


Smart Building: Building Exterior Wall Defect Detection Based on YOLOv7
01 Outlook Summary
Current deep learning-based methods face some challenges in terms of recognition speed and model complexity. In order to ensure the accuracy and speed of building exterior wall defect detection, we studied an improved YOLOv7 method named BFD-YOLO. First, the original ELAN module in YOLOv7 is replaced by the lightweight MobileOne module to reduce the number of parameters and increase the inference speed. Secondly, a coordinate attention module is added to the model to enhance feature extraction capabilities. Next, SCYLLA-IoU is used to speed up detection and increase the recall of the model. Finally, we extended the open dataset and constructed a building facade damage dataset including three typical defects. BFD-YOLO demonstrates excellent accuracy and efficiency based on this dataset. Compared with YOLOv7, the accuracy and mAP@0.5 of BFD-YOLO are improved by 2.2% and 2.9% respectively, while maintaining considerable efficiency. Experiments show that this method achieves high detection accuracy while ensuring real-time performance.
02 Current Situation and Project Motivation
The presence of facade defects is a pressing issue during the operational phase of construction and is often attributed to mechanical and environmental factor. Typical defects include concrete spalling, decorative spalling, component cracks, large-scale deformation, tile damage, moisture damage, etc. These defects can affect the appearance and reduce the service life of the building. As an integral part of structural damage detection, building exterior wall defect detection can enable governments and management to accurately understand the comprehensive condition of building exterior walls, thereby helping to formulate reasonable repair plans. This is crucial to reducing building maintenance costs and extending the service life of the building. As an integral part of structural damage detection, building exterior wall defect detection can enable governments and management to accurately understand the comprehensive condition of building exterior walls, thereby helping to formulate reasonable repair plans. This is crucial to reducing building maintenance costs and extending the service life of the building. Many countries and regions are developing policies for periodic standardized visual inspections. Detection of defects in building exterior walls has become an important part of building maintenance, which helps to accurately understand the comprehensive condition of building exterior walls, thereby helping to formulate reasonable repair plans. This is an important way to reduce building maintenance costs and extend the service life of the building. Many countries and regions are developing policies for periodic standardized visual inspections. Detection of defects in building exterior walls has become an important part of building maintenance, which helps to accurately understand the comprehensive condition of building exterior walls, thereby helping to formulate reasonable repair plans. This is an important way to reduce building maintenance costs and extend the service life of the building. Detection of defects in building exterior walls has become an important part of building maintenance, which helps to accurately understand the comprehensive condition of building exterior walls, thereby helping to formulate reasonable repair plans. This is an important way to reduce building maintenance costs and extend the service life of the building.
Visual inspection is a simple and reliable way to assess the condition of a building’s exterior. Traditional building exterior inspections typically require professionals to arrive at the inspection site armed with specialized tools, where they are evaluated using visual inspection, hammering and other techniques. These methods rely on the expertise and experience of the inspector, which is subjective, dangerous and inefficient. Due to the increase in the number and scale of buildings, manual visual inspection methods are no longer sufficient to meet the requirements for large-scale inspections. As technology advances, many new methods (such as laser scanning, 3D thermal imaging and SLAM) are being used for exterior wall damage detection via drones and robotic platforms. These new methods are more convenient and safer than traditional techniques, but are time-consuming and costly. Therefore, these methods also face challenges in meeting the needs of large-scale inspections. Therefore, it is necessary to develop a more accurate and effective surface defect detection method to improve detection efficiency and reduce computational costs.
03 New ideas and practical details
There are many types of building exterior wall defects, and different detection methods are applicable. Common types include pullout defects, spalling and wall hollows. For cracks, there are more studies using semantic segmentation for detection. For wall hollows, the tapping method and infrared thermal imaging method are more widely used. After research and research, we selected defect types that are suitable for target detection methods and easy to construct data sets. The images in the data set mainly come from building facade images taken by mobile phones, cameras and drones. In addition, some images from the Internet and public datasets are also used for expansion. All images range in width from 1000 pixels to 3000 pixels. The height range is between 2000 pixels and 5000 pixels. The dataset consists of three building facade defects: delamination, spalling, and tile loss. A total of 1907 original images were collected, which contained background images of infrared thermal imaging images. Background images are defect-free images added to the dataset to reduce error locations. The training set, validation set and test set are divided in a ratio of 7:2:1. The image below shows an example of defects in the dataset.
From left to right are delamination, spalling and tile loss.
Data Augmentation
A large amount of data is often required in model training of neural networks. However, it is relatively difficult to obtain images of building facade defects, and there is a class imbalance problem in the collected data. To mitigate the impact of this problem, we apply data augmentation techniques to the training data. Data augmentation is a common technique for performing various transformations on raw data. It is widely used in the field of deep learning to generate more training data. Data augmentation can help the model learn more data variations and prevent it from being overly dependent on specific training samples. Surveillance data enhancement techniques include geometric transformations (e.g., flipping, rotating, scaling, cropping, etc.) and pixel transformations (e.g., noise, blur, brightness adjustment, saturation adjustment, etc.). The goal of data augmentation is to generate more training samples while preventing it from being overly dependent on a specific training sample. This can help the model better respond to changes in data and prevent overfitting. Data augmentation can help the model learn more data variations and prevent it from being overly dependent on specific training samples. For surveillance data enhancement techniques, various transformations (e.g., flipping, rotating, scaling, cropping, etc.) and pixel transformations (e.g., noise, blur, brightness adjustment, saturation adjustment, etc.) can be applied. These techniques can help the model better respond to changes in data and prevent overfitting. To make full use of data augmentation techniques, we can use different data transformations (such as flipping, rotating, scaling, cropping, etc.) and pixel transformations (such as noise, blur, brightness adjustment, saturation adjustment
##New design framework
04 Project implementation effect
An experimental platform is established for training. model and conduct testing. The hardware and software configuration of the experimental platform are as shown in the following table.
System | Win 10 |
|
CPU |
I7- 11700 |
|
GPU |
##RTX 3090 |
|
RAM | ##32GB||
|
||
|
The above is the detailed content of Smart Building: Building Exterior Wall Defect Detection Based on YOLOv7. For more information, please follow other related articles on the PHP Chinese website!

Hot AI Tools

Undresser.AI Undress
AI-powered app for creating realistic nude photos

AI Clothes Remover
Online AI tool for removing clothes from photos.

Undress AI Tool
Undress images for free

Clothoff.io
AI clothes remover

Video Face Swap
Swap faces in any video effortlessly with our completely free AI face swap tool!

Hot Article

Hot Tools

Notepad++7.3.1
Easy-to-use and free code editor

SublimeText3 Chinese version
Chinese version, very easy to use

Zend Studio 13.0.1
Powerful PHP integrated development environment

Dreamweaver CS6
Visual web development tools

SublimeText3 Mac version
God-level code editing software (SublimeText3)

Hot Topics










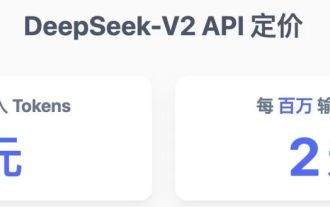
Imagine an artificial intelligence model that not only has the ability to surpass traditional computing, but also achieves more efficient performance at a lower cost. This is not science fiction, DeepSeek-V2[1], the world’s most powerful open source MoE model is here. DeepSeek-V2 is a powerful mixture of experts (MoE) language model with the characteristics of economical training and efficient inference. It consists of 236B parameters, 21B of which are used to activate each marker. Compared with DeepSeek67B, DeepSeek-V2 has stronger performance, while saving 42.5% of training costs, reducing KV cache by 93.3%, and increasing the maximum generation throughput to 5.76 times. DeepSeek is a company exploring general artificial intelligence
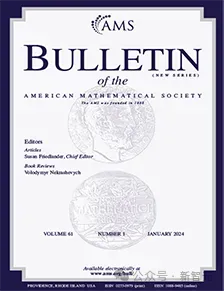
AI is indeed changing mathematics. Recently, Tao Zhexuan, who has been paying close attention to this issue, forwarded the latest issue of "Bulletin of the American Mathematical Society" (Bulletin of the American Mathematical Society). Focusing on the topic "Will machines change mathematics?", many mathematicians expressed their opinions. The whole process was full of sparks, hardcore and exciting. The author has a strong lineup, including Fields Medal winner Akshay Venkatesh, Chinese mathematician Zheng Lejun, NYU computer scientist Ernest Davis and many other well-known scholars in the industry. The world of AI has changed dramatically. You know, many of these articles were submitted a year ago.
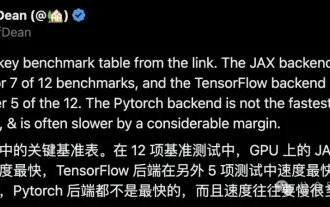
The performance of JAX, promoted by Google, has surpassed that of Pytorch and TensorFlow in recent benchmark tests, ranking first in 7 indicators. And the test was not done on the TPU with the best JAX performance. Although among developers, Pytorch is still more popular than Tensorflow. But in the future, perhaps more large models will be trained and run based on the JAX platform. Models Recently, the Keras team benchmarked three backends (TensorFlow, JAX, PyTorch) with the native PyTorch implementation and Keras2 with TensorFlow. First, they select a set of mainstream
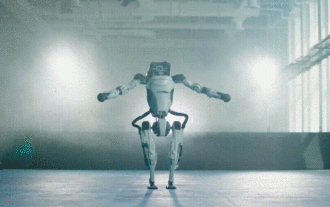
Boston Dynamics Atlas officially enters the era of electric robots! Yesterday, the hydraulic Atlas just "tearfully" withdrew from the stage of history. Today, Boston Dynamics announced that the electric Atlas is on the job. It seems that in the field of commercial humanoid robots, Boston Dynamics is determined to compete with Tesla. After the new video was released, it had already been viewed by more than one million people in just ten hours. The old people leave and new roles appear. This is a historical necessity. There is no doubt that this year is the explosive year of humanoid robots. Netizens commented: The advancement of robots has made this year's opening ceremony look like a human, and the degree of freedom is far greater than that of humans. But is this really not a horror movie? At the beginning of the video, Atlas is lying calmly on the ground, seemingly on his back. What follows is jaw-dropping
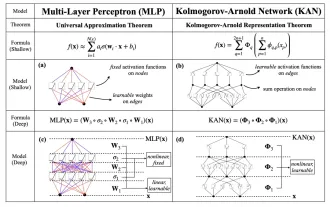
Earlier this month, researchers from MIT and other institutions proposed a very promising alternative to MLP - KAN. KAN outperforms MLP in terms of accuracy and interpretability. And it can outperform MLP running with a larger number of parameters with a very small number of parameters. For example, the authors stated that they used KAN to reproduce DeepMind's results with a smaller network and a higher degree of automation. Specifically, DeepMind's MLP has about 300,000 parameters, while KAN only has about 200 parameters. KAN has a strong mathematical foundation like MLP. MLP is based on the universal approximation theorem, while KAN is based on the Kolmogorov-Arnold representation theorem. As shown in the figure below, KAN has
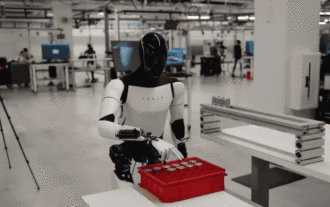
The latest video of Tesla's robot Optimus is released, and it can already work in the factory. At normal speed, it sorts batteries (Tesla's 4680 batteries) like this: The official also released what it looks like at 20x speed - on a small "workstation", picking and picking and picking: This time it is released One of the highlights of the video is that Optimus completes this work in the factory, completely autonomously, without human intervention throughout the process. And from the perspective of Optimus, it can also pick up and place the crooked battery, focusing on automatic error correction: Regarding Optimus's hand, NVIDIA scientist Jim Fan gave a high evaluation: Optimus's hand is the world's five-fingered robot. One of the most dexterous. Its hands are not only tactile
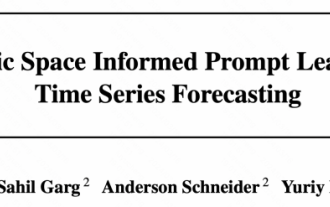
Today I would like to share a recent research work from the University of Connecticut that proposes a method to align time series data with large natural language processing (NLP) models on the latent space to improve the performance of time series forecasting. The key to this method is to use latent spatial hints (prompts) to enhance the accuracy of time series predictions. Paper title: S2IP-LLM: SemanticSpaceInformedPromptLearningwithLLMforTimeSeriesForecasting Download address: https://arxiv.org/pdf/2403.05798v1.pdf 1. Large problem background model
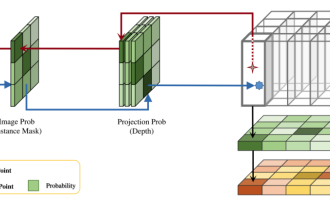
This paper explores the problem of accurately detecting objects from different viewing angles (such as perspective and bird's-eye view) in autonomous driving, especially how to effectively transform features from perspective (PV) to bird's-eye view (BEV) space. Transformation is implemented via the Visual Transformation (VT) module. Existing methods are broadly divided into two strategies: 2D to 3D and 3D to 2D conversion. 2D-to-3D methods improve dense 2D features by predicting depth probabilities, but the inherent uncertainty of depth predictions, especially in distant regions, may introduce inaccuracies. While 3D to 2D methods usually use 3D queries to sample 2D features and learn the attention weights of the correspondence between 3D and 2D features through a Transformer, which increases the computational and deployment time.
