How to Run Qwen2.5 Models Locally in 3 Minutes?
Qwen2.5-Max: A Cost-Effective, Human-Like Reasoning Large Language Model
The AI landscape is buzzing with powerful, cost-effective models like DeepSeek, Mistral Small 3, and Qwen2.5 Max. Qwen2.5-Max, in particular, is making waves as a potent Mixture-of-Experts (MoE) model, even outperforming DeepSeek V3 in some benchmarks. Its advanced architecture and massive training dataset (up to 18 trillion tokens) are setting new standards for performance. This article explores Qwen2.5-Max's architecture, its competitive advantages, and its potential to rival DeepSeek V3. We'll also guide you through running Qwen2.5 models locally.
Key Qwen2.5 Model Features:
- Multilingual Support: Supports over 29 languages.
- Extended Context: Handles long contexts up to 128K tokens.
- Enhanced Capabilities: Significant improvements in coding, math, instruction following, and structured data understanding.
Table of Contents:
- Key Qwen2.5 Model Features
- Using Qwen2.5 with Ollama
- Qwen2.5:7b Inference
- Qwen2.5-coder:3b Inference
- Conclusion
Running Qwen2.5 Locally with Ollama:
First, install Ollama: Ollama Download Link
Linux/Ubuntu users: curl -fsSL https://ollama.com/install.sh | sh
Available Qwen2.5 Ollama Models:
We'll use the 7B parameter model (approx. 4.7 GB). Smaller models are available for users with limited resources.
Qwen2.5:7b Inference:
ollama pull qwen2.5:7b
The pull
command will download the model. You'll see output similar to this:
<code>pulling manifest pulling 2bada8a74506... 100% ▕████████████████▏ 4.7 GB ... (rest of the output) ... success</code>
Then run the model:
ollama run qwen2.5:7b
Example Queries:
Prompt: Define vector databases in 30 words.
<code>Vector databases efficiently store and query numerical arrays (vectors), often using approximations for fast similarity searches in large datasets.</code>
Prompt: List some examples.
<code>Popular vector databases include Pinecone, Weaviate, Milvus, ChromaDB, and Amazon Aurora Vectorstore.</code>
(Press Ctrl D to exit)
Note: Locally-run models lack real-time access and web search capabilities. For example:
Prompt: What's today's date?
<code>Today's date is unavailable. My knowledge is not updated in real-time.</code>
Qwen2.5-coder:3b Inference:
Follow the same process, substituting qwen2.5-coder:3b
for qwen2.5:7b
in the pull
and run
commands.
Example Coding Prompts:
Prompt: Provide Python code for the Fibonacci sequence.
(Output: Python code for Fibonacci sequence will be displayed here)
Prompt: Create a simple calculator using Python functions.
(Output: Python code for a simple calculator will be displayed here)
Conclusion:
This guide demonstrates how to run Qwen2.5 models locally using Ollama, highlighting Qwen2.5-Max's strengths: 128K context length, multilingual support, and enhanced capabilities. While local execution improves security, it sacrifices real-time information access. Qwen2.5 offers a compelling balance between efficiency, security, and performance, making it a strong alternative to DeepSeek V3 for various AI applications. Further information on accessing Qwen2.5-Max via Google Colab is available in a separate resource.
The above is the detailed content of How to Run Qwen2.5 Models Locally in 3 Minutes?. For more information, please follow other related articles on the PHP Chinese website!

Hot AI Tools

Undresser.AI Undress
AI-powered app for creating realistic nude photos

AI Clothes Remover
Online AI tool for removing clothes from photos.

Undress AI Tool
Undress images for free

Clothoff.io
AI clothes remover

Video Face Swap
Swap faces in any video effortlessly with our completely free AI face swap tool!

Hot Article

Hot Tools

Notepad++7.3.1
Easy-to-use and free code editor

SublimeText3 Chinese version
Chinese version, very easy to use

Zend Studio 13.0.1
Powerful PHP integrated development environment

Dreamweaver CS6
Visual web development tools

SublimeText3 Mac version
God-level code editing software (SublimeText3)

Hot Topics










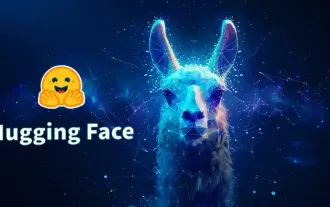
Meta's Llama 3.2: A Leap Forward in Multimodal and Mobile AI Meta recently unveiled Llama 3.2, a significant advancement in AI featuring powerful vision capabilities and lightweight text models optimized for mobile devices. Building on the success o
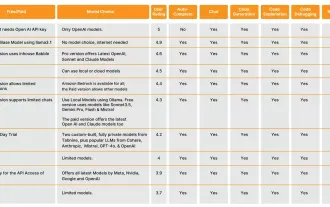
Hey there, Coding ninja! What coding-related tasks do you have planned for the day? Before you dive further into this blog, I want you to think about all your coding-related woes—better list those down. Done? – Let’
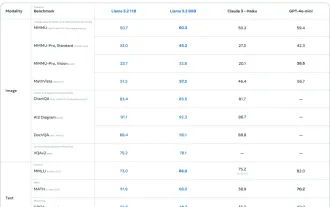
This week's AI landscape: A whirlwind of advancements, ethical considerations, and regulatory debates. Major players like OpenAI, Google, Meta, and Microsoft have unleashed a torrent of updates, from groundbreaking new models to crucial shifts in le
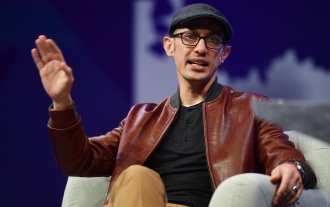
Shopify CEO Tobi Lütke's recent memo boldly declares AI proficiency a fundamental expectation for every employee, marking a significant cultural shift within the company. This isn't a fleeting trend; it's a new operational paradigm integrated into p
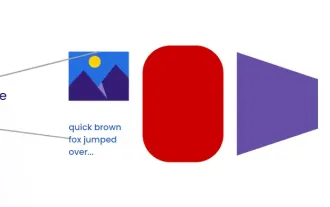
Introduction Imagine walking through an art gallery, surrounded by vivid paintings and sculptures. Now, what if you could ask each piece a question and get a meaningful answer? You might ask, “What story are you telling?
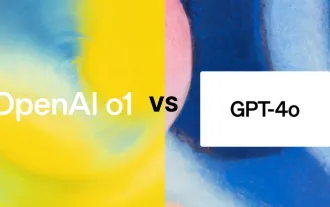
Introduction OpenAI has released its new model based on the much-anticipated “strawberry” architecture. This innovative model, known as o1, enhances reasoning capabilities, allowing it to think through problems mor
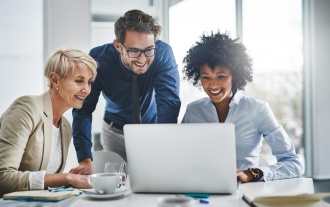
For those of you who might be new to my column, I broadly explore the latest advances in AI across the board, including topics such as embodied AI, AI reasoning, high-tech breakthroughs in AI, prompt engineering, training of AI, fielding of AI, AI re
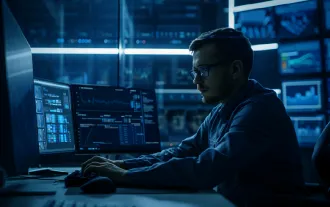
SQL's ALTER TABLE Statement: Dynamically Adding Columns to Your Database In data management, SQL's adaptability is crucial. Need to adjust your database structure on the fly? The ALTER TABLE statement is your solution. This guide details adding colu
