MiniRAG: RAG That Works on the Edge
MiniRAG: A Lightweight RAG Framework for Resource-Constrained Environments
The increasing demand for efficient and compact Retrieval-Augmented Generation (RAG) systems, especially in resource-limited settings, presents significant hurdles. Existing RAG frameworks heavily rely on Large Language Models (LLMs), leading to substantial computational costs and scalability limitations on edge devices. Researchers from the University of Hong Kong address this challenge with MiniRAG, a novel framework prioritizing simplicity and efficiency.
Key Learning Points:
- Understanding the limitations of traditional LLM-based RAG systems and the need for lightweight alternatives like MiniRAG.
- Exploring MiniRAG's integration of Small Language Models (SLMs) with graph-based indexing for optimized retrieval and generation.
- Examining MiniRAG's core components: Heterogeneous Graph Indexing and Topology-Enhanced Retrieval.
- Appreciating MiniRAG's advantages in resource-constrained environments, such as edge devices.
- Grasping the implementation and setup of MiniRAG for on-device AI applications.
This article is part of the Data Science Blogathon.
Table of Contents:
- Challenges of Current RAG Systems
- The MiniRAG Framework
- MiniRAG Workflow
- MiniRAG's Significance
- Hands-on with MiniRAG
- Future Implications
- Conclusion
Challenges of Current RAG Systems:
While LLM-centric RAG systems excel in tasks demanding semantic understanding and reasoning, their resource intensity makes them unsuitable for edge devices or privacy-focused applications. Replacing LLMs with SLMs often fails due to:
- Diminished semantic comprehension.
- Difficulties handling large, noisy datasets.
- Inefficiency in multi-step reasoning.
The MiniRAG Framework:
MiniRAG significantly differs from traditional RAG systems by offering a lightweight, efficient architecture designed for SLMs. This is achieved through two key components: Heterogeneous Graph Indexing and Lightweight Graph-Based Knowledge Retrieval.
Heterogeneous Graph Indexing:
MiniRAG's core innovation is its Heterogeneous Graph Indexing, simplifying knowledge representation while mitigating SLMs' semantic understanding limitations.
-
Key Features:
- Dual-Node Design: Text chunk nodes (preserving context) and entity nodes (key semantic elements).
- Edge Connections: Entity-entity edges (capturing relationships) and entity-chunk edges (maintaining contextual relevance).
-
Functionality: Extracts entities and chunks, constructs a graph linking them, and semantically enriches edges.
-
Benefits: Reduces dependence on semantic understanding and offers efficient knowledge representation.
Lightweight Graph-Based Knowledge Retrieval:
MiniRAG's retrieval mechanism uses the graph structure for precise and efficient query resolution, maximizing SLMs' strengths in localized reasoning and pattern matching.
-
Key Features:
- Query Semantic Mapping: SLMs extract entities and predict answer types, aligning the query with graph nodes using lightweight sentence embeddings.
- Reasoning Path Discovery: Identifies relevant entities and connections by analyzing graph topology and semantic relevance, scoring paths based on query importance.
- Topology-Enhanced Retrieval: Combines semantic relevance with structural coherence to find meaningful reasoning paths, reducing noise.
-
Functionality: Processes queries, explores graph paths, retrieves relevant text chunks, and generates responses.
-
Benefits: Offers precision and efficiency, and adaptability across various datasets.
MiniRAG Workflow:
The workflow integrates the components into a streamlined pipeline: input query processing, graph interaction, knowledge retrieval, and output generation.
MiniRAG's Significance:
MiniRAG's design ensures scalability, robustness, and privacy, setting a new standard for RAG systems in low-resource environments.
Hands-on with MiniRAG:
MiniRAG is a lightweight framework for RAG designed for efficient use with SLMs. The provided installation and usage instructions are simplified for clarity. Refer to the original documentation for complete details.
Future Implications:
MiniRAG's lightweight design enables edge device deployment of RAG systems, balancing efficiency, privacy, and accuracy. Its contributions include a novel indexing and retrieval approach and a benchmark dataset for evaluating on-device RAG capabilities.
Conclusion:
MiniRAG bridges the gap between computational efficiency and semantic understanding, enabling scalable and robust RAG systems for resource-constrained environments. Its simplicity and graph-based structure offer a transformative solution for on-device AI applications.
Key Takeaways:
- MiniRAG optimizes SLMs for efficient RAG.
- It combines Heterogeneous Graph Indexing and Topology-Enhanced Retrieval for enhanced performance without large models.
- MiniRAG significantly reduces computational costs and storage compared to traditional RAG systems.
- It provides a scalable, robust solution for resource-constrained environments, prioritizing privacy.
- It simplifies retrieval and leverages graph structures to address the challenges of using SLMs for semantic understanding and reasoning.
Q&A: (Simplified answers provided for brevity)
Q1: What is MiniRAG? A1: A lightweight RAG framework using SLMs and graph-based indexing for resource-constrained environments.
Q2: Key features of MiniRAG? A2: Heterogeneous Graph Indexing and Topology-Enhanced Retrieval.
Q3: How does MiniRAG differ from other RAG systems? A3: It uses SLMs and graph structures instead of computationally expensive LLMs.
Q4: What models does MiniRAG support? A4: Several SLMs (specific models listed in the original text).
(Note: Image URLs remain unchanged.)
The above is the detailed content of MiniRAG: RAG That Works on the Edge. For more information, please follow other related articles on the PHP Chinese website!

Hot AI Tools

Undresser.AI Undress
AI-powered app for creating realistic nude photos

AI Clothes Remover
Online AI tool for removing clothes from photos.

Undress AI Tool
Undress images for free

Clothoff.io
AI clothes remover

Video Face Swap
Swap faces in any video effortlessly with our completely free AI face swap tool!

Hot Article

Hot Tools

Notepad++7.3.1
Easy-to-use and free code editor

SublimeText3 Chinese version
Chinese version, very easy to use

Zend Studio 13.0.1
Powerful PHP integrated development environment

Dreamweaver CS6
Visual web development tools

SublimeText3 Mac version
God-level code editing software (SublimeText3)

Hot Topics










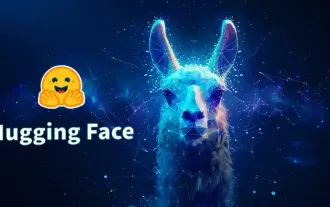
Meta's Llama 3.2: A Leap Forward in Multimodal and Mobile AI Meta recently unveiled Llama 3.2, a significant advancement in AI featuring powerful vision capabilities and lightweight text models optimized for mobile devices. Building on the success o
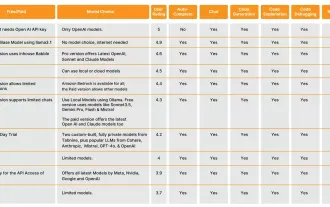
Hey there, Coding ninja! What coding-related tasks do you have planned for the day? Before you dive further into this blog, I want you to think about all your coding-related woes—better list those down. Done? – Let’
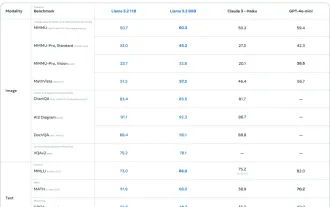
This week's AI landscape: A whirlwind of advancements, ethical considerations, and regulatory debates. Major players like OpenAI, Google, Meta, and Microsoft have unleashed a torrent of updates, from groundbreaking new models to crucial shifts in le
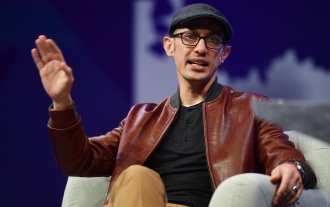
Shopify CEO Tobi Lütke's recent memo boldly declares AI proficiency a fundamental expectation for every employee, marking a significant cultural shift within the company. This isn't a fleeting trend; it's a new operational paradigm integrated into p
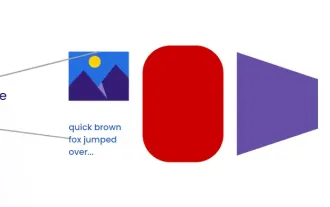
Introduction Imagine walking through an art gallery, surrounded by vivid paintings and sculptures. Now, what if you could ask each piece a question and get a meaningful answer? You might ask, “What story are you telling?
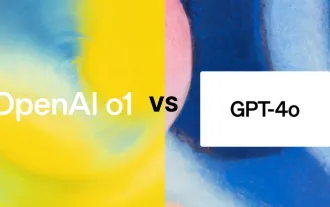
Introduction OpenAI has released its new model based on the much-anticipated “strawberry” architecture. This innovative model, known as o1, enhances reasoning capabilities, allowing it to think through problems mor
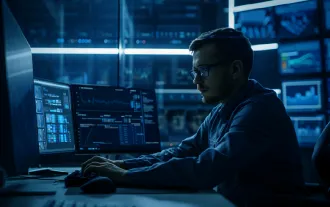
SQL's ALTER TABLE Statement: Dynamically Adding Columns to Your Database In data management, SQL's adaptability is crucial. Need to adjust your database structure on the fly? The ALTER TABLE statement is your solution. This guide details adding colu
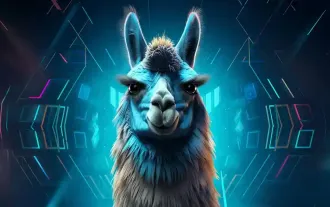
Meta's Llama 3.2: A Multimodal AI Powerhouse Meta's latest multimodal model, Llama 3.2, represents a significant advancement in AI, boasting enhanced language comprehension, improved accuracy, and superior text generation capabilities. Its ability t
