


Nature sub-journal, 10 times faster, reverse protein sequence design method based on Transformer
Editor | Radish Skin
With advances in deep learning, protein design and engineering are advancing at an unprecedented rate. However, current models cannot naturally account for non-protein entities during the design process.
Here, researchers from the Ecole Polytechnique Fédérale de Lausanne (EPFL) in Switzerland proposed a deep learning method based entirely on geometric transformers of atomic coordinates and element names, which can backbone scaffolds based on the constraints imposed by different molecular environments. Predict protein sequences.
Using this method, researchers can produce enzymes with high thermal stability and catalytic activity with a high success rate. This is expected to increase the versatility of protein design pipelines to achieve desired functions.
The research was titled "Context-aware geometric deep learning for protein sequence design" and was published in "Nature Communications" on July 25, 2024.
Designing proteins to achieve functional tasks is a challenge with major biological, medical, biotechnology and materials science implications. A key application area is the design of protein therapeutics, where tailoring proteins to precisely target specific diseases may be more competitive than small molecule drugs. This approach could revolutionize the treatment of many health problems, from autoimmune diseases to cancer, providing more effective and personalized treatments.
In addition, designing enzyme functions is also another important challenge in protein design. Enzymes serve as natural catalysts and play key roles in biological processes. By designing new enzymes or modifying existing enzymes, it is possible to create catalysts that promote reactions that are rare or do not exist in nature. This has far-reaching implications for multiple industries, including pharmaceuticals and environmental technologies, such as synthesizing complex drug molecules or breaking down pollutants and plastics.
Deep learning methods significantly accelerate the success rate and diversity of protein design. However, while current protein design models can handle multiple protein chains, they perform poorly when dealing with non-protein entities, limiting their application scope.
To solve this problem, EPFL’s research team once introduced a deep learning model-Protein Structure Transformer (PeSTo), a geometric transformer architecture that acts on atomic point clouds.
PeSTo incorporates a transformer attention mechanism that utilizes scalar and vector states to represent atoms and can predict interactions at virtually any molecule-protein interface, including proteins, nucleic acids, lipids, ions, small ligands, cofactors, or carbohydrates.
In the latest work, the team took advantage of the unique capabilities of this model and introduced the PeSTo-based protein sequence generator model CARBonAra (Context-aware Amino acid Recovery from Backbone Atoms and heteroatoms).
CARBonAra is uniquely trained on structural data available in the PDB to predict amino acid confidence for all positions of a given backbone scaffold, which can be provided alone or with any kind and number of tools that help drive sequence design molecular complex.
Composition and Performance of CARBonAra
CARBonAra uses a deep learning model composed of geometric transformers to predict the likelihood of finding a given amino acid at each position in a protein sequence from an input backbone scaffold. CARBonAra takes as input the coordinates and elements of the backbone atoms (Cα, C, N, O) and adds virtual Cβ atoms using ideal bond angles and bond lengths. The geometry is described using the distance between each atom and the normalized relative displacement vector.
Geometric transformer operations encode the interactions of all neighboring atoms, and use transformers to process scalar and vector information and update the state of each atom. Finally, by aggregating atomic states from the atomic level to the residue level, the researchers trained the model to predict the amino acid confidence for each position in the protein sequence in the form of a position-specific scoring matrix.
In fact, these confidences can be interpreted as and mapped into probabilities, by characterizing the probability of a correct prediction given the prediction confidence for each amino acid type.
Like other models, CARBonAra supports autoregressive predictions by using one-hot encoding to imprint previous sequence information for specific amino acids into the backbone atoms.
Most importantly, CARBonAra inherits PeSTo’s ability to use only element names and atomic coordinates without extensive parameterization, making it easy to adapt to various scenarios.
したがって、CARBonAra は、他のタンパク質、小分子、核酸、脂質、イオン、水分子などのさまざまな入力を含む、設計中のタンパク質骨格の近くにあるあらゆる分子エンティティを解析して処理できます。
データセット
CARBonnAra の固有の柔軟性を利用して、研究者は RCSB PDB 内のすべての生物学的アセンブリをトレーニング データセットに組み込むことができます。
これには、イオン、リガンド、核酸などの他の分子実体と複合体を形成したタンパク質が含まれます。トレーニング データセットは約 370,000 のサブユニットで構成され、さらに 100,000 のサブユニットが検証データセットで使用されました。これらはすべて RCSB PDB 生物学的アセンブリから派生し、可能な限り最善の注釈が付けられました。
以前に確立された方法と比較してわずかに厳格なプロトコルに従って、テストデータセットは約70,000のサブユニットで構成され、共有CATHドメインを持たないトレーニングセットとは異なり、30%未満の配列同一性フィルターで実行されました。
この選択基準は、トレーニング データセットに存在する同様のフォールドとシーケンスを除外するため、テストの堅牢性を保証します。
非タンパク質分子を含まない骨格構造からタンパク質またはタンパク質複合体を分離する配列設計では、CARBonAra は ProteinMPNN や ESM-IF1 などの最先端の配列予測法と同等の性能を発揮します。 Competitive の計算コスト (GPU 上の ProteinMPNN より約 3 倍、ESM-IF1 より 10 倍高速)。
主鎖構造からタンパク質配列を再構築する
この方法で主鎖構造からタンパク質配列を再構築する場合、タンパク質モノマー設計の中央値配列回収率は51.3%、ダイマー設計の中央値配列回収率は51.3%です。 56.0%。同様の回収率にもかかわらず、3 つの方法の最良の配列間の配列同一性の中央値は 54% から 58% まで変化しました。
さらに、研究者らは、CARBonnAra が、単一配列モードで AlphaFold を使用して予測した場合、0.9 を超える TM スコアで予想どおりにフォールディングされる高品質な配列を生成できることを観察しました。
CARBonAra は、タンパク質コアでのより緊密なアミノ酸パッキングを学習し、その結果、回収率が高くなり、典型的な埋もれたアミノ酸置換に対する耐性の低下を反映すると同時に、追加の機能的または構造的制約が提供されない限り、タンパク質表面でのより高い変動を許容します。
バックボーン足場からの配列予測の方法は、主に理想的なバックボーン形状の実験データに基づいてトレーニングされるため、生成されたバックボーンに適用するとパフォーマンスが低下します。トレーニング中にジオメトリにノイズを追加すると、この問題を軽減できます。
研究者らは、CARBonnAra を分子動力学 (MD) シミュレーションからの構造軌道に適用することで、この方法の堅牢性を特徴付けました。配列回収率 (53 ± 10%) は、バックボーンの構造変化と、以前に低い回収率を示した症例の増加により、コンセンサス予測 (54 ± 7%) から大幅に減少しませんでした。
同時に、研究者らは、位置ごとに予測される可能性のあるアミノ酸の数が全体的に減少していることを観察し、立体構造空間の探索が配列空間を制約し、標的構造立体構造の設計を可能にすることを示唆しています。
タンパク質の設計自体を超えた影響がある
研究者らは、CARBonAra が酵素工学の課題にどのように対処するかを実証するために、主力システムである TEM-1 セリン ベータ ラクタマーゼで実験を実施し、実際のタンパク質を設計しました。高温で折りたたまれ、触媒活性を保持します。さらに、研究者らは、CARBonnAra の出力からタンパク質配列空間をサンプリングするための焦点を絞った戦略を検討しました。
最高スコアのアミノ酸から得られるタンパク質配列が機能的であるかどうかを判断できないため、in vitroで組換え発現できる安定した機能的なタンパク質を生成するにはサンプリング戦略が必要です。
これはこれまでのところ徹底的にテストされていません。研究チームはここで、適切なサンプリング戦略により、機能性タンパク質を生成するための豊富な情報を生成できるだけでなく、天然配列で観察された、または突然変異誘発と選択の比較を通じて実験的にサンプリングされた自然の変異を反映する合成多重配列も生成できることを示しました。
これはタンパク質の設計自体を超えた意味を持ち、特に生物物理学的に一貫したタンパク質進化モデルの枠組み内でタンパク質がどのように進化するかについての扉を開きます。
基本的に、コンピューテーショナルデザインの成功率を向上させることは、この分野を真にマスターするために重要であり、より現実的には、研究室で実際の発現と精製を試みる際のコストを削減するために重要です。
超高い成功率
AI ベースの手法が安定し始めている現在、これは重要な議論のポイントになります。さまざまな方法やレポートでは成功率が大きく異なりますが、各方法をどのように評価するかが不明瞭なことがよくあります。
Chroma は非常に保守的な評価を行っており、その成功率を約 3% に設定していますが、RoseTTAFold/ProteinMPNN の論文はいくつかのタンパク質の平均成功率を 15% と報告しています。
この研究で報告された TEM-1 ベータラクタマーゼ設計を使用すると、成功率は 40% に達しました。同様に、TIM バレルと NTF2 の折り畳み成功率は 40 ~ 55% と高く、以前の平均である 15% を大きく上回っています。
タンパク質の熱安定性の向上に適しています
新しいタンパク質の設計やタンパク質の機能調整における直接的な応用に加えて、CARBonAra は熱安定性の向上にも適しているようです。他のタンパク質設計方法も堅牢で熱安定性の高いものを生成するためです。タンパク質。
この観察によって明らかになった興味深い側面は、製造および産業プロセスを安定化するために使用される酵素の設計された配列の知的財産権に関連しています。通常、設計された酵素は、より狭い範囲ではあるがかなり広い範囲の配列類似性をカバーする方法で保護されます。 。
歴史的には、これは十分に包括的でしたが、CARBonnAra を含む最新のタンパク質設計手法では、機能を保持し、安定性の高い類似性の低いタンパク質を設計できます。
結論
今後を見据えると、CARBonAra には他のタンパク質設計手法と比較していくつかの利点があり、主に要素名と座標のみに基づく内部動作に関連しており、さらなるパラメーター化や中間計算は必要ありません。
したがって、CARBonAra は他の選択肢よりも柔軟性があるように見えます。基本的にあらゆる種類の分子システムを解決できるため、他の種類の生体分子 (核酸、小分子、イオン、さらには水など) や生物学的集合体に存在しない分子 (材料や表面など) についてもトレーニングできます。十分なデータがあることが前提となります。
要約すると、CARBonAra は構造データに基づいた独自の手法であり、タンパク質配列の予測と設計に対する概念的に異なるアプローチであり、分子設計と合成生物学における将来の課題に対処するために必要なさらなる柔軟性を備えています。
論文リンク:https://www.nature.com/articles/s41467-024-50571-y
The above is the detailed content of Nature sub-journal, 10 times faster, reverse protein sequence design method based on Transformer. For more information, please follow other related articles on the PHP Chinese website!

Hot AI Tools

Undresser.AI Undress
AI-powered app for creating realistic nude photos

AI Clothes Remover
Online AI tool for removing clothes from photos.

Undress AI Tool
Undress images for free

Clothoff.io
AI clothes remover

Video Face Swap
Swap faces in any video effortlessly with our completely free AI face swap tool!

Hot Article

Hot Tools

Notepad++7.3.1
Easy-to-use and free code editor

SublimeText3 Chinese version
Chinese version, very easy to use

Zend Studio 13.0.1
Powerful PHP integrated development environment

Dreamweaver CS6
Visual web development tools

SublimeText3 Mac version
God-level code editing software (SublimeText3)

Hot Topics










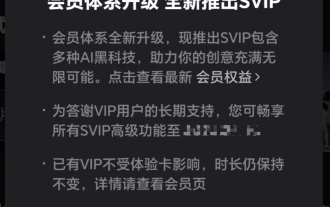
This site reported on June 27 that Jianying is a video editing software developed by FaceMeng Technology, a subsidiary of ByteDance. It relies on the Douyin platform and basically produces short video content for users of the platform. It is compatible with iOS, Android, and Windows. , MacOS and other operating systems. Jianying officially announced the upgrade of its membership system and launched a new SVIP, which includes a variety of AI black technologies, such as intelligent translation, intelligent highlighting, intelligent packaging, digital human synthesis, etc. In terms of price, the monthly fee for clipping SVIP is 79 yuan, the annual fee is 599 yuan (note on this site: equivalent to 49.9 yuan per month), the continuous monthly subscription is 59 yuan per month, and the continuous annual subscription is 499 yuan per year (equivalent to 41.6 yuan per month) . In addition, the cut official also stated that in order to improve the user experience, those who have subscribed to the original VIP
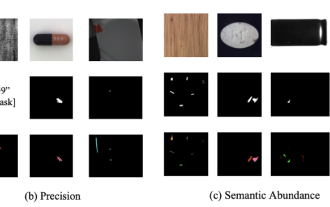
In modern manufacturing, accurate defect detection is not only the key to ensuring product quality, but also the core of improving production efficiency. However, existing defect detection datasets often lack the accuracy and semantic richness required for practical applications, resulting in models unable to identify specific defect categories or locations. In order to solve this problem, a top research team composed of Hong Kong University of Science and Technology Guangzhou and Simou Technology innovatively developed the "DefectSpectrum" data set, which provides detailed and semantically rich large-scale annotation of industrial defects. As shown in Table 1, compared with other industrial data sets, the "DefectSpectrum" data set provides the most defect annotations (5438 defect samples) and the most detailed defect classification (125 defect categories
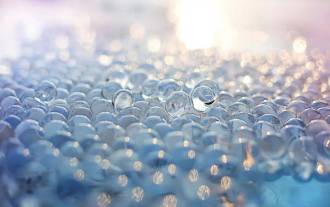
Editor |KX To this day, the structural detail and precision determined by crystallography, from simple metals to large membrane proteins, are unmatched by any other method. However, the biggest challenge, the so-called phase problem, remains retrieving phase information from experimentally determined amplitudes. Researchers at the University of Copenhagen in Denmark have developed a deep learning method called PhAI to solve crystal phase problems. A deep learning neural network trained using millions of artificial crystal structures and their corresponding synthetic diffraction data can generate accurate electron density maps. The study shows that this deep learning-based ab initio structural solution method can solve the phase problem at a resolution of only 2 Angstroms, which is equivalent to only 10% to 20% of the data available at atomic resolution, while traditional ab initio Calculation
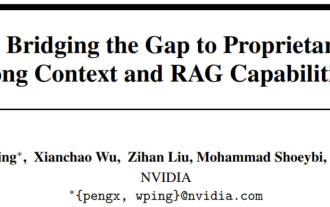
The open LLM community is an era when a hundred flowers bloom and compete. You can see Llama-3-70B-Instruct, QWen2-72B-Instruct, Nemotron-4-340B-Instruct, Mixtral-8x22BInstruct-v0.1 and many other excellent performers. Model. However, compared with proprietary large models represented by GPT-4-Turbo, open models still have significant gaps in many fields. In addition to general models, some open models that specialize in key areas have been developed, such as DeepSeek-Coder-V2 for programming and mathematics, and InternVL for visual-language tasks.
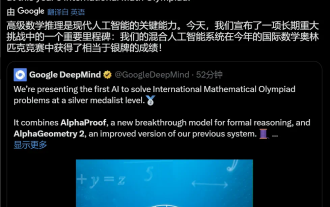
For AI, Mathematical Olympiad is no longer a problem. On Thursday, Google DeepMind's artificial intelligence completed a feat: using AI to solve the real question of this year's International Mathematical Olympiad IMO, and it was just one step away from winning the gold medal. The IMO competition that just ended last week had six questions involving algebra, combinatorics, geometry and number theory. The hybrid AI system proposed by Google got four questions right and scored 28 points, reaching the silver medal level. Earlier this month, UCLA tenured professor Terence Tao had just promoted the AI Mathematical Olympiad (AIMO Progress Award) with a million-dollar prize. Unexpectedly, the level of AI problem solving had improved to this level before July. Do the questions simultaneously on IMO. The most difficult thing to do correctly is IMO, which has the longest history, the largest scale, and the most negative
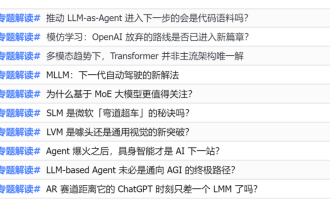
In 2023, almost every field of AI is evolving at an unprecedented speed. At the same time, AI is constantly pushing the technological boundaries of key tracks such as embodied intelligence and autonomous driving. Under the multi-modal trend, will the situation of Transformer as the mainstream architecture of AI large models be shaken? Why has exploring large models based on MoE (Mixed of Experts) architecture become a new trend in the industry? Can Large Vision Models (LVM) become a new breakthrough in general vision? ...From the 2023 PRO member newsletter of this site released in the past six months, we have selected 10 special interpretations that provide in-depth analysis of technological trends and industrial changes in the above fields to help you achieve your goals in the new year. be prepared. This interpretation comes from Week50 2023

Editor | Radish Skin Since the release of the powerful AlphaFold2 in 2021, scientists have been using protein structure prediction models to map various protein structures within cells, discover drugs, and draw a "cosmic map" of every known protein interaction. . Just now, Google DeepMind released the AlphaFold3 model, which can perform joint structure predictions for complexes including proteins, nucleic acids, small molecules, ions and modified residues. The accuracy of AlphaFold3 has been significantly improved compared to many dedicated tools in the past (protein-ligand interaction, protein-nucleic acid interaction, antibody-antigen prediction). This shows that within a single unified deep learning framework, it is possible to achieve
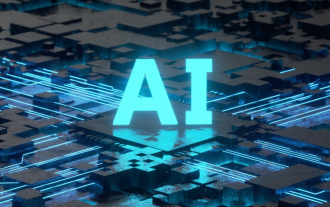
Editor |ScienceAI Question Answering (QA) data set plays a vital role in promoting natural language processing (NLP) research. High-quality QA data sets can not only be used to fine-tune models, but also effectively evaluate the capabilities of large language models (LLM), especially the ability to understand and reason about scientific knowledge. Although there are currently many scientific QA data sets covering medicine, chemistry, biology and other fields, these data sets still have some shortcomings. First, the data form is relatively simple, most of which are multiple-choice questions. They are easy to evaluate, but limit the model's answer selection range and cannot fully test the model's ability to answer scientific questions. In contrast, open-ended Q&A
