DeepSeek Launches FlashMLA
DeepSeek's groundbreaking open-source release: FlashMLA, a CUDA kernel accelerating LLMs. This optimized multi-latent attention (MLA) decoding kernel, specifically designed for Hopper GPUs, significantly boosts the speed and efficiency of AI model hosting. Key improvements include BF16 support and a paged KV cache (64-block size), resulting in impressive performance benchmarks.
? Day 1 of #OpenSourceWeek: FlashMLA
DeepSeek proudly unveils FlashMLA, a high-efficiency MLA decoding kernel for Hopper GPUs. Optimized for variable-length sequences and now in production.
✅ BF16 support
✅ Paged KV cache (block size 64)
⚡ 3000 GB/s memory-bound & 580 TFLOPS…— DeepSeek (@deepseek_ai) February 24, 2025
Key Features:
- BF16 Precision: Enables efficient computation while maintaining numerical stability.
- Paged KV Cache (64-block size): Enhances memory efficiency and reduces latency, especially crucial for large models.
These optimizations achieve up to 3000 GB/s memory bandwidth and 580 TFLOPS in computation-bound scenarios on H800 SXM5 GPUs using CUDA 12.6. This dramatically improves AI inference performance. Previously used in DeepSeek Models, FlashMLA now accelerates DeepSeek AI's R1 V3.
Table of Contents:
- What is FlashMLA?
- Understanding Multi-head Latent Attention (MLA)
- Standard Multi-Head Attention Limitations
- MLA's Memory Optimization Strategy
- Key-Value Caching and Autoregressive Decoding
- KV Caching Mechanics
- Addressing Memory Challenges
- FlashMLA's Role in DeepSeek Models
- NVIDIA Hopper Architecture
- Performance Analysis and Implications
- Conclusion
What is FlashMLA?
FlashMLA is a highly optimized MLA decoding kernel built for NVIDIA Hopper GPUs. Its design prioritizes speed and efficiency, reflecting DeepSeek's commitment to scalable AI model acceleration.
Hardware and Software Requirements:
- Hopper architecture GPUs (e.g., H800 SXM5)
- CUDA 12.3
- PyTorch 2.0
Performance Benchmarks:
FlashMLA demonstrates exceptional performance:
- Memory Bandwidth: Up to 3000 GB/s (approaching the H800 SXM5 theoretical peak).
- Computational Throughput: Up to 580 TFLOPS for BF16 matrix multiplication (significantly exceeding the H800's theoretical peak).
This superior performance makes FlashMLA ideal for demanding AI workloads.
Understanding Multi-head Latent Attention (MLA)
MLA, introduced with DeepSeek-V2, addresses the memory limitations of standard multi-head attention (MHA) by using a low-rank factorized projection matrix. Unlike methods like Group-Query Attention, MLA improves performance while reducing memory overhead.
Standard Multi-Head Attention Limitations:
MHA's KV cache scales linearly with sequence length, creating a memory bottleneck for long sequences. The cache size is calculated as: seq_len * n_h * d_h
(where n_h
is the number of attention heads and d_h
is the head dimension).
MLA's Memory Optimization:
MLA compresses keys and values into a smaller latent vector (c_t
), reducing the KV cache size to seq_len * d_c
(where d_c
is the latent vector dimension). This significantly reduces memory usage (up to 93.3% reduction in DeepSeek-V2).
Key-Value Caching and Autoregressive Decoding
KV caching accelerates autoregressive decoding by reusing previously computed key-value pairs. However, this increases memory usage.
Addressing Memory Challenges:
Techniques like Multi-Query Attention (MQA) and Grouped-Query Attention (GQA) mitigate the memory issues associated with KV caching.
FlashMLA's Role in DeepSeek Models:
FlashMLA powers DeepSeek's R1 and V3 models, enabling efficient large-scale AI applications.
NVIDIA Hopper Architecture
NVIDIA Hopper is a high-performance GPU architecture designed for AI and HPC workloads. Its innovations, such as the Transformer Engine and second-generation MIG, enable exceptional speed and scalability.
Performance Analysis and Implications
FlashMLA achieves 580 TFLOPS for BF16 matrix multiplication, more than double the H800 GPU's theoretical peak. This demonstrates highly efficient utilization of GPU resources.
Conclusion
FlashMLA represents a major advancement in AI inference efficiency, particularly for Hopper GPUs. Its MLA optimization, combined with BF16 support and paged KV caching, delivers remarkable performance improvements. This makes large-scale AI models more accessible and cost-effective, setting a new benchmark for model efficiency.
The above is the detailed content of DeepSeek Launches FlashMLA. For more information, please follow other related articles on the PHP Chinese website!

Hot AI Tools

Undresser.AI Undress
AI-powered app for creating realistic nude photos

AI Clothes Remover
Online AI tool for removing clothes from photos.

Undress AI Tool
Undress images for free

Clothoff.io
AI clothes remover

Video Face Swap
Swap faces in any video effortlessly with our completely free AI face swap tool!

Hot Article

Hot Tools

Notepad++7.3.1
Easy-to-use and free code editor

SublimeText3 Chinese version
Chinese version, very easy to use

Zend Studio 13.0.1
Powerful PHP integrated development environment

Dreamweaver CS6
Visual web development tools

SublimeText3 Mac version
God-level code editing software (SublimeText3)

Hot Topics










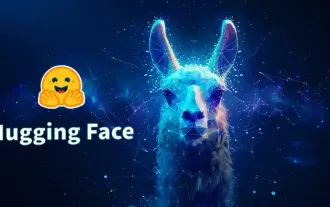
Meta's Llama 3.2: A Leap Forward in Multimodal and Mobile AI Meta recently unveiled Llama 3.2, a significant advancement in AI featuring powerful vision capabilities and lightweight text models optimized for mobile devices. Building on the success o
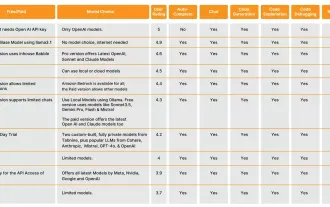
Hey there, Coding ninja! What coding-related tasks do you have planned for the day? Before you dive further into this blog, I want you to think about all your coding-related woes—better list those down. Done? – Let’
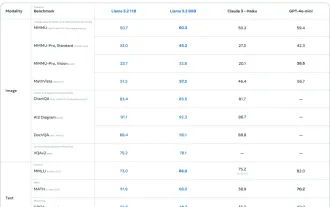
This week's AI landscape: A whirlwind of advancements, ethical considerations, and regulatory debates. Major players like OpenAI, Google, Meta, and Microsoft have unleashed a torrent of updates, from groundbreaking new models to crucial shifts in le
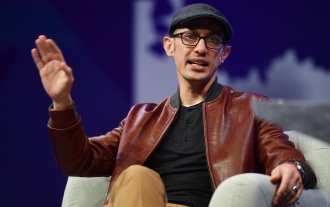
Shopify CEO Tobi Lütke's recent memo boldly declares AI proficiency a fundamental expectation for every employee, marking a significant cultural shift within the company. This isn't a fleeting trend; it's a new operational paradigm integrated into p
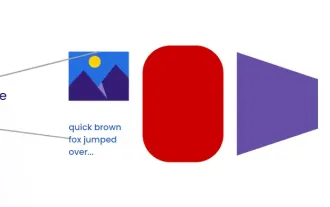
Introduction Imagine walking through an art gallery, surrounded by vivid paintings and sculptures. Now, what if you could ask each piece a question and get a meaningful answer? You might ask, “What story are you telling?
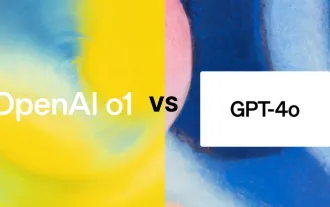
Introduction OpenAI has released its new model based on the much-anticipated “strawberry” architecture. This innovative model, known as o1, enhances reasoning capabilities, allowing it to think through problems mor
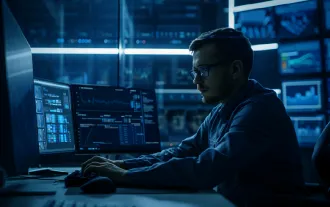
SQL's ALTER TABLE Statement: Dynamically Adding Columns to Your Database In data management, SQL's adaptability is crucial. Need to adjust your database structure on the fly? The ALTER TABLE statement is your solution. This guide details adding colu
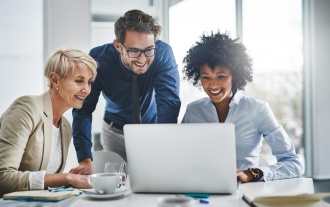
For those of you who might be new to my column, I broadly explore the latest advances in AI across the board, including topics such as embodied AI, AI reasoning, high-tech breakthroughs in AI, prompt engineering, training of AI, fielding of AI, AI re
