


Understanding the Evolution of ChatGPT: Part 3- Insights from Codex and InstructGPT
This article delves into the practical aspects of fine-tuning large language models (LLMs), focusing on Codex and InstructGPT as prime examples. It's the third in a series exploring GPT models, building upon previous discussions of pre-training and scaling.
Fine-tuning is crucial because while pre-trained LLMs are versatile, they often fall short of specialized models tailored to specific tasks. Furthermore, even powerful models like GPT-3 may struggle with complex instructions and maintaining safety and ethical standards. This necessitates fine-tuning strategies.
The article highlights two key fine-tuning challenges: adapting to new modalities (like Codex's adaptation to code generation) and aligning the model with human preferences (as demonstrated by InstructGPT). Both require careful consideration of data collection, model architecture, objective functions, and evaluation metrics.
Codex: Fine-tuning for Code Generation
The article emphasizes the inadequacy of traditional metrics like BLEU score for evaluating code generation. It introduces "functional correctness" and the pass@k metric, offering a more robust evaluation method. The creation of the HumanEval dataset, comprising hand-written programming problems with unit tests, is also highlighted. Data cleaning strategies specific to code are discussed, along with the importance of adapting tokenizers to handle the unique characteristics of programming languages (e.g., whitespace encoding). The article presents results demonstrating Codex's superior performance compared to GPT-3 on HumanEval and explores the impact of model size and temperature on performance.
InstructGPT and ChatGPT: Aligning with Human Preferences
The article defines alignment as the model exhibiting helpfulness, honesty, and harmlessness. It explains how these qualities are translated into measurable aspects like instruction following, hallucination rate, and bias/toxicity. The use of Reinforcement Learning from Human Feedback (RLHF) is detailed, outlining the three stages: collecting human feedback, training a reward model, and optimizing the policy using Proximal Policy Optimization (PPO). The article emphasizes the importance of data quality control in the human feedback collection process. Results showcasing InstructGPT's improved alignment, reduced hallucination, and mitigation of performance regressions are presented.
Summary and Best Practices
The article concludes by summarizing key considerations for fine-tuning LLMs, including defining desired behaviors, evaluating performance, collecting and cleaning data, adapting model architecture, and mitigating potential negative consequences. It encourages careful consideration of hyperparameter tuning and emphasizes the iterative nature of the fine-tuning process.
The above is the detailed content of Understanding the Evolution of ChatGPT: Part 3- Insights from Codex and InstructGPT. For more information, please follow other related articles on the PHP Chinese website!

Hot AI Tools

Undresser.AI Undress
AI-powered app for creating realistic nude photos

AI Clothes Remover
Online AI tool for removing clothes from photos.

Undress AI Tool
Undress images for free

Clothoff.io
AI clothes remover

Video Face Swap
Swap faces in any video effortlessly with our completely free AI face swap tool!

Hot Article

Hot Tools

Notepad++7.3.1
Easy-to-use and free code editor

SublimeText3 Chinese version
Chinese version, very easy to use

Zend Studio 13.0.1
Powerful PHP integrated development environment

Dreamweaver CS6
Visual web development tools

SublimeText3 Mac version
God-level code editing software (SublimeText3)

Hot Topics










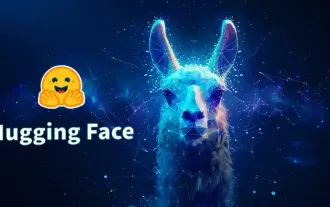
Meta's Llama 3.2: A Leap Forward in Multimodal and Mobile AI Meta recently unveiled Llama 3.2, a significant advancement in AI featuring powerful vision capabilities and lightweight text models optimized for mobile devices. Building on the success o
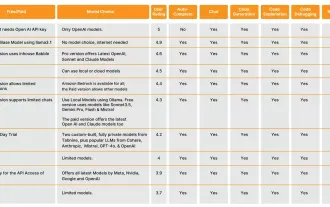
Hey there, Coding ninja! What coding-related tasks do you have planned for the day? Before you dive further into this blog, I want you to think about all your coding-related woes—better list those down. Done? – Let’
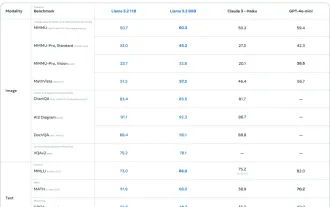
This week's AI landscape: A whirlwind of advancements, ethical considerations, and regulatory debates. Major players like OpenAI, Google, Meta, and Microsoft have unleashed a torrent of updates, from groundbreaking new models to crucial shifts in le
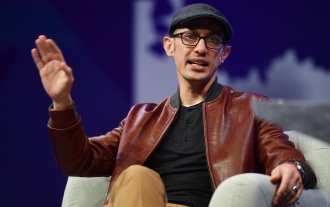
Shopify CEO Tobi Lütke's recent memo boldly declares AI proficiency a fundamental expectation for every employee, marking a significant cultural shift within the company. This isn't a fleeting trend; it's a new operational paradigm integrated into p
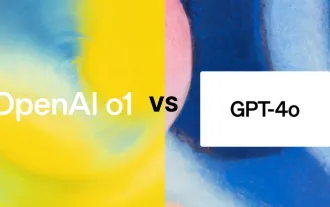
Introduction OpenAI has released its new model based on the much-anticipated “strawberry” architecture. This innovative model, known as o1, enhances reasoning capabilities, allowing it to think through problems mor
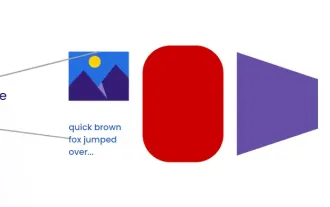
Introduction Imagine walking through an art gallery, surrounded by vivid paintings and sculptures. Now, what if you could ask each piece a question and get a meaningful answer? You might ask, “What story are you telling?
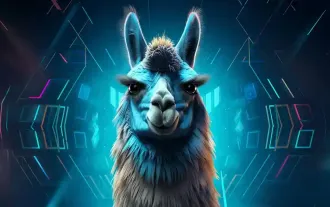
Meta's Llama 3.2: A Multimodal AI Powerhouse Meta's latest multimodal model, Llama 3.2, represents a significant advancement in AI, boasting enhanced language comprehension, improved accuracy, and superior text generation capabilities. Its ability t
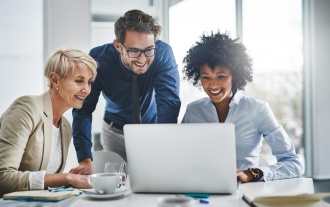
For those of you who might be new to my column, I broadly explore the latest advances in AI across the board, including topics such as embodied AI, AI reasoning, high-tech breakthroughs in AI, prompt engineering, training of AI, fielding of AI, AI re
