DeepGEMM Released on Day 3 of DeepSeek Open Source Week
DeepSeek Releases DeepGEMM: A High-Performance FP8 GEMM Library for AI
As part of #OpenSourceWeek, DeepSeek unveiled DeepGEMM, a cutting-edge library optimized for efficient FP8 General Matrix Multiplications (GEMMs). This library supports both dense and Mixture-of-Experts (MoE) GEMMs, proving invaluable for V3/R1 model training and inference. DeepGEMM aims to significantly boost performance and efficiency in AI workloads, reinforcing DeepSeek's commitment to open-source innovation.
? Day 3 of #OpenSourceWeek: DeepGEMM
Introducing DeepGEMM – an FP8 GEMM library supporting dense and MoE GEMMs, powering V3/R1 training and inference.
⚡ Up to 1350 FP8 TFLOPS on Hopper GPUs
✅ Minimal dependencies, designed for ease of use
✅ Fully Just-In-Time compiled…— DeepSeek (@deepseek_ai) February 26, 2025
This release follows the successful launches of DeepSeek FlashML (Day 1) and DeepSeek DeepEP (Day 2).
Table of Contents
- What is GEMM?
- What is FP8?
- The Need for DeepGEMM
- Key Features of DeepGEMM
- Performance Benchmarks
- Installation Instructions
- Conclusion
What is GEMM?
General Matrix Multiplication (GEMM) is a fundamental linear algebra operation multiplying two matrices to produce a third. Widely used across numerous applications, its formula is:
GEMM is crucial for model performance optimization, particularly in deep learning for neural network training and inference.
This illustration shows GEMM, highlighting tiling (dividing matrices into smaller blocks – Mtile, Ntile, Ktile) for optimized cache utilization. This improves performance through enhanced data locality and parallelism.
What is FP8?
FP8 (8-bit floating-point) is a high-performance computing format offering reduced precision and efficient numerical data representation. It's particularly beneficial for handling the computational demands of large datasets in machine learning.
The typical FP8 format includes:
- 1 sign bit
- 5 exponent bits
- 2 fraction bits
This compact structure enables faster computations and reduced memory usage, ideal for training large models. While precision might be slightly compromised, this is often acceptable, even leading to performance gains due to reduced computational overhead.
This image compares FP8 (E4M3 and E5M2 formats) with FP16 and BF16, illustrating the trade-offs between precision and range for different floating-point formats.
The Need for DeepGEMM
DeepGEMM addresses matrix multiplication challenges by offering a lightweight, high-performance, and user-friendly library for diverse GEMM operations.
- Fills a critical need for optimized FP8 GEMM in the AI community.
- High performance with a small memory footprint.
- Supports both dense and MoE layouts.
- Crucial for large-scale AI model training and execution.
- Optimizes MoE architectures with specialized GEMM types.
- Directly enhances DeepSeek's AI models.
- Benefits the broader AI development ecosystem.
Key Features of DeepGEMM
DeepGEMM's strengths include:
- High Performance: Achieves up to 1350 FP8 TFLOPS on NVIDIA Hopper GPUs.
- Lightweight Design: Minimal dependencies for simplified usage.
- Just-In-Time Compilation: Compiles kernels at runtime for streamlined user experience.
- Concise Core Logic: Approximately 300 lines of core code, outperforming many expert-tuned kernels.
- Support for Diverse Layouts: Supports dense and two MoE layouts.
Performance Benchmarks
DeepGEMM's efficiency across various matrix configurations is shown below:
M | N | K | Computation | Memory Bandwidth | Speedup |
---|---|---|---|---|---|
64 | 2112 | 7168 | 206 TFLOPS | 1688 GB/s | 2.7x |
128 | 7168 | 2048 | 510 TFLOPS | 2277 GB/s | 1.7x |
4096 | 4096 | 7168 | 1304 TFLOPS | 500 GB/s | 1.1x |
Table 1: DeepGEMM Performance Benchmarks
Installation Instructions
DeepGEMM installation is straightforward:
Step 1: Prerequisites
- Hopper architecture GPUs (sm_90a)
- Python 3.8
- CUDA 12.3 (recommended: 12.8 )
- PyTorch 2.1
- CUTLASS 3.6 (can be a Git submodule)
Step 2: Clone the Repository
git clone --recursive [email protected]:deepseek-ai/DeepGEMM.git
Step 3: Install the Library
python setup.py install
Step 4: Import DeepGEMM
import deep_gemm
See the DeepGEMM GitHub repository for detailed instructions.
Conclusion
DeepGEMM is a high-performance, user-friendly FP8 GEMM library ideal for advanced machine learning tasks. Its lightweight design, speed, and flexibility make it a valuable tool for AI developers. Check the Analytics Vidhya Blog for updates on DeepSeek's Day 4 release!
The above is the detailed content of DeepGEMM Released on Day 3 of DeepSeek Open Source Week. For more information, please follow other related articles on the PHP Chinese website!

Hot AI Tools

Undresser.AI Undress
AI-powered app for creating realistic nude photos

AI Clothes Remover
Online AI tool for removing clothes from photos.

Undress AI Tool
Undress images for free

Clothoff.io
AI clothes remover

Video Face Swap
Swap faces in any video effortlessly with our completely free AI face swap tool!

Hot Article

Hot Tools

Notepad++7.3.1
Easy-to-use and free code editor

SublimeText3 Chinese version
Chinese version, very easy to use

Zend Studio 13.0.1
Powerful PHP integrated development environment

Dreamweaver CS6
Visual web development tools

SublimeText3 Mac version
God-level code editing software (SublimeText3)

Hot Topics
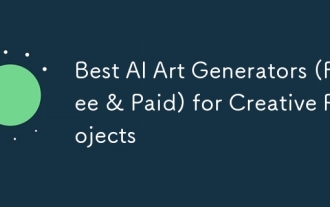
The article reviews top AI art generators, discussing their features, suitability for creative projects, and value. It highlights Midjourney as the best value for professionals and recommends DALL-E 2 for high-quality, customizable art.
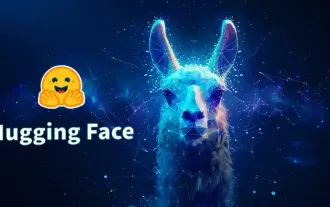
Meta's Llama 3.2: A Leap Forward in Multimodal and Mobile AI Meta recently unveiled Llama 3.2, a significant advancement in AI featuring powerful vision capabilities and lightweight text models optimized for mobile devices. Building on the success o
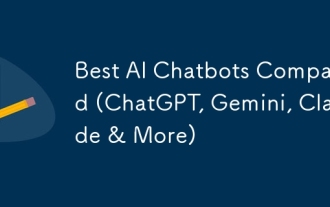
The article compares top AI chatbots like ChatGPT, Gemini, and Claude, focusing on their unique features, customization options, and performance in natural language processing and reliability.
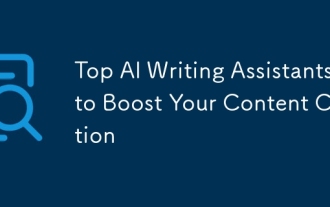
The article discusses top AI writing assistants like Grammarly, Jasper, Copy.ai, Writesonic, and Rytr, focusing on their unique features for content creation. It argues that Jasper excels in SEO optimization, while AI tools help maintain tone consist
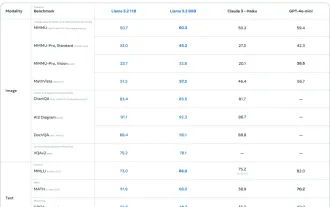
This week's AI landscape: A whirlwind of advancements, ethical considerations, and regulatory debates. Major players like OpenAI, Google, Meta, and Microsoft have unleashed a torrent of updates, from groundbreaking new models to crucial shifts in le
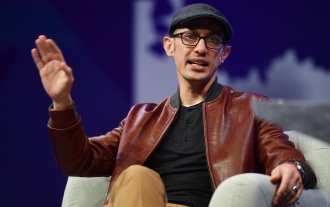
Shopify CEO Tobi Lütke's recent memo boldly declares AI proficiency a fundamental expectation for every employee, marking a significant cultural shift within the company. This isn't a fleeting trend; it's a new operational paradigm integrated into p
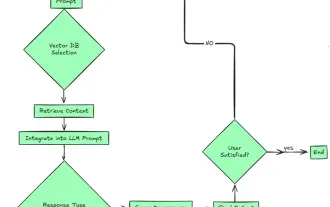
2024 witnessed a shift from simply using LLMs for content generation to understanding their inner workings. This exploration led to the discovery of AI Agents – autonomous systems handling tasks and decisions with minimal human intervention. Buildin
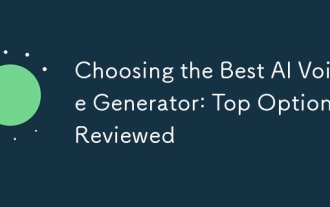
The article reviews top AI voice generators like Google Cloud, Amazon Polly, Microsoft Azure, IBM Watson, and Descript, focusing on their features, voice quality, and suitability for different needs.
