


GPT-4o and SQL: How capable is a large model of changing its own architecture?
Author丨David Eastman
Compiled丨Noah
produced | 51CTO technology stack (WeChat ID: blog51cto)
Although there is no large language model ( LLM) have driven bicycles, but they clearly understand the role of driving behavior in the realm of human transportation. They are similar to what software developers provide as a kind of semantic-like real-world knowledge combined with an understanding of the technical world. We saw this clearly in a recent article where we were able to generate a simple SQL schema for book publishing simply by describing it in natural language.
While I was pleased with the performance of the Llama 3 creation schema, a colleague from my previous days at Oracle pointed out that the book publishing schema was a fairly familiar example. For ease of understanding, this is naturally a good thing, but in order to further expand the capabilities of LLM, in this article I will explore the ability of large language models to adjust their architecture according to problems described in English. This time, I will use OpenAI’s GPT-4o as it has served me well with code reviews recently.
As a starting point, we will start with the same questions as in the first article and summarize the answers. This answer is similar to last time. This time, GPT-4o not only provides us with an ERD (entity relationship diagram), but also explains the relationship between entities very well.
Picture
Similar to the previous attempt, it proposed the following architecture:
CREATE TABLE Author ( author_id INT AUTO_INCREMENT PRIMARY KEY, first_name VARCHAR(50), last_name VARCHAR(50), birth_date DATE, nationality VARCHAR(50) ); CREATE TABLE Publisher ( publisher_id INT AUTO_INCREMENT PRIMARY KEY, name VARCHAR(100), address VARCHAR(255), contact_number VARCHAR(20), email VARCHAR(100) ); CREATE TABLE Book ( book_id INT AUTO_INCREMENT PRIMARY KEY, title VARCHAR(100), genre VARCHAR(50), publication_date DATE, isbn VARCHAR(20) UNIQUE, author_id INT, publisher_id INT, FOREIGN KEY (author_id) REFERENCES Author(author_id), FOREIGN KEY (publisher_id) REFERENCES Publisher(publisher_id) );
I Prefer to have table names use the plural form of the contained objects, which I think is a widely accepted standard.
Large language models point out these relational limitations:
Picture
So, using the same example data from last time, let’s Check whether the same result can be obtained in the SQL sandbox environment DB Fiddle.
If we populate this data and add the last view...
INSERT INTO Author (first_name, last_name, birth_date) VALUES ('Iain', 'Banks', '1954-02-16'); INSERT INTO Author (first_name, last_name, birth_date) VALUES ('Iain', 'M Banks', '1954-02-16'); INSERT INTO Publisher (name, address) VALUES ('Abacus', 'London'); INSERT INTO Publisher (name, address) VALUES ('Orbit', 'New York'); INSERT INTO Book (title, author_id, publisher_id, publication_date)VALUES ('Consider Phlebas', 2, 2, '1988-04-14'); INSERT INTO Book (title, author_id, publisher_id, publication_date)VALUES ('The Wasp Factory', 1, 1, '1984-02-15'); CREATE VIEW ViewableBooks ASSELECT Book.title 'Book', Author.first_name 'Author firstname', Author.last_name 'Author surname', Publisher.name 'Publisher', Book.publication_dateFROM Book, Publisher, AuthorWHERE Book.author_id = Author.author_idAND Book.publisher_id = Publisher.publisher_id;
We can get the desired result view from DB Fiddle in the table below:
Picture
The second surname contains the middle name "M", which looks a bit awkward. Next, we will explore issues related to this.
1. First revision
As I mentioned in my previous article about SQL generation, "Ian Banks" and "Ian M Banks" are actually the same author. Last time, we didn't address this pen name issue. So, let's ask for the big model to fix this:
Picture
So that's a good start. This time it needed to map the literary concept of "pen name" onto the existing architectural design it had produced. So it has to do more than just discover existing solutions. First, let's take a look at the newly established relationship:
Picture
This seems reasonable. The following is the modified new table structure:
CREATE TABLE Pseudonym ( pseudonym_id INT AUTO_INCREMENT PRIMARY KEY, pseudonym VARCHAR(100), author_id INT, FOREIGN KEY (author_id) REFERENCES Author(author_id) ); CREATE TABLE Book ( book_id INT AUTO_INCREMENT PRIMARY KEY, title VARCHAR(100), genre VARCHAR(50), publication_date DATE, isbn VARCHAR(20) UNIQUE, pseudonym_id INT, publisher_id INT, FOREIGN KEY (pseudonym_id) REFERENCES Pseudonym(pseudonym_id), FOREIGN KEY (publisher_id) REFERENCES Publisher(publisher_id) );
This also feels right. The schema now associates books to pen names rather than directly to authors. Let's re-do a dbfiddle with the new schema, input the modified data to work with, and see if we can get the desired results again:
Picture
Actually, the pen name column is just a field now, and the table looks neater.
2. Another Modification Request
Now I will request a further schema modification. We know that a book can have multiple authors (you may remember that Llama 3 proposed this without prompting last time), so we expect GPT-4o to revise its architecture again.
Picture
The new table that needs to be added is:
CREATE TABLE BookAuthor ( book_id INT, pseudonym_id INT, PRIMARY KEY (book_id, pseudonym_id), FOREIGN KEY (book_id) REFERENCES Book(book_id), FOREIGN KEY (pseudonym_id) REFERENCES Pseudonym(pseudonym_id) );
Thus, the relationship changes are as follows:
Picture
(Note that there is a weird bracket error after describing the first few relationships. This The error is repeated in the description of all relationships. It seems to prevent the text "1:M" or "M:M" from being printed - maybe due to emoji confusion)
Of course, GPT- 4o is also following a single conversational thread – it takes its previous work into context. This much-lauded capability does make interacting with it more natural. Overall, it did a good job (and very quickly) of parsing our English descriptions to adapt its suggested schema.
3. Before we get too excited
Architecture is primarily about the relationships between things—it doesn’t require a deep understanding of the things themselves. However, this does not entirely mean that the road is clear for large models to take over database design.
Optimizing SQL queries and schemas has always been a bit of an art. You need to understand which common queries will be best suited for a certain design, how many tables will be involved, dependencies between queries, index definitions, partitioning, and so on. And that's before dealing with the CAP theorem dilemma - the trade-off between consistency and availability. Beneath these technical abstractions are expectations that data retrieval will be far more than simple.
I have no doubt that some combination of large language models and specialization will gradually solve these engineering problems over time, but for now we should be grateful for GPT-4o's ability to efficiently generate and modify reasonable architectures ability to feel victorious.
Reference link: https://thenewstack.io/gpt-4o-and-sql-how-well-can-an-llm-alter-its-own-schema/
To learn more about AIGC, please visit:
https://www.51cto.com/aigc /
The above is the detailed content of GPT-4o and SQL: How capable is a large model of changing its own architecture?. For more information, please follow other related articles on the PHP Chinese website!

Hot AI Tools

Undresser.AI Undress
AI-powered app for creating realistic nude photos

AI Clothes Remover
Online AI tool for removing clothes from photos.

Undress AI Tool
Undress images for free

Clothoff.io
AI clothes remover

Video Face Swap
Swap faces in any video effortlessly with our completely free AI face swap tool!

Hot Article

Hot Tools

Notepad++7.3.1
Easy-to-use and free code editor

SublimeText3 Chinese version
Chinese version, very easy to use

Zend Studio 13.0.1
Powerful PHP integrated development environment

Dreamweaver CS6
Visual web development tools

SublimeText3 Mac version
God-level code editing software (SublimeText3)

Hot Topics










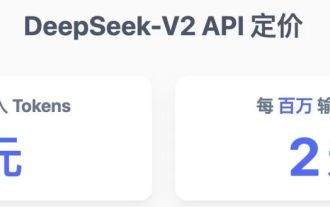
Imagine an artificial intelligence model that not only has the ability to surpass traditional computing, but also achieves more efficient performance at a lower cost. This is not science fiction, DeepSeek-V2[1], the world’s most powerful open source MoE model is here. DeepSeek-V2 is a powerful mixture of experts (MoE) language model with the characteristics of economical training and efficient inference. It consists of 236B parameters, 21B of which are used to activate each marker. Compared with DeepSeek67B, DeepSeek-V2 has stronger performance, while saving 42.5% of training costs, reducing KV cache by 93.3%, and increasing the maximum generation throughput to 5.76 times. DeepSeek is a company exploring general artificial intelligence
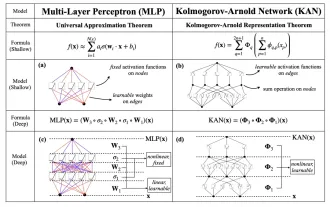
Earlier this month, researchers from MIT and other institutions proposed a very promising alternative to MLP - KAN. KAN outperforms MLP in terms of accuracy and interpretability. And it can outperform MLP running with a larger number of parameters with a very small number of parameters. For example, the authors stated that they used KAN to reproduce DeepMind's results with a smaller network and a higher degree of automation. Specifically, DeepMind's MLP has about 300,000 parameters, while KAN only has about 200 parameters. KAN has a strong mathematical foundation like MLP. MLP is based on the universal approximation theorem, while KAN is based on the Kolmogorov-Arnold representation theorem. As shown in the figure below, KAN has
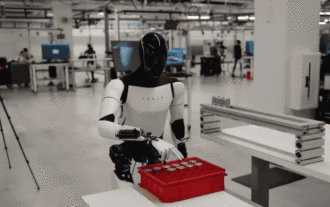
The latest video of Tesla's robot Optimus is released, and it can already work in the factory. At normal speed, it sorts batteries (Tesla's 4680 batteries) like this: The official also released what it looks like at 20x speed - on a small "workstation", picking and picking and picking: This time it is released One of the highlights of the video is that Optimus completes this work in the factory, completely autonomously, without human intervention throughout the process. And from the perspective of Optimus, it can also pick up and place the crooked battery, focusing on automatic error correction: Regarding Optimus's hand, NVIDIA scientist Jim Fan gave a high evaluation: Optimus's hand is the world's five-fingered robot. One of the most dexterous. Its hands are not only tactile
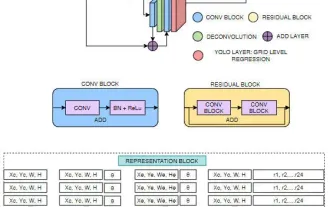
Target detection is a relatively mature problem in autonomous driving systems, among which pedestrian detection is one of the earliest algorithms to be deployed. Very comprehensive research has been carried out in most papers. However, distance perception using fisheye cameras for surround view is relatively less studied. Due to large radial distortion, standard bounding box representation is difficult to implement in fisheye cameras. To alleviate the above description, we explore extended bounding box, ellipse, and general polygon designs into polar/angular representations and define an instance segmentation mIOU metric to analyze these representations. The proposed model fisheyeDetNet with polygonal shape outperforms other models and simultaneously achieves 49.5% mAP on the Valeo fisheye camera dataset for autonomous driving
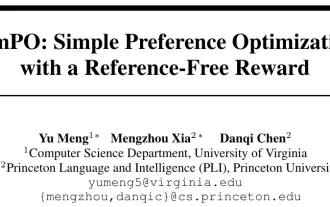
In order to align large language models (LLMs) with human values and intentions, it is critical to learn human feedback to ensure that they are useful, honest, and harmless. In terms of aligning LLM, an effective method is reinforcement learning based on human feedback (RLHF). Although the results of the RLHF method are excellent, there are some optimization challenges involved. This involves training a reward model and then optimizing a policy model to maximize that reward. Recently, some researchers have explored simpler offline algorithms, one of which is direct preference optimization (DPO). DPO learns the policy model directly based on preference data by parameterizing the reward function in RLHF, thus eliminating the need for an explicit reward model. This method is simple and stable
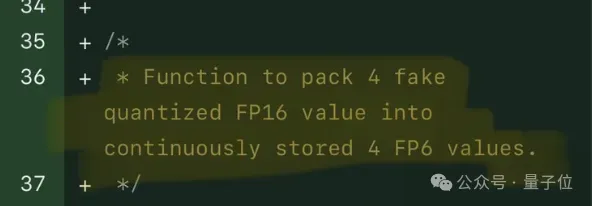
FP8 and lower floating point quantification precision are no longer the "patent" of H100! Lao Huang wanted everyone to use INT8/INT4, and the Microsoft DeepSpeed team started running FP6 on A100 without official support from NVIDIA. Test results show that the new method TC-FPx's FP6 quantization on A100 is close to or occasionally faster than INT4, and has higher accuracy than the latter. On top of this, there is also end-to-end large model support, which has been open sourced and integrated into deep learning inference frameworks such as DeepSpeed. This result also has an immediate effect on accelerating large models - under this framework, using a single card to run Llama, the throughput is 2.65 times higher than that of dual cards. one
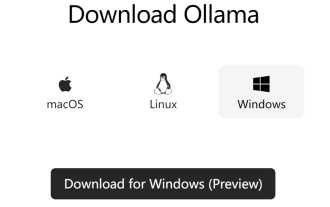
Overview LLaMA-3 (LargeLanguageModelMetaAI3) is a large-scale open source generative artificial intelligence model developed by Meta Company. It has no major changes in model structure compared with the previous generation LLaMA-2. The LLaMA-3 model is divided into different scale versions, including small, medium and large, to suit different application needs and computing resources. The parameter size of small models is 8B, the parameter size of medium models is 70B, and the parameter size of large models reaches 400B. However, during training, the goal is to achieve multi-modal and multi-language functionality, and the results are expected to be comparable to GPT4/GPT4V. Install OllamaOllama is an open source large language model (LL
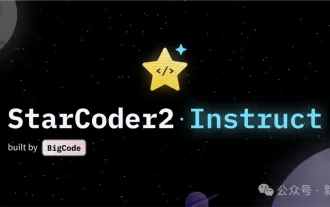
At the forefront of software technology, UIUC Zhang Lingming's group, together with researchers from the BigCode organization, recently announced the StarCoder2-15B-Instruct large code model. This innovative achievement achieved a significant breakthrough in code generation tasks, successfully surpassing CodeLlama-70B-Instruct and reaching the top of the code generation performance list. The unique feature of StarCoder2-15B-Instruct is its pure self-alignment strategy. The entire training process is open, transparent, and completely autonomous and controllable. The model generates thousands of instructions via StarCoder2-15B in response to fine-tuning the StarCoder-15B base model without relying on expensive manual annotation.
