


From the college entrance examination to the Olympic arena: the ultimate battle between large models and human intelligence

AIxiv专栏是本站发布学术、技术内容的栏目。过去数年,本站AIxiv专栏接收报道了2000多篇内容,覆盖全球各大高校与企业的顶级实验室,有效促进了学术交流与传播。如果您有优秀的工作想要分享,欢迎投稿或者联系报道。投稿邮箱:liyazhou@jiqizhixin.com;zhaoyunfeng@jiqizhixin.com
上海交通大学生成式人工智能实验室(GAIR Lab)的研究团队,主要研究方向是:大模型训练、对齐与评估。团队主页:https://plms.ai/
在未来20年内,AI有望超过人类的智能水平。图灵奖得主Hinton在他的访谈中提及“在未来20年内,AI有望超越人类的智能水平”,并建议各大科技公司早做准备,评定大模型(包括多模态大模型)的“智力水平”则是这一准备的必要前提。
一个具有跨学科问题集、可以从多维度严谨评估AI的认知推理能力评估基准已经变得相当亟需。
论文地址:https://arxiv.org/pdf/2406.12753 项目地址:https://gair-nlp.github.io/OlympicArena/ 代码地址:https://github.com/GAIR-NLP/OlympicArena
OlympicArena的特点概述了它对多模态支持、多种认知能力考察以及细粒度评估(既考虑对错的评估,又考虑每个推理步骤评估)的例子。
Comprehensive: OlympicArena includes a total of 11,163 questions from 62 different Olympic competitions, spanning seven core subjects: mathematics, physics, chemistry, biology, geography, astronomy and computer, involving 34 professional branches. At the same time, unlike previous benchmarks that mostly focused on objective questions such as multiple-choice questions, OlympicArena supports a variety of question types, including expressions, equations, intervals, writing chemical equations and even programming questions. In addition, OlympicArena supports multi-modality (nearly half of the questions contain pictures), and adopts the most realistic text-image input format (interleaved text-image), fully testing the use of visual information to assist large models in solving problems. The ability to reason. Extremely challenging: Unlike previous benchmarks that either focused on high school (college entrance exam) questions or college questions, OlympicArena focuses more on the pure examination of complex reasoning abilities rather than on the massive knowledge of large models. Points of memory, recall ability or simple application ability. Therefore, all questions in OlympicArena are of Olympiad difficulty level. Moreover, in order to fine-grainedly evaluate the performance of large models in different types of reasoning capabilities, the research team also summarized 8 types of logical reasoning capabilities and 5 types of visual reasoning capabilities. Subsequently, they specifically analyzed the performance of existing large models in different types of reasoning capabilities. Differences in performance on reasoning abilities. Rigor: Guiding the healthy development of large models is the role that academia should play. Currently, in public benchmarks, many popular large models will have data leakage problems (that is, the test data of the benchmark is leaked in the large model) in the training data). Therefore, the research team specifically tested the data leakage of OlympicArena on some popular large models to more rigorously verify the effectiveness of the benchmark. Fine-grained evaluation: Previous benchmarks often only evaluate whether the final answer given by a large model is consistent with the correct answer. This is one-sided in the evaluation of very complex reasoning problems and cannot well reflect the current model. More realistic reasoning skills. Therefore, in addition to evaluating the answers, the research team also included an evaluation of the correctness of the question process (steps). At the same time, the research team also analyzed different results from multiple different dimensions, such as analyzing the performance differences of models in different disciplines, different modalities, and different reasoning capabilities.
The accuracy of different models in different subjects of OlympicArena. The CS programming questions use the unbiased pass@k index, and the rest use the accuracy index.
The performance of each model in logical reasoning and visual reasoning abilities. Logical reasoning abilities include: deductive reasoning (DED), inductive reasoning (IND), abductive reasoning (ABD), analogical reasoning (ANA), causal reasoning (CAE), critical thinking (CT), decomposition reasoning (DEC) and quantitative Reasoning (QUA). Visual reasoning abilities include: pattern recognition (PR), spatial reasoning (SPA), diagrammatic reasoning (DIA), symbolic interpretation (SYB), and visual comparison (COM).
Comparison of different multimodal models (LMMs) and their corresponding text-only models (LLMs) in three different experimental settings.
When text and images are input together, LMMs may pay more attention to the text and ignore the information in the image. Some LMMs may lose some of their inherent language capabilities (e.g., reasoning capabilities) when training visual capabilities based on their text models, which is especially obvious in the complex scenarios of this project. This benchmark question uses a complex text-image wrapping input format. Some models cannot support this format well, resulting in their inability to process and understand image position information embedded in text.
Left picture: The correlation between the correctness of the answers and the correctness of the process for all models in all questions where the inference process is evaluated. Right: Distribution of locations of erroneous process steps.
As shown in (b) above, step-level evaluation There is usually a high degree of agreement between the results of and assessments that rely solely on answers. When a model generates correct answers, the quality of its inference process is mostly higher. The accuracy of the reasoning process is usually higher than the accuracy of just looking at the answers. This shows that even for very complex problems, the model can correctly perform some intermediate steps. Therefore, models may have significant potential in cognitive reasoning, which opens up new research directions for researchers. The research team also found that in some disciplines, some models that performed well when evaluated solely on answers performed poorly on the inference process. The research team speculates that this is because models sometimes ignore the plausibility of intermediate steps when generating answers, even though these steps may not be critical to the final result. In addition, the research team conducted a statistical analysis of the location distribution of error steps (see Figure c) and found that a higher proportion of errors occurred in the later reasoning steps of a question. This shows that as the reasoning process accumulates, the model is more prone to errors and produces an accumulation of errors, which shows that the model still has a lot of room for improvement when dealing with long-chain logical reasoning.
An example of GPT-4V making mistakes on a Mathematical Olympiad question
The number of leaked samples detected and the corresponding plain text and multi-modal models on these sample questions Make the right amount.
Vision: A glorious moment of joint progress between humans and AI
The above is the detailed content of From the college entrance examination to the Olympic arena: the ultimate battle between large models and human intelligence. For more information, please follow other related articles on the PHP Chinese website!

Hot AI Tools

Undresser.AI Undress
AI-powered app for creating realistic nude photos

AI Clothes Remover
Online AI tool for removing clothes from photos.

Undress AI Tool
Undress images for free

Clothoff.io
AI clothes remover

Video Face Swap
Swap faces in any video effortlessly with our completely free AI face swap tool!

Hot Article

Hot Tools

Notepad++7.3.1
Easy-to-use and free code editor

SublimeText3 Chinese version
Chinese version, very easy to use

Zend Studio 13.0.1
Powerful PHP integrated development environment

Dreamweaver CS6
Visual web development tools

SublimeText3 Mac version
God-level code editing software (SublimeText3)

Hot Topics










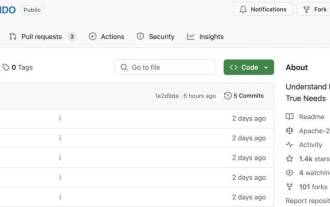
It is also a Tusheng video, but PaintsUndo has taken a different route. ControlNet author LvminZhang started to live again! This time I aim at the field of painting. The new project PaintsUndo has received 1.4kstar (still rising crazily) not long after it was launched. Project address: https://github.com/lllyasviel/Paints-UNDO Through this project, the user inputs a static image, and PaintsUndo can automatically help you generate a video of the entire painting process, from line draft to finished product. follow. During the drawing process, the line changes are amazing. The final video result is very similar to the original image: Let’s take a look at a complete drawing.

The AIxiv column is a column where this site publishes academic and technical content. In the past few years, the AIxiv column of this site has received more than 2,000 reports, covering top laboratories from major universities and companies around the world, effectively promoting academic exchanges and dissemination. If you have excellent work that you want to share, please feel free to contribute or contact us for reporting. Submission email: liyazhou@jiqizhixin.com; zhaoyunfeng@jiqizhixin.com The authors of this paper are all from the team of teacher Zhang Lingming at the University of Illinois at Urbana-Champaign (UIUC), including: Steven Code repair; Deng Yinlin, fourth-year doctoral student, researcher

The AIxiv column is a column where this site publishes academic and technical content. In the past few years, the AIxiv column of this site has received more than 2,000 reports, covering top laboratories from major universities and companies around the world, effectively promoting academic exchanges and dissemination. If you have excellent work that you want to share, please feel free to contribute or contact us for reporting. Submission email: liyazhou@jiqizhixin.com; zhaoyunfeng@jiqizhixin.com In the development process of artificial intelligence, the control and guidance of large language models (LLM) has always been one of the core challenges, aiming to ensure that these models are both powerful and safe serve human society. Early efforts focused on reinforcement learning methods through human feedback (RL
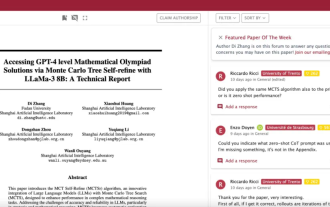
cheers! What is it like when a paper discussion is down to words? Recently, students at Stanford University created alphaXiv, an open discussion forum for arXiv papers that allows questions and comments to be posted directly on any arXiv paper. Website link: https://alphaxiv.org/ In fact, there is no need to visit this website specifically. Just change arXiv in any URL to alphaXiv to directly open the corresponding paper on the alphaXiv forum: you can accurately locate the paragraphs in the paper, Sentence: In the discussion area on the right, users can post questions to ask the author about the ideas and details of the paper. For example, they can also comment on the content of the paper, such as: "Given to
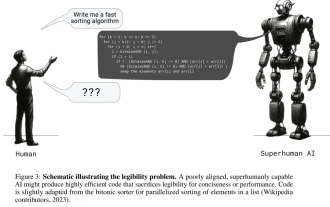
If the answer given by the AI model is incomprehensible at all, would you dare to use it? As machine learning systems are used in more important areas, it becomes increasingly important to demonstrate why we can trust their output, and when not to trust them. One possible way to gain trust in the output of a complex system is to require the system to produce an interpretation of its output that is readable to a human or another trusted system, that is, fully understandable to the point that any possible errors can be found. For example, to build trust in the judicial system, we require courts to provide clear and readable written opinions that explain and support their decisions. For large language models, we can also adopt a similar approach. However, when taking this approach, ensure that the language model generates

Recently, the Riemann Hypothesis, known as one of the seven major problems of the millennium, has achieved a new breakthrough. The Riemann Hypothesis is a very important unsolved problem in mathematics, related to the precise properties of the distribution of prime numbers (primes are those numbers that are only divisible by 1 and themselves, and they play a fundamental role in number theory). In today's mathematical literature, there are more than a thousand mathematical propositions based on the establishment of the Riemann Hypothesis (or its generalized form). In other words, once the Riemann Hypothesis and its generalized form are proven, these more than a thousand propositions will be established as theorems, which will have a profound impact on the field of mathematics; and if the Riemann Hypothesis is proven wrong, then among these propositions part of it will also lose its effectiveness. New breakthrough comes from MIT mathematics professor Larry Guth and Oxford University
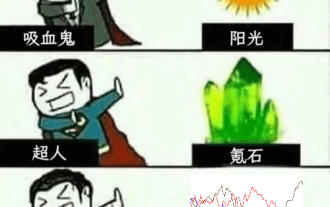
Can language models really be used for time series prediction? According to Betteridge's Law of Headlines (any news headline ending with a question mark can be answered with "no"), the answer should be no. The fact seems to be true: such a powerful LLM cannot handle time series data well. Time series, that is, time series, as the name suggests, refers to a set of data point sequences arranged in the order of time. Time series analysis is critical in many areas, including disease spread prediction, retail analytics, healthcare, and finance. In the field of time series analysis, many researchers have recently been studying how to use large language models (LLM) to classify, predict, and detect anomalies in time series. These papers assume that language models that are good at handling sequential dependencies in text can also generalize to time series.

The AIxiv column is a column where this site publishes academic and technical content. In the past few years, the AIxiv column of this site has received more than 2,000 reports, covering top laboratories from major universities and companies around the world, effectively promoting academic exchanges and dissemination. If you have excellent work that you want to share, please feel free to contribute or contact us for reporting. Submission email: liyazhou@jiqizhixin.com; zhaoyunfeng@jiqizhixin.com. Introduction In recent years, the application of multimodal large language models (MLLM) in various fields has achieved remarkable success. However, as the basic model for many downstream tasks, current MLLM consists of the well-known Transformer network, which
