Is ReFT All We Needed?
ReFT: A Revolutionary Approach to Fine-tuning LLMs
ReFT (Representation Finetuning), introduced in Stanford's May 2024 paper, offers a groundbreaking method for efficiently fine-tuning large language models (LLMs). Its potential was immediately apparent, further highlighted by Oxen.ai's July 2024 experiment fine-tuning Llama3 (8B) on a single Nvidia A10 GPU in just 14 minutes.
Unlike existing Parameter-Efficient Fine-Tuning (PEFT) methods like LoRA, which modify model weights or input, ReFT leverages the Distributed Interchange Intervention (DII) method. DII projects embeddings into a lower-dimensional subspace, enabling fine-tuning through this subspace.
This article first reviews popular PEFT algorithms (LoRA, Prompt Tuning, Prefix Tuning), then explains DII, before delving into ReFT and its experimental results.
Parameter-Efficient Fine-Tuning (PEFT) Techniques
Hugging Face provides a comprehensive overview of PEFT techniques. Let's briefly summarize key methods:
LoRA (Low-Rank Adaptation): Introduced in 2021, LoRA's simplicity and generalizability have made it a leading technique for fine-tuning LLMs and diffusion models. Instead of adjusting all layer weights, LoRA adds low-rank matrices, significantly reducing trainable parameters (often less than 0.3%), accelerating training and minimizing GPU memory usage.
Prompt Tuning: This method uses "soft prompts"—learnable task-specific embeddings—as prefixes, enabling efficient multi-task prediction without duplicating the model for each task.
Prefix Tuning (P-Tuning v2): Addressing limitations of prompt tuning at scale, Prefix Tuning adds trainable prompt embeddings to various layers, allowing task-specific learning at different levels.
LoRA's robustness and efficiency make it the most widely used PEFT method for LLMs. A detailed empirical comparison can be found in this paper.
Distributed Interchange Intervention (DII)
DII is rooted in causal abstraction, a framework using intervention between a high-level (causal) model and a low-level (neural network) model to assess alignment. DII projects both models into subspaces via orthogonal projections, creating an intervened model through rotation operations. A detailed visual example is available here.
The DII process can be mathematically represented as:
where R
represents orthogonal projections, and the distributed alignment search (DAS) optimizes the subspace to maximize the probability of expected counterfactual outputs post-intervention.
ReFT – Representation Finetuning
ReFT intervenes in the model's hidden representation within a lower-dimensional space. The illustration below shows the intervention (phi) applied to layer L and position P:
LoReFT (Low-rank Linear Subspace Reft) introduces a learned projected source:
where h
is the hidden representation, and Rs
edits h
in the low-dimensional space spanned by R
. The LoReFT integration into a neural network layer is shown below:
During LLM fine-tuning, the LLM parameters remain frozen, and only the projection parameters (phi={R, W, b}
) are trained.
Experimental Results
The original ReFT paper presents comparative experiments against full fine-tuning (FT), LoRA, and Prefix Tuning across various benchmarks. ReFT techniques consistently outperform existing methods, reducing parameters by at least 90% while achieving superior performance.
Discussion
ReFT's appeal stems from its superior performance with Llama-family models across diverse benchmarks and its grounding in causal abstraction, which aids model interpretability. ReFT demonstrates that a linear subspace distributed across neurons can effectively control numerous tasks, offering valuable insights into LLMs.
References
- Wu et al., ReFT: Representation Finetuning for Language Models
- Hu et al., LoRA: Low-Rank Adaptation of Large Language Models
- Zhuang et al., Time-Varying LoRA
- Liu et al., P-tuning v2
- Geiger et al., Finding alignments between interpretable causal variables and distributed neural representations
- Lester et al., The power of scale for parameter-efficient prompt tuning
- Pu et al., Empirical analysis of the strengths and weaknesses of Peft techniques for LLMs
(Note: Please replace the bracketed https://www.php.cn/link/6c11cb78b7bbb5c22d5f5271b5494381
placeholders with the actual links to the research papers.)
The above is the detailed content of Is ReFT All We Needed?. For more information, please follow other related articles on the PHP Chinese website!

Hot AI Tools

Undresser.AI Undress
AI-powered app for creating realistic nude photos

AI Clothes Remover
Online AI tool for removing clothes from photos.

Undress AI Tool
Undress images for free

Clothoff.io
AI clothes remover

Video Face Swap
Swap faces in any video effortlessly with our completely free AI face swap tool!

Hot Article

Hot Tools

Notepad++7.3.1
Easy-to-use and free code editor

SublimeText3 Chinese version
Chinese version, very easy to use

Zend Studio 13.0.1
Powerful PHP integrated development environment

Dreamweaver CS6
Visual web development tools

SublimeText3 Mac version
God-level code editing software (SublimeText3)

Hot Topics










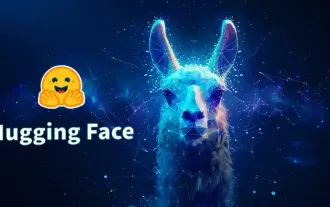
Meta's Llama 3.2: A Leap Forward in Multimodal and Mobile AI Meta recently unveiled Llama 3.2, a significant advancement in AI featuring powerful vision capabilities and lightweight text models optimized for mobile devices. Building on the success o
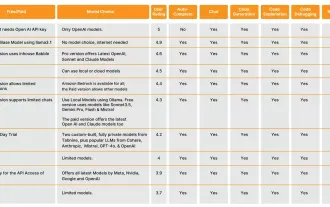
Hey there, Coding ninja! What coding-related tasks do you have planned for the day? Before you dive further into this blog, I want you to think about all your coding-related woes—better list those down. Done? – Let’
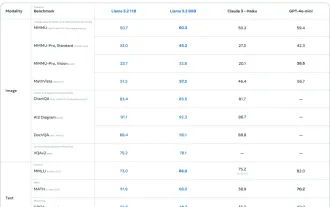
This week's AI landscape: A whirlwind of advancements, ethical considerations, and regulatory debates. Major players like OpenAI, Google, Meta, and Microsoft have unleashed a torrent of updates, from groundbreaking new models to crucial shifts in le
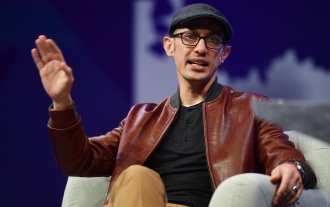
Shopify CEO Tobi Lütke's recent memo boldly declares AI proficiency a fundamental expectation for every employee, marking a significant cultural shift within the company. This isn't a fleeting trend; it's a new operational paradigm integrated into p
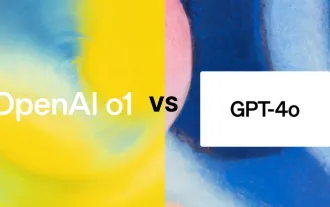
Introduction OpenAI has released its new model based on the much-anticipated “strawberry” architecture. This innovative model, known as o1, enhances reasoning capabilities, allowing it to think through problems mor
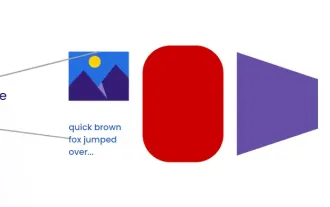
Introduction Imagine walking through an art gallery, surrounded by vivid paintings and sculptures. Now, what if you could ask each piece a question and get a meaningful answer? You might ask, “What story are you telling?
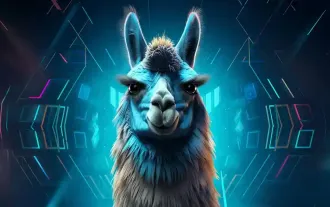
Meta's Llama 3.2: A Multimodal AI Powerhouse Meta's latest multimodal model, Llama 3.2, represents a significant advancement in AI, boasting enhanced language comprehension, improved accuracy, and superior text generation capabilities. Its ability t
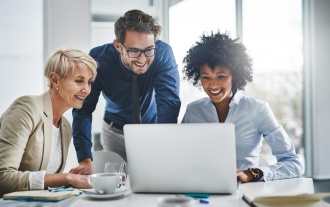
For those of you who might be new to my column, I broadly explore the latest advances in AI across the board, including topics such as embodied AI, AI reasoning, high-tech breakthroughs in AI, prompt engineering, training of AI, fielding of AI, AI re
