
Troubled Benchmarks: A Llama Case Study In early April 2025, Meta unveiled its Llama 4 suite of models, boasting impressive performance metrics that positioned them favorably against competitors like GPT-4o and Claude 3.5 Sonnet. Central to the launc
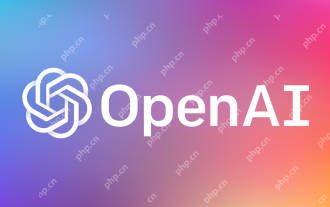
The release includes three distinct models, GPT-4.1, GPT-4.1 mini and GPT-4.1 nano, signaling a move toward task-specific optimizations within the large language model landscape. These models are not immediately replacing user-facing interfaces like
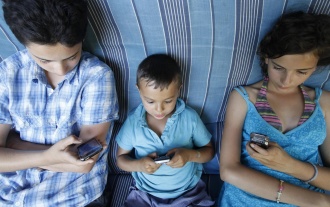
Can a video game ease anxiety, build focus, or support a child with ADHD? As healthcare challenges surge globally — especially among youth — innovators are turning to an unlikely tool: video games. Now one of the world’s largest entertainment indus
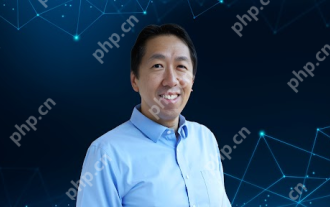
Unlock the Power of Embedding Models: A Deep Dive into Andrew Ng's New Course Imagine a future where machines understand and respond to your questions with perfect accuracy. This isn't science fiction; thanks to advancements in AI, it's becoming a r
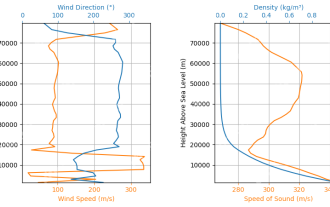
Simulate Rocket Launches with RocketPy: A Comprehensive Guide This article guides you through simulating high-power rocket launches using RocketPy, a powerful Python library. We'll cover everything from defining rocket components to analyzing simula
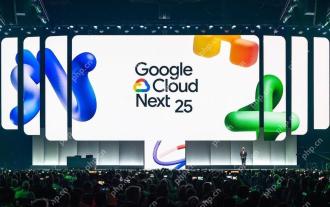
Gemini as the Foundation of Google’s AI Strategy Gemini is the cornerstone of Google’s AI agent strategy, leveraging its advanced multimodal capabilities to process and generate responses across text, images, audio, video and code. Developed by DeepM
