


TPAMI 2024 | ProCo: Long-tail contrastive learning of infinite contrastive pairs

The AIxiv column is a column where this site publishes academic and technical content. In the past few years, the AIxiv column of this site has received more than 2,000 reports, covering top laboratories from major universities and companies around the world, effectively promoting academic exchanges and dissemination. If you have excellent work that you want to share, please feel free to contribute or contact us for reporting. Submission email: liyazhou@jiqizhixin.com; zhaoyunfeng@jiqizhixin.com
Du Chaoqun, the first author of this paper, is a 2020 direct PhD student in the Department of Automation, Tsinghua University. The tutor is Associate Professor Huang Gao. He previously received a Bachelor of Science degree from the Department of Physics of Tsinghua University. His research interests include model generalization and robustness research on different data distributions, such as long-tail learning, semi-supervised learning, transfer learning, etc. Published many papers in first-class international journals and conferences such as TPAMI and ICML.
Personal homepage: https://andy-du20.github.io
This article introduces a paper on long-tail visual recognition from Tsinghua University: Probabilistic Contrastive Learning for Long-Tailed Visual Recognition. This work has been TPAMI 2024 accepted, the code has been open source.
This research mainly focuses on the application of contrastive learning in long-tail visual recognition tasks. It proposes a new long-tail contrastive learning method ProCo. By improving the contrastive loss, it achieves contrastive learning of an unlimited number of contrastive pairs, effectively solving the problem Supervised contrastive learning[1] has an inherent dependence on batch (memory bank) size. In addition to long-tail visual classification tasks, this method was also experimented on long-tail semi-supervised learning, long-tail object detection, and balanced datasets, achieving significant performance improvements.
- Paper link: https://arxiv.org/pdf/2403.06726
- Project link: https://github.com/LeapLabTHU/ProCo
Comparison The success of learning in self-supervised learning demonstrates its effectiveness in learning visual feature representations. The core factor affecting contrastive learning performance is the number of
contrastive pairs, which enables the model to learn from more negative samples, which is reflected in the two most representative methods SimCLR [2] and MoCo [3] respectively. batch size and memory bank size. However, in long-tail visual recognition tasks, due to category imbalance, the gain brought by increasing the number of contrastive pairs will produce a serious marginal diminishing effect. This is because most of the contrastive pairs are composed of head categories. Composed of samples, it is difficult to cover the tail categories. For example, in the long-tail Imagenet data set, if the batch size (memory bank) is set to the common 4096 and 8192, then there are an average of 212
and89 categories in each batch (memory bank) respectively. The sample size is less than one. Therefore, the core idea of the ProCo method is: on the long-tail data set, by modeling the distribution of each type of data, estimating parameters and sampling from it to build contrasting pairs, ensuring that all categories can be covered. Furthermore, when the number of samples tends to infinity, the expected analytical solution of contrastive loss can be strictly derived theoretically, so that it can be directly used as the optimization target to avoid inefficient sampling of contrastive pairs and achieve an infinite number of contrastive pairs. Comparative learning.
However, there are several main difficulties in realizing the above ideas: How to model the distribution of each type of data.
- How to efficiently estimate the parameters of a distribution, especially for tail categories with a small number of samples.
- How to ensure that the expected analytical solution of contrastive loss exists and can be calculated.
- In fact, the above problems can be solved by a unified probability model, that is, a simple and effective probability distribution is selected to model the characteristic distribution, so that maximum likelihood estimation can be used to efficiently estimate the parameters of the distribution and calculate Expect an analytical solution to contrastive loss.
Since the features of contrastive learning are distributed on the unit hypersphere, a feasible solution is to select the von Mises-Fisher (vMF) distribution on the sphere as the feature distribution (this distribution is similar to the normal distribution on the sphere) . The maximum likelihood estimation of vMF distribution parameters has an approximate analytical solution and only relies on the first-order moment statistics of the feature. Therefore, the parameters of the distribution can be estimated efficiently and the expectation of contrastive loss can be strictly derived, thereby achieving the comparison of an unlimited number of contrastive pairs. study.
Figure 1 L'algorithme ProCo estime la distribution des échantillons en fonction des caractéristiques des différents lots. En échantillonnant un nombre illimité d'échantillons, la solution analytique de la perte contrastive attendue peut être obtenue, éliminant ainsi la dépendance inhérente de l'apprentissage contrastif supervisé à l'égard du taille du lot (banque de mémoire).
Détails de la méthode
Ce qui suit présentera la méthode ProCo en détail sous quatre aspects : hypothèse de distribution, estimation des paramètres, objectifs d'optimisation et analyse théorique.
Hypothèse de distribution
Comme mentionné précédemment, les fonctionnalités de l'apprentissage contrastif sont limitées à l'hypersphère unitaire. Par conséquent, on peut supposer que la distribution à laquelle obéissent ces caractéristiques est la distribution de von Mises-Fisher (vMF) et que sa fonction de densité de probabilité est :
où z est le vecteur unitaire des caractéristiques à p dimensions, I est le vecteur unitaire modifié. Fonction de Bessel du premier type,
μ est la direction moyenne de la distribution, κ est le paramètre de concentration, qui contrôle le degré de concentration de la distribution. Lorsque κ est plus grand, le degré de regroupement des échantillons est proche de la moyenne. est plus élevé lorsque κ = 0, la distribution vMF dégénère en une distribution uniforme.
Estimation des paramètres
Sur la base de l'hypothèse de distribution ci-dessus, la distribution globale des caractéristiques des données est une distribution vMF mixte, où chaque catégorie correspond à une distribution vMF.
où le paramètre représente la probabilité a priori de chaque catégorie, correspondant à la fréquence de la catégorie y dans l'ensemble d'entraînement. Le vecteur moyen
et le paramètre groupé
de la distribution des caractéristiques sont estimés par estimation du maximum de vraisemblance.
En supposant que N vecteurs unitaires indépendants sont échantillonnés à partir de la distribution vMF de catégorie y, l'estimation du maximum de vraisemblance (environ) [4] des paramètres de direction moyenne et de concentration satisfait l'équation suivante :
où est l'échantillon moyenne,
est la longueur du module de la moyenne de l'échantillon. De plus, afin d'utiliser des échantillons historiques, ProCo adopte une méthode d'estimation en ligne, qui peut estimer efficacement les paramètres de la catégorie de queue.
Objectif d'optimisation
Sur la base des paramètres estimés, une approche simple consiste à échantillonner à partir de la distribution vMF mixte pour construire des paires contrastives. Cependant, l'échantillonnage d'un grand nombre d'échantillons de la distribution vMF à chaque itération d'entraînement est inefficace. Par conséquent, cette étude étend théoriquement le nombre d’échantillons à l’infini et dérive strictement la solution analytique de la fonction de perte de contraste attendue directement comme cible d’optimisation.
En introduisant une branche de fonctionnalités supplémentaire (apprentissage des représentations basé sur cet objectif d'optimisation) pendant le processus de formation, cette branche peut être entraînée avec la branche de classification et n'augmentera pas puisque seule la branche de classification est nécessaire lors de l'inférence Calcul supplémentaire coût. La somme pondérée des pertes des deux branches est utilisée comme objectif d'optimisation final, et α=1 est défini dans l'expérience. Enfin, le processus global de l'algorithme ProCo est le suivant : Analyse théorique
Afin d'aller plus loin. analyser la Pour vérifier théoriquement l'efficacité de la méthode ProCo, les chercheurs ont analysé sa limite d'erreur de généralisation et sa limite d'excès de risque. Pour simplifier l'analyse, on suppose ici qu'il n'y a que deux catégories, à savoir y∈{-1,+1}. L'analyse montre que la limite d'erreur de généralisation est principalement contrôlée par le nombre d'échantillons d'apprentissage et la variance des données. L'analyse théorique des travaux connexes [6] [7] est cohérente, garantissant que la perte ProCo n'introduit pas de facteurs supplémentaires et n'augmente pas la limite d'erreur de généralisation, ce qui garantit théoriquement l'efficacité de cette méthode.
De plus, cette méthode repose sur certaines hypothèses concernant les distributions de caractéristiques et les estimations de paramètres. Pour évaluer l'impact de ces paramètres sur les performances du modèle, les chercheurs ont également analysé la limite de risque excédentaire de perte ProCo, qui mesure l'écart entre le risque attendu à l'aide des paramètres estimés et le risque optimal de Bayes, qui se situe dans la vraie distribution du risque attendu. paramètres.
Cela montre que le risque excédentaire de perte de ProCo est principalement contrôlé par le terme de premier ordre de l'erreur d'estimation du paramètre.
Résultats expérimentaux
Pour vérifier la motivation fondamentale, les chercheurs ont d'abord comparé les performances de différentes méthodes d'apprentissage contrastées sous différentes tailles de lots. Baseline inclut Balanced Contrastive Learning [5] (BCL), une méthode améliorée également basée sur SCL sur les tâches de reconnaissance à longue traîne. Le cadre expérimental spécifique suit la stratégie de formation en deux étapes de l'apprentissage contrastif supervisé (SCL), c'est-à-dire qu'il faut d'abord utiliser uniquement la perte contrastive pour la formation à l'apprentissage des représentations, puis entraîner un classificateur linéaire pour les tests avec le squelette gelé.
La figure ci-dessous montre les résultats expérimentaux sur l'ensemble de données CIFAR100-LT (IF100). Les performances de BCL et SupCon sont évidemment limitées par la taille du lot, mais ProCo élimine efficacement l'impact de SupCon sur la taille du lot en introduisant la fonctionnalité. distribution de chaque dépendance de catégorie, obtenant ainsi les meilleures performances sous différentes tailles de lots.
De plus, les chercheurs ont également mené des expériences sur des tâches de reconnaissance à longue traîne, l'apprentissage semi-supervisé à longue traîne, la détection d'objets à longue traîne et des ensembles de données équilibrés. Ici, nous montrons principalement les résultats expérimentaux sur les ensembles de données à grande échelle Imagenet-LT et iNaturalist2018. Premièrement, dans le cadre d'un programme de formation de 90 époques, par rapport à des méthodes similaires d'amélioration de l'apprentissage contrasté, ProCo présente une amélioration des performances d'au moins 1 % sur deux ensembles de données et deux piliers.
Les résultats suivants montrent en outre que ProCo peut également bénéficier d'un programme d'entraînement plus long Dans le cadre du programme de 400 époques, ProCo a atteint les performances SOTA sur l'ensemble de données iNaturalist2018 et a également vérifié qu'il peut rivaliser avec d'autres combinaisons non A. de méthodes d'apprentissage contrastées, y compris la distillation (NCL) et d'autres méthodes. "Un cadre simple pour l'apprentissage contrastif des représentations visuelles." Conférence internationale sur l'apprentissage automatique, 2020.
- S Sra, « Une brève note sur l'approximation des paramètres pour les distributions de von mises-fisher : et une implémentation rapide de is (x) », Computational Statistics, 2012.
-
- W. Jitkrittum, et al. « ELM : intégration et marges logit pour l'apprentissage à longue queue », Préimpression arXiv, 2022.
- A K. Menon, et al . « Apprentissage longue traîne via l'ajustement logit », dans ICLR, 2021.
.
The above is the detailed content of TPAMI 2024 | ProCo: Long-tail contrastive learning of infinite contrastive pairs. For more information, please follow other related articles on the PHP Chinese website!

Hot AI Tools

Undresser.AI Undress
AI-powered app for creating realistic nude photos

AI Clothes Remover
Online AI tool for removing clothes from photos.

Undress AI Tool
Undress images for free

Clothoff.io
AI clothes remover

Video Face Swap
Swap faces in any video effortlessly with our completely free AI face swap tool!

Hot Article

Hot Tools

Notepad++7.3.1
Easy-to-use and free code editor

SublimeText3 Chinese version
Chinese version, very easy to use

Zend Studio 13.0.1
Powerful PHP integrated development environment

Dreamweaver CS6
Visual web development tools

SublimeText3 Mac version
God-level code editing software (SublimeText3)

Hot Topics










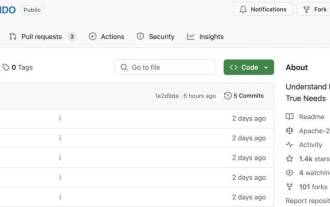
It is also a Tusheng video, but PaintsUndo has taken a different route. ControlNet author LvminZhang started to live again! This time I aim at the field of painting. The new project PaintsUndo has received 1.4kstar (still rising crazily) not long after it was launched. Project address: https://github.com/lllyasviel/Paints-UNDO Through this project, the user inputs a static image, and PaintsUndo can automatically help you generate a video of the entire painting process, from line draft to finished product. follow. During the drawing process, the line changes are amazing. The final video result is very similar to the original image: Let’s take a look at a complete drawing.

The AIxiv column is a column where this site publishes academic and technical content. In the past few years, the AIxiv column of this site has received more than 2,000 reports, covering top laboratories from major universities and companies around the world, effectively promoting academic exchanges and dissemination. If you have excellent work that you want to share, please feel free to contribute or contact us for reporting. Submission email: liyazhou@jiqizhixin.com; zhaoyunfeng@jiqizhixin.com The authors of this paper are all from the team of teacher Zhang Lingming at the University of Illinois at Urbana-Champaign (UIUC), including: Steven Code repair; Deng Yinlin, fourth-year doctoral student, researcher

The AIxiv column is a column where this site publishes academic and technical content. In the past few years, the AIxiv column of this site has received more than 2,000 reports, covering top laboratories from major universities and companies around the world, effectively promoting academic exchanges and dissemination. If you have excellent work that you want to share, please feel free to contribute or contact us for reporting. Submission email: liyazhou@jiqizhixin.com; zhaoyunfeng@jiqizhixin.com In the development process of artificial intelligence, the control and guidance of large language models (LLM) has always been one of the core challenges, aiming to ensure that these models are both powerful and safe serve human society. Early efforts focused on reinforcement learning methods through human feedback (RL
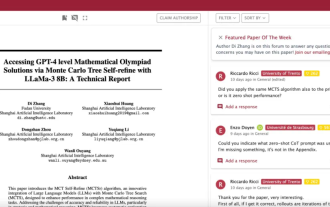
cheers! What is it like when a paper discussion is down to words? Recently, students at Stanford University created alphaXiv, an open discussion forum for arXiv papers that allows questions and comments to be posted directly on any arXiv paper. Website link: https://alphaxiv.org/ In fact, there is no need to visit this website specifically. Just change arXiv in any URL to alphaXiv to directly open the corresponding paper on the alphaXiv forum: you can accurately locate the paragraphs in the paper, Sentence: In the discussion area on the right, users can post questions to ask the author about the ideas and details of the paper. For example, they can also comment on the content of the paper, such as: "Given to
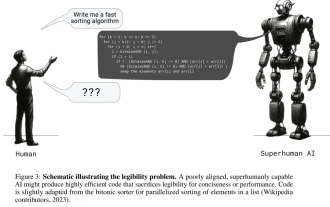
If the answer given by the AI model is incomprehensible at all, would you dare to use it? As machine learning systems are used in more important areas, it becomes increasingly important to demonstrate why we can trust their output, and when not to trust them. One possible way to gain trust in the output of a complex system is to require the system to produce an interpretation of its output that is readable to a human or another trusted system, that is, fully understandable to the point that any possible errors can be found. For example, to build trust in the judicial system, we require courts to provide clear and readable written opinions that explain and support their decisions. For large language models, we can also adopt a similar approach. However, when taking this approach, ensure that the language model generates

Recently, the Riemann Hypothesis, known as one of the seven major problems of the millennium, has achieved a new breakthrough. The Riemann Hypothesis is a very important unsolved problem in mathematics, related to the precise properties of the distribution of prime numbers (primes are those numbers that are only divisible by 1 and themselves, and they play a fundamental role in number theory). In today's mathematical literature, there are more than a thousand mathematical propositions based on the establishment of the Riemann Hypothesis (or its generalized form). In other words, once the Riemann Hypothesis and its generalized form are proven, these more than a thousand propositions will be established as theorems, which will have a profound impact on the field of mathematics; and if the Riemann Hypothesis is proven wrong, then among these propositions part of it will also lose its effectiveness. New breakthrough comes from MIT mathematics professor Larry Guth and Oxford University
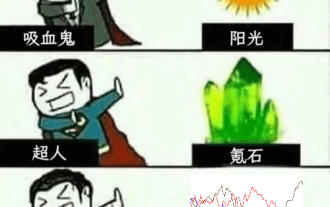
Can language models really be used for time series prediction? According to Betteridge's Law of Headlines (any news headline ending with a question mark can be answered with "no"), the answer should be no. The fact seems to be true: such a powerful LLM cannot handle time series data well. Time series, that is, time series, as the name suggests, refers to a set of data point sequences arranged in the order of time. Time series analysis is critical in many areas, including disease spread prediction, retail analytics, healthcare, and finance. In the field of time series analysis, many researchers have recently been studying how to use large language models (LLM) to classify, predict, and detect anomalies in time series. These papers assume that language models that are good at handling sequential dependencies in text can also generalize to time series.

The AIxiv column is a column where this site publishes academic and technical content. In the past few years, the AIxiv column of this site has received more than 2,000 reports, covering top laboratories from major universities and companies around the world, effectively promoting academic exchanges and dissemination. If you have excellent work that you want to share, please feel free to contribute or contact us for reporting. Submission email: liyazhou@jiqizhixin.com; zhaoyunfeng@jiqizhixin.com. Introduction In recent years, the application of multimodal large language models (MLLM) in various fields has achieved remarkable success. However, as the basic model for many downstream tasks, current MLLM consists of the well-known Transformer network, which
