


To predict protein-DNA binding specificity, USC team develops new geometric deep learning method
Editor | Radish Skin
Predicting protein-DNA binding specificity is a challenging but crucial task that helps understand gene regulation. Protein-DNA complexes typically bind to selected DNA targets, whereas proteins bind to a broad range of DNA sequences with varying degrees of binding specificity. This information is not directly accessible in a single structure.
To obtain this information, researchers from the University of Southern California and the University of Washington proposed the Deep Binding Specificity Predictor (DeepPBS), a geometric deep learning model designed to Prediction of binding specificity based on protein-DNA structure.
DeepPBS can extract interpretable protein heavy atom importance scores of interface residues. These scores were validated by mutagenesis experiments when aggregated at the protein residue level. Applied to designed proteins targeting specific DNA sequences, DeepPBS was shown to predict experimentally measured binding specificities.
The study was titled "Geometric deep learning of protein–DNA binding specificity" and was published in "Nature Methods" on August 5, 2024.
Transcription factors regulate life processes by binding to specific DNA sequences. This binding mechanism includes electrostatic interactions, deoxyribose stacking effects, and the formation of hydrogen bonds.
Protein-DNA structural information is usually obtained through experimental methods such as X-ray crystallography, nuclear magnetic resonance spectroscopy or cryo-electron microscopy, and is stored in the Protein Data Bank (PDB). These structures typically demonstrate bound DNA sequences and their physicochemical interactions, but do not cover all possible binding sequences.
On the other hand, high-throughput experiments such as protein binding microarrays, SELEX-seq, etc. are able to capture the range of potential binding sequences but lack structural information.
Therefore, combining structural data and high-throughput experimental data is crucial to fully understand the binding specificity of transcription factors.
Currently, predicting the binding specificity of a specific protein sequence within a protein family remains a challenging and unsolved problem. This difficulty is exacerbated by structural changes in the context and the vast mechanistic diversity.
"The structure of a protein-DNA complex contains proteins that typically bind to a single DNA sequence. In order to understand gene regulation, it is important to understand the binding specificity of a protein to any DNA sequence or genomic region." said Professor Remo Rohs of the University of Southern California. .
In the latest study, researchers from the University of Southern California and the University of Washington introduced Binding Specificity Deep Predictors (DeepPBS).
Rohs explained: "DeepPBS is an artificial intelligence tool that replaces high-throughput sequencing or structural biology experiments to reveal protein-DNA binding specificity."
This deep learning model aims to capture the physicochemical and geometric context of protein-DNA interactions to predict binding specificity, expressed as a position weight matrix (PWM) based on a given protein-DNA structure. DeepPBS functions across protein families and serves as a bridge between structure determination and binding specificity determination experiments.
Illustration: Performance of DeepPBS for predicting binding specificity across protein families. (Source: paper)
Inputs to DeepPBS are not limited to experimental structures. The rapid development of protein structure prediction methods, including AlphaFold, OpenFold, and RoseTTAFold, and protein-DNA complex modelers, such as RoseTTAFoldNA (RFNA), RoseTTAFold All-Atom, MELD-DNA, and AlphaFold3, has resulted in structural data available for analysis The numbers are growing exponentially.
This scenario highlights the growing need for universal computational models for analyzing protein-DNA structures. The researchers demonstrate how DeepPBS can be used in conjunction with structure prediction methods to predict the specificity of proteins for which no experimental structure is available.
Additionally, the design of protein-DNA complexes can be improved by using DeepPBS feedback to optimize binding to DNA. The researchers show that this pipeline performs comparably to a recent family-specific model, rCLAMPS, while being more general: Specifically, DeepPBS is not restricted by protein families, can handle biological assemblies, and can predict DNA side chain preferences.
Illustration: Application of DeepPBS in predicting the structure of protein-DNA complexes. (Source: paper)
In terms of interpretability, the “relative importance” (RI) scores of different heavy atoms in proteins interacting with DNA can be extracted from DeepPBS.
As a case study of proteins important for cancer development, the researchers analyzed the p53-DNA interface through these RI scores and linked them to existing literature for validation.
Also, DeepPBS scores agree well with existing knowledge and can be aggregated to produce reasonable agreement with alanine scanning mutagenesis experiments.
Illustration: Taking the visualization of DeepPBS importance scores in the p53-DNA interface as an example to study and conduct experimental verification. (Source: paper)
In additional proof-of-principle studies, researchers applied DeepPBS to in silico-designed protein-DNA complexes targeting specific DNA sequences from a recent experiment combining structural design and DNA mutagenesis research. DeepPBS can also be used to analyze molecular simulation trajectories.
"It is important for researchers to find a method that works for all proteins and is not limited to a well-studied protein family. This method also allows us to design new proteins." Rohs said.
Illustration: Applying DeepPBS to in silico designed HTH scaffolds targeting specific DNA sequences. (Source: paper)
The current version of DeepPBS has inherent limitations. It is tailored for double-stranded DNA and does not yet work with single-stranded DNA, RNA, or chemically modified bases.
However, the model has the potential to be extended to accommodate these different scenarios as well as other polymer-polymer interactions, and potentially to mechanistic mutations. The DeepPBS architecture can be optimized and extended in terms of application and engineering enhancements.
Nevertheless, Rohs said DeepPBS will have a wide range of applications. This new research approach may accelerate the design of new drugs and treatments that target specific mutations in cancer cells, as well as lead to new discoveries in synthetic biology and applications in RNA research.
DeepPBS: https://deeppbs.usc.edu
The above is the detailed content of To predict protein-DNA binding specificity, USC team develops new geometric deep learning method. For more information, please follow other related articles on the PHP Chinese website!

Hot AI Tools

Undresser.AI Undress
AI-powered app for creating realistic nude photos

AI Clothes Remover
Online AI tool for removing clothes from photos.

Undress AI Tool
Undress images for free

Clothoff.io
AI clothes remover

Video Face Swap
Swap faces in any video effortlessly with our completely free AI face swap tool!

Hot Article

Hot Tools

Notepad++7.3.1
Easy-to-use and free code editor

SublimeText3 Chinese version
Chinese version, very easy to use

Zend Studio 13.0.1
Powerful PHP integrated development environment

Dreamweaver CS6
Visual web development tools

SublimeText3 Mac version
God-level code editing software (SublimeText3)

Hot Topics










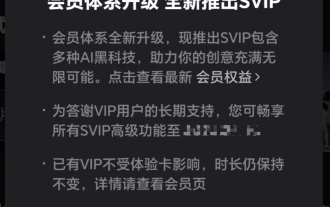
This site reported on June 27 that Jianying is a video editing software developed by FaceMeng Technology, a subsidiary of ByteDance. It relies on the Douyin platform and basically produces short video content for users of the platform. It is compatible with iOS, Android, and Windows. , MacOS and other operating systems. Jianying officially announced the upgrade of its membership system and launched a new SVIP, which includes a variety of AI black technologies, such as intelligent translation, intelligent highlighting, intelligent packaging, digital human synthesis, etc. In terms of price, the monthly fee for clipping SVIP is 79 yuan, the annual fee is 599 yuan (note on this site: equivalent to 49.9 yuan per month), the continuous monthly subscription is 59 yuan per month, and the continuous annual subscription is 499 yuan per year (equivalent to 41.6 yuan per month) . In addition, the cut official also stated that in order to improve the user experience, those who have subscribed to the original VIP
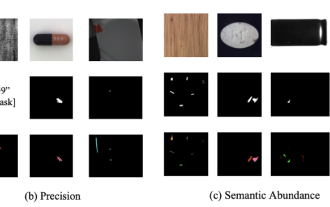
In modern manufacturing, accurate defect detection is not only the key to ensuring product quality, but also the core of improving production efficiency. However, existing defect detection datasets often lack the accuracy and semantic richness required for practical applications, resulting in models unable to identify specific defect categories or locations. In order to solve this problem, a top research team composed of Hong Kong University of Science and Technology Guangzhou and Simou Technology innovatively developed the "DefectSpectrum" data set, which provides detailed and semantically rich large-scale annotation of industrial defects. As shown in Table 1, compared with other industrial data sets, the "DefectSpectrum" data set provides the most defect annotations (5438 defect samples) and the most detailed defect classification (125 defect categories
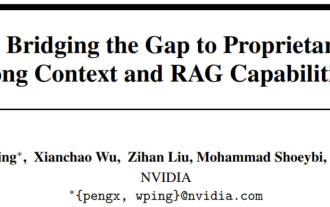
The open LLM community is an era when a hundred flowers bloom and compete. You can see Llama-3-70B-Instruct, QWen2-72B-Instruct, Nemotron-4-340B-Instruct, Mixtral-8x22BInstruct-v0.1 and many other excellent performers. Model. However, compared with proprietary large models represented by GPT-4-Turbo, open models still have significant gaps in many fields. In addition to general models, some open models that specialize in key areas have been developed, such as DeepSeek-Coder-V2 for programming and mathematics, and InternVL for visual-language tasks.
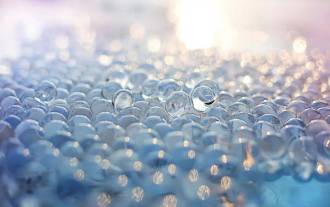
Editor |KX To this day, the structural detail and precision determined by crystallography, from simple metals to large membrane proteins, are unmatched by any other method. However, the biggest challenge, the so-called phase problem, remains retrieving phase information from experimentally determined amplitudes. Researchers at the University of Copenhagen in Denmark have developed a deep learning method called PhAI to solve crystal phase problems. A deep learning neural network trained using millions of artificial crystal structures and their corresponding synthetic diffraction data can generate accurate electron density maps. The study shows that this deep learning-based ab initio structural solution method can solve the phase problem at a resolution of only 2 Angstroms, which is equivalent to only 10% to 20% of the data available at atomic resolution, while traditional ab initio Calculation
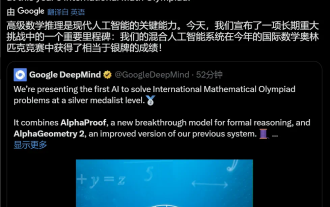
For AI, Mathematical Olympiad is no longer a problem. On Thursday, Google DeepMind's artificial intelligence completed a feat: using AI to solve the real question of this year's International Mathematical Olympiad IMO, and it was just one step away from winning the gold medal. The IMO competition that just ended last week had six questions involving algebra, combinatorics, geometry and number theory. The hybrid AI system proposed by Google got four questions right and scored 28 points, reaching the silver medal level. Earlier this month, UCLA tenured professor Terence Tao had just promoted the AI Mathematical Olympiad (AIMO Progress Award) with a million-dollar prize. Unexpectedly, the level of AI problem solving had improved to this level before July. Do the questions simultaneously on IMO. The most difficult thing to do correctly is IMO, which has the longest history, the largest scale, and the most negative
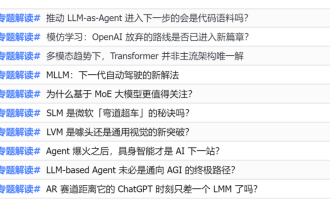
In 2023, almost every field of AI is evolving at an unprecedented speed. At the same time, AI is constantly pushing the technological boundaries of key tracks such as embodied intelligence and autonomous driving. Under the multi-modal trend, will the situation of Transformer as the mainstream architecture of AI large models be shaken? Why has exploring large models based on MoE (Mixed of Experts) architecture become a new trend in the industry? Can Large Vision Models (LVM) become a new breakthrough in general vision? ...From the 2023 PRO member newsletter of this site released in the past six months, we have selected 10 special interpretations that provide in-depth analysis of technological trends and industrial changes in the above fields to help you achieve your goals in the new year. be prepared. This interpretation comes from Week50 2023
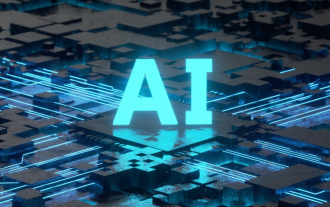
Editor |ScienceAI Question Answering (QA) data set plays a vital role in promoting natural language processing (NLP) research. High-quality QA data sets can not only be used to fine-tune models, but also effectively evaluate the capabilities of large language models (LLM), especially the ability to understand and reason about scientific knowledge. Although there are currently many scientific QA data sets covering medicine, chemistry, biology and other fields, these data sets still have some shortcomings. First, the data form is relatively simple, most of which are multiple-choice questions. They are easy to evaluate, but limit the model's answer selection range and cannot fully test the model's ability to answer scientific questions. In contrast, open-ended Q&A

Editor | Radish Skin Since the release of the powerful AlphaFold2 in 2021, scientists have been using protein structure prediction models to map various protein structures within cells, discover drugs, and draw a "cosmic map" of every known protein interaction. . Just now, Google DeepMind released the AlphaFold3 model, which can perform joint structure predictions for complexes including proteins, nucleic acids, small molecules, ions and modified residues. The accuracy of AlphaFold3 has been significantly improved compared to many dedicated tools in the past (protein-ligand interaction, protein-nucleic acid interaction, antibody-antigen prediction). This shows that within a single unified deep learning framework, it is possible to achieve
