


ECCV2024 | Harvard team develops FairDomain to achieve fairness in cross-domain medical image segmentation and classification
Editor | ScienceAI
In the field of artificial intelligence (AI), especially medical AI, addressing fairness issues is crucial to ensuring fair medical outcomes.
Recently, efforts to enhance fairness have introduced new methods and datasets. However, the issue of fairness has been little explored in the context of domain transfer, even though clinics often rely on different imaging technologies (e.g., different retinal imaging modalities) for patient diagnosis.
This paper proposes FairDomain, which is the first systematic study of the fairness of algorithms under domain transfer. We test the state-of-the-art Domain Adaptation (DA) and Domain Generalization (DG) algorithms for medical image segmentation and classification tasks. , designed to understand how biases are transferred between different domains.
We also propose a new plug-and-play Fair Identity Attention (FIA) module to improve the fairness of various DA and DG algorithms by using a self-attention mechanism to adjust feature importance based on demographic attributes sex.
In addition, we have organized and published the first domain-shift dataset focusing on fairness, which contains medical segmentation and classification tasks of two paired imaging modalities of the same patient population to rigorously evaluate domain-shift scenarios. fairness. Excluding the confounding effects of differences in population distribution between source and target domains will allow for clearer quantification of domain transfer model performance.
Our extensive evaluation shows that the proposed FIA significantly enhances model fairness and performance under different demographics in all domain transfer tasks (i.e., DA and DG), outperforming state-of-the-art in both segmentation and classification tasks. There is a way.
Share the work of the ECCV 2024 final draft here "FairDomain: Achieving Fairness in Cross-Domain Medical Image Segmentation and Classification"
Article address: https://arxiv.org/abs/2407.08813
Code address: https://github.com/Harvard-Ophthalmology-AI-Lab/FairDomain
Dataset website: https://ophai.hms.harvard.edu/datasets/harvard-fairdomain20k
Dataset download link: https://drive.google.com/drive/folders/1huH93JVeXMj9rK6p1OZRub868vv0UK0O?usp=sharing
Harvard-Ophthalmology-AI-Lab is committed to providing high-quality fairness data sets and more fairness data sets Please click on the lab’s dataset homepage: https://ophai.hms.harvard.edu/datasets/
Background
In recent years, the advancement of deep learning in the field of medical imaging has greatly improved classification and segmentation tasks Effect. These technologies help improve diagnostic accuracy, simplify treatment planning, and ultimately improve patient health. However, an important challenge when deploying deep learning models in different medical settings is the algorithm's inherent bias and discrimination against specific demographic groups, which may undermine the fairness of medical diagnosis and treatment.
Some recent research has begun to solve the problem of algorithm bias in medical imaging and developed some methods to enhance the fairness of deep learning models. However, these methods usually assume that the data distribution remains unchanged during training and testing phases, which is often not true in actual medical scenarios.
For example, different primary care clinics and specialty hospitals may rely on different imaging technologies (e.g., different retinal imaging modalities) for diagnosis, resulting in significant domain shifts, which in turn affects model performance and fairness.
Therefore, in actual deployment, domain transfer must be considered and models that can maintain fairness in cross-domain scenarios must be learned.
Although domain adaptation and domain generalization have been extensively explored in the literature, these studies have mainly focused on improving model accuracy, ignoring the criticality of ensuring that models provide fair predictions across different population groups. Especially in the medical field, decision-making models directly affect human health and safety, so studying cross-domain fairness is of great significance.
However, there are currently only a few studies that have begun to explore the issue of cross-domain fairness, and these studies lack systematic and comprehensive investigation and usually only focus on domain adaptation or generalization, but rarely both at the same time. In addition, existing research mainly solves medical classification problems, while ignoring the equally important medical segmentation task under domain transfer.
To solve these problems, we introduce FairDomain, which is the first study in the field of medical imaging to systematically explore algorithm fairness under domain transfer.
We conduct extensive experiments with multiple state-of-the-art domain adaptation and generalization algorithms to evaluate their accuracy and fairness under different demographic attributes and understand how fairness transfers across different domains.
Our observations reveal that group performance differences between source and target domains are significantly exacerbated in different medical classification and segmentation tasks. This demonstrates the need to design fairness-oriented algorithms to effectively address this pressing issue.
To compensate for the shortcomings of existing bias mitigation efforts, we introduce a new versatile Fair Identity Attention (FIA) mechanism designed to be seamlessly integrated into various domain adaptation and generalization strategies , feature importance is coordinated with self-attention derived from demographic attributes (e.g., racial group) to promote fairness.
A key challenge in the development of the FairDomain benchmark is the lack of a medical imaging dataset that truly reflects domain shifts in real-world medical domains, which are often caused by different imaging technologies.
In existing medical datasets, differences in patient demographics between source and target domains introduce confounds, making it difficult to distinguish whether observed algorithmic biases are due to demographic distribution changes or inherent domain shifts.
To address this issue, we curated a unique dataset consisting of paired retinal fundus images of the same patient cohort, using two different imaging modalities (En face and SLO fundus images), specifically for analyzing domain transfer scenarios algorithmic bias.
Summary of our contributions:
2. Fair identity attention technology is introduced to improve accuracy and fairness in domain adaptation and generalization.
3. Created a large-scale paired medical segmentation and classification dataset for fairness research, specifically studying fairness issues under domain transfer.
Data Collection and Quality Control
Subjects were selected from a large academic eye hospital at Harvard Medical School between 2010 and 2021. Two cross-domain tasks are explored in this study, namely medical segmentation and medical classification tasks. For the medical segmentation task, the data includes the following five types:
2. SLO fundus image scan;
3. Patient demographic information;
4. Glaucoma diagnosis;
5. Cup and plate mask annotation.
Specifically, the pixel annotation of the cup-disc area is first acquired through the OCT device, and the OCT manufacturer's software segments the disc edge in 3D OCT into Bruch's membrane openings, and detects the cup edge as the internal limiting membrane (ILM) intersecting the plane The intersection point of minimum surface area.
Due to the high contrast of Bruch’s membrane openings and internal limiting membranes with the background, these boundaries can be easily segmented. Because the OCT manufacturer's software utilizes 3D information, cup-disc segmentation is generally reliable.
Considering the limited availability and high cost of OCT equipment in primary care, we propose a method to transfer 3D OCT annotations to 2D SLO fundus images to improve the efficiency of early glaucoma screening.
We use the NiftyReg tool to precisely align SLO fundus images with OCT-derived pixel annotations, generating a large number of high-quality SLO fundus mask annotations.
This process has been validated by a team of medical experts with an 80% registration success rate, streamlining the annotation process for wider use in primary care settings. We leverage these aligned and manually inspected annotations, combined with SLO and En face fundus images, to study the algorithmic fairness of segmentation models under domain shifting.
For the medical classification task, the data includes the following four types:
2. SLO fundus image scan;
3. Patient demographic information;
4. Glaucoma diagnosis.
Subjects in the medical classification data set are divided into two categories: normal and glaucoma based on visual field test results.
Data Features
The medical segmentation dataset contains 10,000 samples from 10,000 subjects. We divide the data into a training set of 8000 samples and a test set of 2000 samples. The mean age of the patients was 60.3 ± 16.5 years.
The dataset contains six demographic attributes including age, gender, race, ethnicity, preferred language, and marital status. The demographic distribution is as follows:
Race: 9.2% Asian, 14.7% black, 76.1% white;
Ethnicity: 90.6% non-Hispanic , Hispanic accounted for 3.7%, unknown accounted for 5.7%;
Preferred language: English accounted for 92.4%, Spanish accounted for 1.5%, other languages accounted for 1%, unknown accounted for 5.1%;
Marital status: married or partnered 57.7%, single 27.1%, divorced 6.8%, legally separated 0.8%, widowed 5.2%, unknown 2.4%.
Similarly, the medical classification dataset contains 10,000 samples of 10,000 subjects with a mean age of 60.9 ± 16.1 years. We divide the data into a training set of 8000 samples and a test set of 2000 samples. The demographic distribution is as follows:
Race: 8.7% Asian, 14.5% black, 76.8% white;
Ethnicity: 96.0% non-Hispanic , Hispanics account for 4.0%;
Preferred language: 92.6% English, 1.7% Spanish, 3.6% other languages, 2.1% unknown;
Marital status: 58.5% married or partnered, 26.1% single, 6.9% divorced, Legal separation accounted for 0.8%, widowed accounted for 1.9%, and unknown accounted for 5.8%.
These detailed demographic information provide a rich data foundation for in-depth research on fairness in cross-domain tasks.
Methods used to improve the fairness of cross-domain AI models Fair Identity Attention (FIA)
Problem definition
Domain Adaptation (DA) and Domain Generalization (DG) It is a key technique in the development of machine learning models and is designed to cope with the variability that may arise when a model is applied from one specific domain to another.
In the field of medical imaging, DA and DG techniques are critical for creating models that can robustly handle variability between different medical institutions, imaging devices, and patient populations. This paper aims to explore fairness dynamics in the context of domain transfer and develop methods to ensure that models remain fair and reliable when adapting or generalizing to new domains.
We aim to develop a method function f that mitigates the common fairness degradation when a model is transferred from the source domain to the target domain. Such exacerbations are primarily due to the potential for domain shifts to amplify existing biases in the dataset, particularly those related to demographic attributes such as gender, race, or ethnicity.
To address this problem, we propose an attention mechanism-based approach that aims to identify and exploit image features relevant to downstream tasks such as segmentation and classification while taking into account demographic attributes.
Figure 3 shows the architecture of the proposed fair identity attention module. This module first obtains the input image embedding E_i and attribute embedding E_a by processing the input image and input statistical attribute labels. These embeddings are then added to position embedding E_p. The detailed calculation formula is as follows:
By calculating the dot product of query and key, we extract the similarity matrix related to the current feature attribute. The dot product of this matrix and value is then used to extract features that are significant in downstream tasks for each feature attribute. This process is represented by the following formula:
where D is a scaling factor to avoid excessively large values in the softmax function.
Subsequently, a residual connection adds E_i to the output of attention to maintain the integrity of the input information. Finally, a normalization layer and a multi-layer perceptron (MLP) layer further extract features. After another residual operation on the outputs of these two layers, we get the final output of the fair attention module.
The Fair Identity Attention Mechanism is a powerful and versatile tool designed to improve model performance while solving fairness issues. By explicitly accounting for demographic attributes such as gender, race, or ethnicity, it ensures that learned representations do not inadvertently amplify biases present in the data.
Its architecture allows it to be seamlessly integrated into any existing network as a plug-in component. This modular nature enables researchers and practitioners to integrate fair identity attention into their models without requiring extensive modifications to the underlying architecture.
Therefore, the fair identity attention module not only helps improve model accuracy and fairness in segmentation and classification tasks, but also promotes the implementation of trustworthy AI by promoting fair treatment of different groups in the data set.
Experiments
Algorithmic fairness in domain transfer
In our experiments, we first analyze fairness in the context of domain transfer, specifically focusing on the cup and plate segmentation task. Cup-to-disc segmentation refers to the process of accurately delineating the optic cup and disc in fundus images, which is critical for calculating the cup-to-disc ratio (CDR), a key parameter in assessing glaucoma progression and risk.
This task is particularly important in the field of medical imaging, especially when diagnosing and managing eye diseases such as glaucoma. Since the optic cup is an important subregion of the optic disc, we reframe the segmentation task as cup and rim (the tissue region between the optic cup and optic disc rim) segmentation to avoid errors due to the large overlap between the optic cup and the optic disc. Resulting in performance distortion.
We studied fairness performance across three different demographics (gender, race and ethnicity) in two different areas: En face fundus images acquired from optical coherence tomography (OCT) and Scanning laser fundus image (SLO).
In subsequent experiments, we selected the En face fundus image as the source domain and the SLO fundus image as the target domain. The reason for this is that compared to SLO fundus images, En face fundus images are more common in specialist eye care settings and therefore the availability of the data is significantly higher.
したがって、ソース ドメインとして En face 眼底画像を使用し、ターゲット ドメインとして SLO 眼底画像を使用することを選択します。分類タスクでは、これら 2 つのドメインの眼底画像をソース ドメインとターゲット ドメインとして使用し、正常と緑内障の 2 つのカテゴリに分類します。
評価メトリクス
Dice メトリクスと IoU メトリクスを使用してセグメンテーションのパフォーマンスを評価し、AUC を使用して分類タスクのパフォーマンスを評価します。これらの従来のセグメンテーションおよび分類メトリクスは、モデルのパフォーマンスを反映するものではありますが、本質的には人口統計グループ間の公平性を考慮していません。
医療画像におけるモデルのパフォーマンスと公平性の間の潜在的なトレードオフに対処するために、新しい ESP (Equity Scaled Performance) メトリクスを使用して、セグメンテーションおよび分類タスクのパフォーマンスと公平性を評価します。
{Dice,IoU, AUC, .}M∈{Dice,IoU,AUC,...} の ∈{Dice,IoU,AUC,...}M をセグメンテーションまたは分類インデックスに適した一般的なパフォーマンスとします。 。従来の評価では、人口統計上のアイデンティティ属性が無視されることが多く、そのため重要な公平性評価が欠落しています。公平性を組み込むために、まずパフォーマンスの差 Δ を計算します。これは、全体的なパフォーマンスからの各人口統計グループの指標の集合的な偏差として定義され、次のように定式化されます:
グループ間のパフォーマンスの公平性が達成されると、Δ はゼロに近づきます。 、最小の差を反映します。次に、ESP メトリクスは次のように定式化できます:
この統一メトリクスは、深層学習モデルを包括的に評価するのに役立ち、その精度 (Dice、IoU、AUC などの測定によるなど) だけでなく、また、人口グループ間の異なる公平性におけるパフォーマンスについても調査しました。
ドメインシフトでのカップリムセグメンテーション結果
ドメインシフトでの緑内障分類結果
概要
この記事は人工知能(特に医療)に焦点を当てていますAI における公平性の問題)は、公平な医療を実現するための鍵となります。
クリニックは異なる画像技術を使用している可能性があるため、ドメイン転送の公平性の問題はほとんど解明されていないままです。私たちの研究では、医療のセグメント化と分類という 2 つの一般的なタスクを含む、ドメインの適応と一般化を含む、ドメイン転送タスクにおけるアルゴリズムの公平性に関する包括的な研究である FairDomain を紹介しています。
私たちは、ドメイン転送タスクの公平性を強化するためのアテンション メカニズムを通じて、人口統計的属性に基づく特徴の相関関係を学習するための、新しいプラグ アンド プレイの Fair Identity Attendance (FIA) モジュールを提案します。
また、モデルの公平性に対する人口統計分布の変化の交絡的な影響を排除するために、同じ患者コホートの 2 つのペアのイメージング画像を含む、公平性に焦点を当てた最初のクロスドメイン データセットも作成しました。これにより、モデルの公平性に対するドメイン移転の影響を正確に評価できるようになります。
私たちの公平なアイデンティティ アテンション モデルは、既存のドメイン適応および一般化手法を改善し、公平性を念頭に置いてモデルのパフォーマンスを向上させることができます。
注: カバー画像は AI によって生成されます。
The above is the detailed content of ECCV2024 | Harvard team develops FairDomain to achieve fairness in cross-domain medical image segmentation and classification. For more information, please follow other related articles on the PHP Chinese website!

Hot AI Tools

Undresser.AI Undress
AI-powered app for creating realistic nude photos

AI Clothes Remover
Online AI tool for removing clothes from photos.

Undress AI Tool
Undress images for free

Clothoff.io
AI clothes remover

Video Face Swap
Swap faces in any video effortlessly with our completely free AI face swap tool!

Hot Article

Hot Tools

Notepad++7.3.1
Easy-to-use and free code editor

SublimeText3 Chinese version
Chinese version, very easy to use

Zend Studio 13.0.1
Powerful PHP integrated development environment

Dreamweaver CS6
Visual web development tools

SublimeText3 Mac version
God-level code editing software (SublimeText3)

Hot Topics










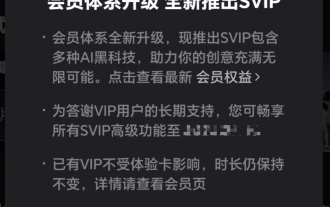
This site reported on June 27 that Jianying is a video editing software developed by FaceMeng Technology, a subsidiary of ByteDance. It relies on the Douyin platform and basically produces short video content for users of the platform. It is compatible with iOS, Android, and Windows. , MacOS and other operating systems. Jianying officially announced the upgrade of its membership system and launched a new SVIP, which includes a variety of AI black technologies, such as intelligent translation, intelligent highlighting, intelligent packaging, digital human synthesis, etc. In terms of price, the monthly fee for clipping SVIP is 79 yuan, the annual fee is 599 yuan (note on this site: equivalent to 49.9 yuan per month), the continuous monthly subscription is 59 yuan per month, and the continuous annual subscription is 499 yuan per year (equivalent to 41.6 yuan per month) . In addition, the cut official also stated that in order to improve the user experience, those who have subscribed to the original VIP
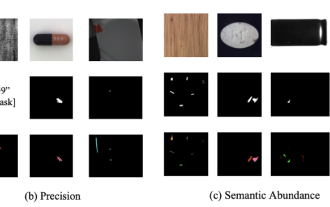
In modern manufacturing, accurate defect detection is not only the key to ensuring product quality, but also the core of improving production efficiency. However, existing defect detection datasets often lack the accuracy and semantic richness required for practical applications, resulting in models unable to identify specific defect categories or locations. In order to solve this problem, a top research team composed of Hong Kong University of Science and Technology Guangzhou and Simou Technology innovatively developed the "DefectSpectrum" data set, which provides detailed and semantically rich large-scale annotation of industrial defects. As shown in Table 1, compared with other industrial data sets, the "DefectSpectrum" data set provides the most defect annotations (5438 defect samples) and the most detailed defect classification (125 defect categories
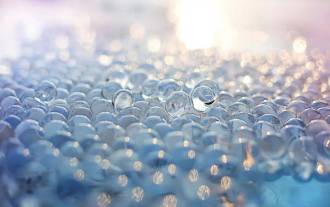
Editor |KX To this day, the structural detail and precision determined by crystallography, from simple metals to large membrane proteins, are unmatched by any other method. However, the biggest challenge, the so-called phase problem, remains retrieving phase information from experimentally determined amplitudes. Researchers at the University of Copenhagen in Denmark have developed a deep learning method called PhAI to solve crystal phase problems. A deep learning neural network trained using millions of artificial crystal structures and their corresponding synthetic diffraction data can generate accurate electron density maps. The study shows that this deep learning-based ab initio structural solution method can solve the phase problem at a resolution of only 2 Angstroms, which is equivalent to only 10% to 20% of the data available at atomic resolution, while traditional ab initio Calculation
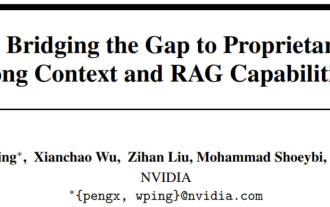
The open LLM community is an era when a hundred flowers bloom and compete. You can see Llama-3-70B-Instruct, QWen2-72B-Instruct, Nemotron-4-340B-Instruct, Mixtral-8x22BInstruct-v0.1 and many other excellent performers. Model. However, compared with proprietary large models represented by GPT-4-Turbo, open models still have significant gaps in many fields. In addition to general models, some open models that specialize in key areas have been developed, such as DeepSeek-Coder-V2 for programming and mathematics, and InternVL for visual-language tasks.
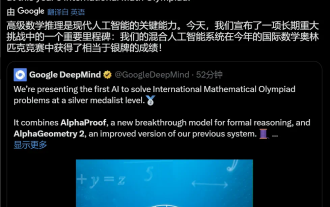
For AI, Mathematical Olympiad is no longer a problem. On Thursday, Google DeepMind's artificial intelligence completed a feat: using AI to solve the real question of this year's International Mathematical Olympiad IMO, and it was just one step away from winning the gold medal. The IMO competition that just ended last week had six questions involving algebra, combinatorics, geometry and number theory. The hybrid AI system proposed by Google got four questions right and scored 28 points, reaching the silver medal level. Earlier this month, UCLA tenured professor Terence Tao had just promoted the AI Mathematical Olympiad (AIMO Progress Award) with a million-dollar prize. Unexpectedly, the level of AI problem solving had improved to this level before July. Do the questions simultaneously on IMO. The most difficult thing to do correctly is IMO, which has the longest history, the largest scale, and the most negative
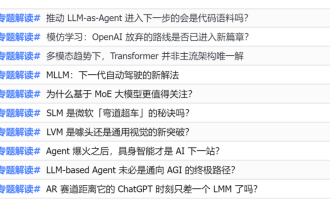
In 2023, almost every field of AI is evolving at an unprecedented speed. At the same time, AI is constantly pushing the technological boundaries of key tracks such as embodied intelligence and autonomous driving. Under the multi-modal trend, will the situation of Transformer as the mainstream architecture of AI large models be shaken? Why has exploring large models based on MoE (Mixed of Experts) architecture become a new trend in the industry? Can Large Vision Models (LVM) become a new breakthrough in general vision? ...From the 2023 PRO member newsletter of this site released in the past six months, we have selected 10 special interpretations that provide in-depth analysis of technological trends and industrial changes in the above fields to help you achieve your goals in the new year. be prepared. This interpretation comes from Week50 2023
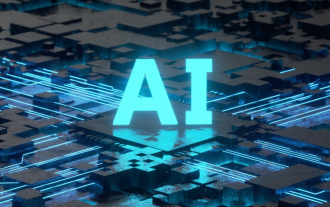
Editor |ScienceAI Question Answering (QA) data set plays a vital role in promoting natural language processing (NLP) research. High-quality QA data sets can not only be used to fine-tune models, but also effectively evaluate the capabilities of large language models (LLM), especially the ability to understand and reason about scientific knowledge. Although there are currently many scientific QA data sets covering medicine, chemistry, biology and other fields, these data sets still have some shortcomings. First, the data form is relatively simple, most of which are multiple-choice questions. They are easy to evaluate, but limit the model's answer selection range and cannot fully test the model's ability to answer scientific questions. In contrast, open-ended Q&A

Editor | KX Retrosynthesis is a critical task in drug discovery and organic synthesis, and AI is increasingly used to speed up the process. Existing AI methods have unsatisfactory performance and limited diversity. In practice, chemical reactions often cause local molecular changes, with considerable overlap between reactants and products. Inspired by this, Hou Tingjun's team at Zhejiang University proposed to redefine single-step retrosynthetic prediction as a molecular string editing task, iteratively refining the target molecular string to generate precursor compounds. And an editing-based retrosynthetic model EditRetro is proposed, which can achieve high-quality and diverse predictions. Extensive experiments show that the model achieves excellent performance on the standard benchmark data set USPTO-50 K, with a top-1 accuracy of 60.8%.
