


How LLMs Work: Pre-Training to Post-Training, Neural Networks, Hallucinations, and Inference
Unveiling the Magic Behind Large Language Models (LLMs): A Two-Part Exploration
Large Language Models (LLMs) often appear magical, but their inner workings are surprisingly systematic. This two-part series demystifies LLMs, explaining their construction, training, and refinement into the AI systems we use today. Inspired by Andrej Karpathy's insightful (and lengthy!) YouTube video, this condensed version provides the core concepts in a more accessible format. While Karpathy's video is highly recommended (800,000 views in just 10 days!), this 10-minute read distills the key takeaways from the first 1.5 hours.
Part 1: From Raw Data to Base Model
LLM development involves two crucial phases: pre-training and post-training.
1. Pre-training: Teaching the Language
Before generating text, an LLM must learn language structure. This computationally intensive pre-training process involves several steps:
- Data Acquisition and Preprocessing: Massive, diverse datasets are gathered, often including sources like Common Crawl (250 billion web pages). However, raw data requires cleaning to remove spam, duplicates, and low-quality content. Services like FineWeb offer preprocessed versions available on Hugging Face.
- Tokenization: Text is converted into numerical tokens (words, subwords, or characters) for neural network processing. GPT-4, for example, utilizes 100,277 unique tokens. Tools like Tiktokenizer visualize this process.
- Neural Network Training: The neural network learns to predict the next token in a sequence based on context. This involves billions of iterations, adjusting parameters (weights) via backpropagation to improve prediction accuracy. The network's architecture dictates how input tokens are processed to generate outputs.
The resulting base model understands word relationships and statistical patterns but lacks real-world task optimization. It functions like an advanced autocomplete, predicting based on probability but with limited instruction-following capabilities. In-context learning, using examples within prompts, can be employed, but further training is necessary.
2. Post-training: Refining for Practical Use
Base models are refined through post-training using smaller, specialized datasets. This isn't explicit programming but rather implicit instruction through structured examples.
Post-training methods include:
- Instruction/Conversation Fine-tuning: Teaches the model to follow instructions, engage in conversations, adhere to safety guidelines, and refuse harmful requests (e.g., InstructGPT).
- Domain-Specific Fine-tuning: Adapts the model for specific fields (medicine, law, programming).
Special tokens are introduced to delineate user input and AI responses.
Inference: Generating Text
Inference, performed at any stage, evaluates model learning. The model assigns probabilities to potential next tokens and samples from this distribution, creating text not explicitly in the training data but statistically consistent with it. This stochastic process allows for varied outputs from the same input.
Hallucinations: Addressing False Information
Hallucinations, where LLMs generate false information, arise from their probabilistic nature. They don't "know" facts but predict likely word sequences. Mitigation strategies include:
- "I don't know" Training: Explicitly training the model to recognize knowledge gaps through self-interrogation and automated question generation.
- Web Search Integration: Extending knowledge by accessing external search tools, incorporating results into the model's context window.
LLMs access knowledge through vague recollections (patterns from pre-training) and working memory (information in the context window). System prompts can establish a consistent model identity.
Conclusion (Part 1)
This part explored the foundational aspects of LLM development. Part 2 will delve into reinforcement learning and examine cutting-edge models. Your questions and suggestions are welcome!
The above is the detailed content of How LLMs Work: Pre-Training to Post-Training, Neural Networks, Hallucinations, and Inference. For more information, please follow other related articles on the PHP Chinese website!

Hot AI Tools

Undresser.AI Undress
AI-powered app for creating realistic nude photos

AI Clothes Remover
Online AI tool for removing clothes from photos.

Undress AI Tool
Undress images for free

Clothoff.io
AI clothes remover

Video Face Swap
Swap faces in any video effortlessly with our completely free AI face swap tool!

Hot Article

Hot Tools

Notepad++7.3.1
Easy-to-use and free code editor

SublimeText3 Chinese version
Chinese version, very easy to use

Zend Studio 13.0.1
Powerful PHP integrated development environment

Dreamweaver CS6
Visual web development tools

SublimeText3 Mac version
God-level code editing software (SublimeText3)

Hot Topics










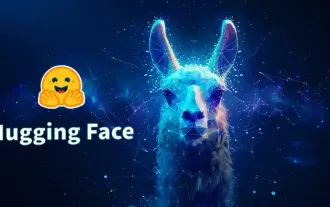
Meta's Llama 3.2: A Leap Forward in Multimodal and Mobile AI Meta recently unveiled Llama 3.2, a significant advancement in AI featuring powerful vision capabilities and lightweight text models optimized for mobile devices. Building on the success o
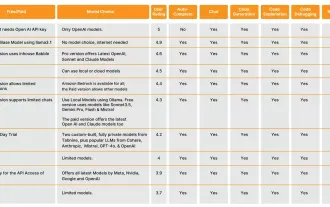
Hey there, Coding ninja! What coding-related tasks do you have planned for the day? Before you dive further into this blog, I want you to think about all your coding-related woes—better list those down. Done? – Let’
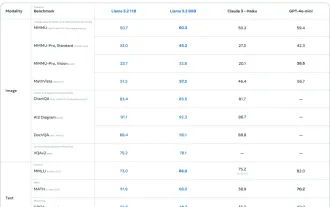
This week's AI landscape: A whirlwind of advancements, ethical considerations, and regulatory debates. Major players like OpenAI, Google, Meta, and Microsoft have unleashed a torrent of updates, from groundbreaking new models to crucial shifts in le
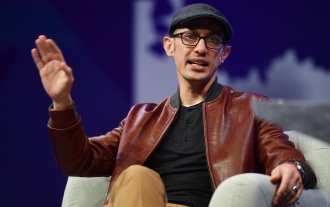
Shopify CEO Tobi Lütke's recent memo boldly declares AI proficiency a fundamental expectation for every employee, marking a significant cultural shift within the company. This isn't a fleeting trend; it's a new operational paradigm integrated into p
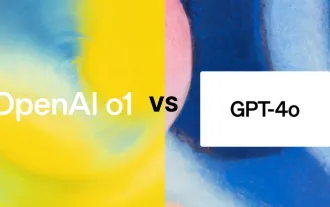
Introduction OpenAI has released its new model based on the much-anticipated “strawberry” architecture. This innovative model, known as o1, enhances reasoning capabilities, allowing it to think through problems mor
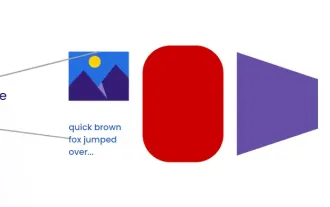
Introduction Imagine walking through an art gallery, surrounded by vivid paintings and sculptures. Now, what if you could ask each piece a question and get a meaningful answer? You might ask, “What story are you telling?
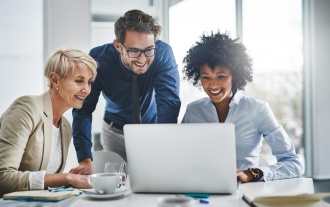
For those of you who might be new to my column, I broadly explore the latest advances in AI across the board, including topics such as embodied AI, AI reasoning, high-tech breakthroughs in AI, prompt engineering, training of AI, fielding of AI, AI re
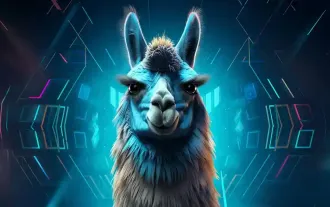
Meta's Llama 3.2: A Multimodal AI Powerhouse Meta's latest multimodal model, Llama 3.2, represents a significant advancement in AI, boasting enhanced language comprehension, improved accuracy, and superior text generation capabilities. Its ability t
