After DeepSeek, Kimi k1.5 Outshines OpenAI o1
Kimi k1.5: A Generative AI Reasoning Model Reshaping the Landscape
Recent breakthroughs in reinforcement learning (RL) and large language models (LLMs) have culminated in the creation of Kimi k1.5, a model poised to revolutionize generative AI reasoning. This article delves into Kimi k1.5's key features, innovations, and potential impact, drawing insights from the accompanying research.
Table of Contents:
- What is Kimi k1.5?
- Kimi k1.5 Training
- Kimi k1.5 Benchmarks
- Kimi k1.5's Key Innovations
- Kimi k1.5 vs. DeepSeek R1
- Accessing Kimi k1.5 via API
- Conclusion
What is Kimi k1.5?
Kimi k1.5 represents a substantial leap forward in scaling RL with LLMs. Unlike conventional models relying on intricate methods like Monte Carlo tree search, it employs a streamlined approach centered on autoregressive prediction and RL techniques. Its design enables it to handle multimodal tasks, showcasing exceptional performance in benchmarks like Math Vista and Live Code Bench.
Kimi k1.5 Training
Kimi k1.5's training is a multi-stage process designed to enhance reasoning through RL and multimodal integration:
-
Pretraining: The model is pretrained on a vast, high-quality multimodal dataset encompassing text (English, Chinese, code, math, general knowledge) and visual data, rigorously filtered for relevance and diversity.
-
Supervised Fine-Tuning (SFT): This involves two phases: vanilla SFT using ~1 million examples across various tasks, and Long-Chain-of-Thought (CoT) SFT for training complex reasoning pathways.
-
Reinforcement Learning (RL): A carefully curated prompt set drives the RL training. The model learns to generate solutions through a sequence of reasoning steps, guided by a reward model evaluating response accuracy. Online mirror descent optimizes the policy.
-
Partial Rollouts: To efficiently handle long contexts, Kimi k1.5 uses partial rollouts, saving unfinished portions for later continuation.
-
Length Penalty & Sampling: A length penalty encourages concise answers, while curriculum and prioritized sampling strategies focus training on easier tasks first.
-
Evaluation & Iteration: Continuous evaluation against benchmarks guides iterative model updates.
Kimi k1.5 System Overview & Partial Rollout Diagrams:
Kimi k1.5 Benchmarks
Kimi k1.5 demonstrates state-of-the-art performance across diverse tasks:
- Mathematics: Achieved a perfect score of 77.5 on AIME 2024, surpassing OpenAI o1 (74.4) and OpenAI o1 mini (63.6). Scored 96.2 on MATH-500.
- Coding: Achieved a score of 94 on CodeForces, matching OpenAI o1 and exceeding o1-mini and QwQ 72B preview.
- Visual Reasoning: Scored 74.9 on MathVista_test, surpassing QvQ 72B (71.4) and OpenAI o1-mini (71).
- General Knowledge: Scored 87.4 on MMLU (EM), outperforming OpenAI 4o (87.2).
Reasoning Strategies Diagram:
Kimi k1.5 Key Innovations
- Long Context Scaling: Processes up to 128,000 tokens, enhancing efficiency through partial rollouts.
- Chain of Thought Reasoning: Combines long and short CoT strategies for adaptability.
- Reinforcement Learning Pipeline: A refined RL pipeline with curated prompts, supervised fine-tuning, and policy optimization.
- Multimodal Data Handling: Effectively processes text and visual data.
Kimi k1.5 vs. DeepSeek R1
Kimi k1.5 and DeepSeek R1 represent different approaches to LLM development. Kimi k1.5's streamlined architecture, integrated RL, and long context handling distinguish it from DeepSeek R1's more traditional methods. The differences impact their performance on complex, context-heavy tasks.
Accessing Kimi k1.5 via API
API access requires registration on KIMI's management console. An example Python code snippet demonstrates API interaction:
# ... (API key setup and message preparation) ... stream = client.chat.completions.create( model="kimi-k1.5-preview", messages=messages, temperature=0.3, stream=True, max_tokens=8192, ) # ... (streaming response handling) ...
Conclusion
Kimi k1.5 represents a significant advancement in generative AI reasoning, simplifying RL design while achieving state-of-the-art results. Its innovations in context scaling and multimodal data handling position it as a leading model with broad implications across various industries.
The above is the detailed content of After DeepSeek, Kimi k1.5 Outshines OpenAI o1. For more information, please follow other related articles on the PHP Chinese website!

Hot AI Tools

Undresser.AI Undress
AI-powered app for creating realistic nude photos

AI Clothes Remover
Online AI tool for removing clothes from photos.

Undress AI Tool
Undress images for free

Clothoff.io
AI clothes remover

Video Face Swap
Swap faces in any video effortlessly with our completely free AI face swap tool!

Hot Article

Hot Tools

Notepad++7.3.1
Easy-to-use and free code editor

SublimeText3 Chinese version
Chinese version, very easy to use

Zend Studio 13.0.1
Powerful PHP integrated development environment

Dreamweaver CS6
Visual web development tools

SublimeText3 Mac version
God-level code editing software (SublimeText3)

Hot Topics










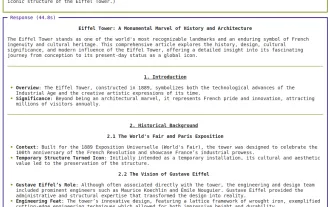
While working on Agentic AI, developers often find themselves navigating the trade-offs between speed, flexibility, and resource efficiency. I have been exploring the Agentic AI framework and came across Agno (earlier it was Phi-
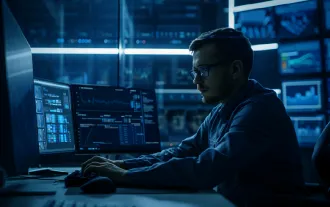
SQL's ALTER TABLE Statement: Dynamically Adding Columns to Your Database In data management, SQL's adaptability is crucial. Need to adjust your database structure on the fly? The ALTER TABLE statement is your solution. This guide details adding colu
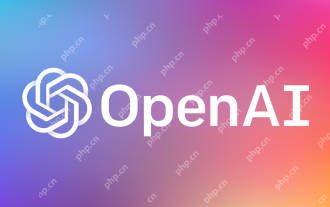
The release includes three distinct models, GPT-4.1, GPT-4.1 mini and GPT-4.1 nano, signaling a move toward task-specific optimizations within the large language model landscape. These models are not immediately replacing user-facing interfaces like
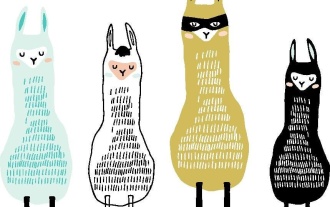
Troubled Benchmarks: A Llama Case Study In early April 2025, Meta unveiled its Llama 4 suite of models, boasting impressive performance metrics that positioned them favorably against competitors like GPT-4o and Claude 3.5 Sonnet. Central to the launc
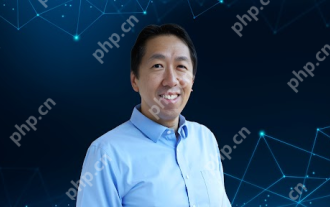
Unlock the Power of Embedding Models: A Deep Dive into Andrew Ng's New Course Imagine a future where machines understand and respond to your questions with perfect accuracy. This isn't science fiction; thanks to advancements in AI, it's becoming a r
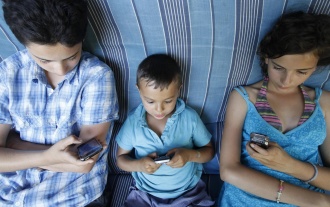
Can a video game ease anxiety, build focus, or support a child with ADHD? As healthcare challenges surge globally — especially among youth — innovators are turning to an unlikely tool: video games. Now one of the world’s largest entertainment indus
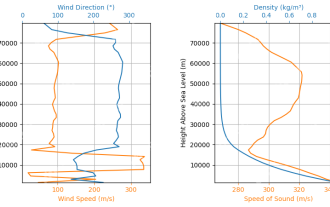
Simulate Rocket Launches with RocketPy: A Comprehensive Guide This article guides you through simulating high-power rocket launches using RocketPy, a powerful Python library. We'll cover everything from defining rocket components to analyzing simula
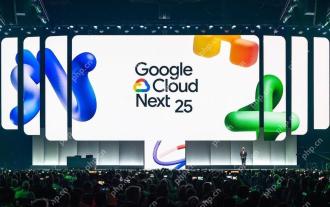
Gemini as the Foundation of Google’s AI Strategy Gemini is the cornerstone of Google’s AI agent strategy, leveraging its advanced multimodal capabilities to process and generate responses across text, images, audio, video and code. Developed by DeepM
