


XJTLU and the University of Liverpool propose: the first comprehensive review of point cloud data enhancement

The AIxiv column is a column where this site publishes academic and technical content. In the past few years, the AIxiv column of this site has received more than 2,000 reports, covering top laboratories from major universities and companies around the world, effectively promoting academic exchanges and dissemination. If you have excellent work that you want to share, please feel free to contribute or contact us for reporting. Submission email: liyazhou@jiqizhixin.com; zhaoyunfeng@jiqizhixin.com
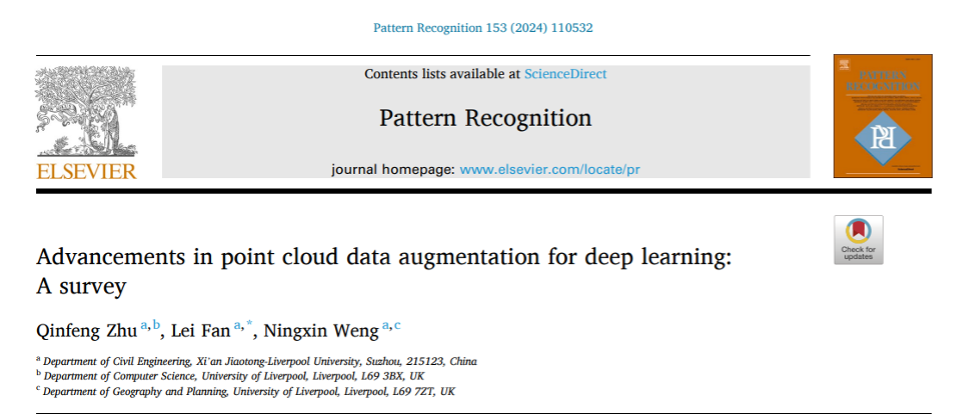
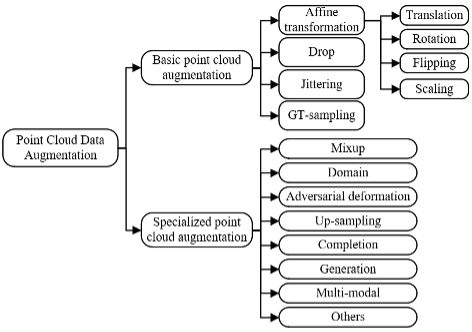
This is the first comprehensive survey A review of point cloud data enhancement methods, covering the latest progress in point cloud data enhancement. Based on the characteristics of the enhancement operation, we propose a classification system of point cloud data enhancement methods. This study summarizes various point cloud data enhancement methods, discusses their applications in typical point cloud processing tasks such as detection, segmentation, and classification, and provides guidance for future Suggestions are provided for potential research.
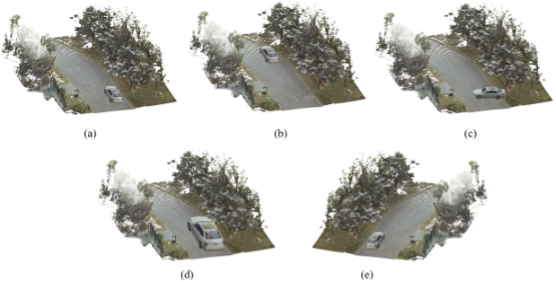
-
研究人员没有充分研究进行点云数据增强的对抗性变形、上采样、补全和生成。鉴于GAN和扩散模型的进步,这些模型可用于生成现实和多样化的点云实例。未来的研究应该在特定点云处理任务的基准数据集上评估这些方法,以评估它们作为增强技术的有效性。 -
目前,很少有研究针对不同的点云处理任务,使用一致的基线网络和数据集来评估点云数据增强方法的性能。这样的评估将增强我们对不同增强方法性能的理解。因此,未来的研究工作可能侧重于建立新的方法、指标和/或数据集,以评估点云数据增强方法的有效性及其对深度学习模型性能的影响。 -
当应用于大规模点云数据集时,某些特定增强方法可能会导致计算成本高昂。未来的工作可以集中在开发有效的算法,在计算成本和增强效率之间进行权衡。此外,一些特定点云增强方法相对复杂,难以复现。建议开发即插即用方法,促进其广泛采用。 -
对于点云数据增强,缺乏普遍接受的基本增强操作组合。因此,未来的工作需要建立一个标准协议,在不牺牲增强效率的情况下,为不同的应用领域、任务和/或数据集选择增强操作。 -
通过增强生成的多个点云变体会影响一致性学习的有效性。目前,据我们所知,一致性学习中只使用了基本的增强方法。探索特定点云增强方法,如对抗变形和生成增强,为提高一致性学习的有效性提供了一种有趣的方法,被认为是一个有价值的未来研究方向。 -
目前,将基础点云增强方法与特定点云增强方法相结合的研究有限。这样的组合有可能进一步增加数据增强的多功能性,值得未来的研究。 -
增强需要真实地模拟点云数据的变化,如物体大小、位置、方向、外观和环境的变化,以确保模拟数据与现实世界的情况保持一致,并保持语义正确。未来的研究可以着眼于标准化各种增强范围,以适应特定的应用场景。 -
某些应用,如目标检测,可能涉及场景中的动态物体。在动态环境中捕获的点云可能需要考虑物体时间变化的特定增强策略。例如,可以设计运动物体的特定轨迹,这可以通过一组组合增强操作来实现,例如平移,旋转和丢弃。 -
ViT在简单组合基本操作的情况下,在分割和分类任务上也取得了较强的性能。当与最先进的ViT作为骨干网络集成时,探索增强方法的性能将是有意义的。
The above is the detailed content of XJTLU and the University of Liverpool propose: the first comprehensive review of point cloud data enhancement. For more information, please follow other related articles on the PHP Chinese website!

Hot AI Tools

Undresser.AI Undress
AI-powered app for creating realistic nude photos

AI Clothes Remover
Online AI tool for removing clothes from photos.

Undress AI Tool
Undress images for free

Clothoff.io
AI clothes remover

Video Face Swap
Swap faces in any video effortlessly with our completely free AI face swap tool!

Hot Article

Hot Tools

Notepad++7.3.1
Easy-to-use and free code editor

SublimeText3 Chinese version
Chinese version, very easy to use

Zend Studio 13.0.1
Powerful PHP integrated development environment

Dreamweaver CS6
Visual web development tools

SublimeText3 Mac version
God-level code editing software (SublimeText3)

Hot Topics










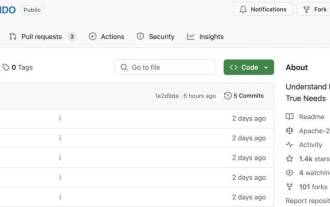
It is also a Tusheng video, but PaintsUndo has taken a different route. ControlNet author LvminZhang started to live again! This time I aim at the field of painting. The new project PaintsUndo has received 1.4kstar (still rising crazily) not long after it was launched. Project address: https://github.com/lllyasviel/Paints-UNDO Through this project, the user inputs a static image, and PaintsUndo can automatically help you generate a video of the entire painting process, from line draft to finished product. follow. During the drawing process, the line changes are amazing. The final video result is very similar to the original image: Let’s take a look at a complete drawing.

The AIxiv column is a column where this site publishes academic and technical content. In the past few years, the AIxiv column of this site has received more than 2,000 reports, covering top laboratories from major universities and companies around the world, effectively promoting academic exchanges and dissemination. If you have excellent work that you want to share, please feel free to contribute or contact us for reporting. Submission email: liyazhou@jiqizhixin.com; zhaoyunfeng@jiqizhixin.com The authors of this paper are all from the team of teacher Zhang Lingming at the University of Illinois at Urbana-Champaign (UIUC), including: Steven Code repair; Deng Yinlin, fourth-year doctoral student, researcher

The AIxiv column is a column where this site publishes academic and technical content. In the past few years, the AIxiv column of this site has received more than 2,000 reports, covering top laboratories from major universities and companies around the world, effectively promoting academic exchanges and dissemination. If you have excellent work that you want to share, please feel free to contribute or contact us for reporting. Submission email: liyazhou@jiqizhixin.com; zhaoyunfeng@jiqizhixin.com In the development process of artificial intelligence, the control and guidance of large language models (LLM) has always been one of the core challenges, aiming to ensure that these models are both powerful and safe serve human society. Early efforts focused on reinforcement learning methods through human feedback (RL
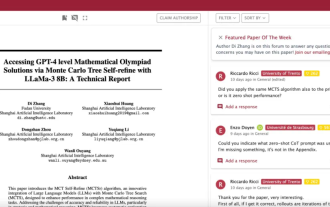
cheers! What is it like when a paper discussion is down to words? Recently, students at Stanford University created alphaXiv, an open discussion forum for arXiv papers that allows questions and comments to be posted directly on any arXiv paper. Website link: https://alphaxiv.org/ In fact, there is no need to visit this website specifically. Just change arXiv in any URL to alphaXiv to directly open the corresponding paper on the alphaXiv forum: you can accurately locate the paragraphs in the paper, Sentence: In the discussion area on the right, users can post questions to ask the author about the ideas and details of the paper. For example, they can also comment on the content of the paper, such as: "Given to

Recently, the Riemann Hypothesis, known as one of the seven major problems of the millennium, has achieved a new breakthrough. The Riemann Hypothesis is a very important unsolved problem in mathematics, related to the precise properties of the distribution of prime numbers (primes are those numbers that are only divisible by 1 and themselves, and they play a fundamental role in number theory). In today's mathematical literature, there are more than a thousand mathematical propositions based on the establishment of the Riemann Hypothesis (or its generalized form). In other words, once the Riemann Hypothesis and its generalized form are proven, these more than a thousand propositions will be established as theorems, which will have a profound impact on the field of mathematics; and if the Riemann Hypothesis is proven wrong, then among these propositions part of it will also lose its effectiveness. New breakthrough comes from MIT mathematics professor Larry Guth and Oxford University
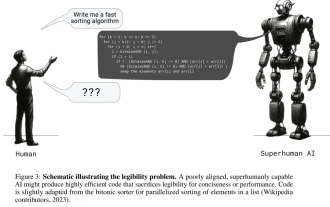
If the answer given by the AI model is incomprehensible at all, would you dare to use it? As machine learning systems are used in more important areas, it becomes increasingly important to demonstrate why we can trust their output, and when not to trust them. One possible way to gain trust in the output of a complex system is to require the system to produce an interpretation of its output that is readable to a human or another trusted system, that is, fully understandable to the point that any possible errors can be found. For example, to build trust in the judicial system, we require courts to provide clear and readable written opinions that explain and support their decisions. For large language models, we can also adopt a similar approach. However, when taking this approach, ensure that the language model generates
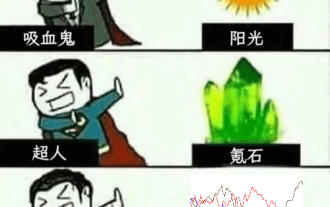
Can language models really be used for time series prediction? According to Betteridge's Law of Headlines (any news headline ending with a question mark can be answered with "no"), the answer should be no. The fact seems to be true: such a powerful LLM cannot handle time series data well. Time series, that is, time series, as the name suggests, refers to a set of data point sequences arranged in the order of time. Time series analysis is critical in many areas, including disease spread prediction, retail analytics, healthcare, and finance. In the field of time series analysis, many researchers have recently been studying how to use large language models (LLM) to classify, predict, and detect anomalies in time series. These papers assume that language models that are good at handling sequential dependencies in text can also generalize to time series.

The AIxiv column is a column where this site publishes academic and technical content. In the past few years, the AIxiv column of this site has received more than 2,000 reports, covering top laboratories from major universities and companies around the world, effectively promoting academic exchanges and dissemination. If you have excellent work that you want to share, please feel free to contribute or contact us for reporting. Submission email: liyazhou@jiqizhixin.com; zhaoyunfeng@jiqizhixin.com. Introduction In recent years, the application of multimodal large language models (MLLM) in various fields has achieved remarkable success. However, as the basic model for many downstream tasks, current MLLM consists of the well-known Transformer network, which
