


DenserRadar: 4D millimeter wave radar point cloud detector based on dense LiDAR point cloud
Original title: DenserRadar: A 4D millimeter-wave radar point cloud detector based on dense LiDAR point clouds
Paper link: https://arxiv.org/pdf/2405.05131
Author's unit: Tsinghua University
Thesis idea:
4D millimeter wave (mmWave) radar is used in extreme environments Its robustness, wide detection range, and ability to measure speed and altitude have shown significant potential to enhance perception when autonomous driving systems face corner-cases. However, the inherent sparsity and noise of 4D millimeter-wave radar point clouds limit its further development and practical applications. This paper introduces a novel 4D millimeter-wave radar point cloud detector that exploits high-resolution dense radar point clouds. Our method constructs dense 3D occupied space ground truth from stitched LiDAR point clouds and uses a specially designed network called DenserRadar. The proposed method surpasses existing probability-based and learning-based millimeter-wave radar point cloud detectors in terms of point cloud density and accuracy, achieving better results on the K-Radar dataset.
Main contribution:
The work of this paper is the first 4D millimeter wave radar point cloud detector supervised by dense 3D occupied data space ground truth. Generated by splicing multi-frame LiDAR point clouds, thereby densifying the detected millimeter wave radar point cloud.
This paper proposes an innovative dense snow 3D occupied data space ground truth generation process, and the splicing of dense LiDAR point clouds of the K-Radar dataset. These point clouds provide comprehensive scene ground truth. In the publication will be available for further research.
Due to the special design of the DenserRadar network, the algorithm in this article is superior to existing CFAR type and learning-based millimeter wave radar point cloud detection methods in terms of point cloud density and accuracy.
Network Design:
Autonomous driving technology aims to provide a safe, convenient and comfortable transportation experience, and its development speed is impressive. To achieve high-level autonomous driving, the ability to perceive and position complex environments is indispensable. Therefore, the sensors equipped on autonomous vehicles, including cameras, lidar (LiDAR) and millimeter-wave radar, as well as their related algorithms, are attracting more and more research interest.
Given its advantages such as compact size, high cost-effectiveness, all-weather adaptability, speed measurement capability, and wide detection range [1], millimeter wave (mmWave) radar has been widely used in the field of autonomous driving. Recent advances in multiple-input multiple-output (MIMO) antenna technology have further improved height resolution, enabling the emergence of 4D millimeter-wave radar. Therefore, 4D millimeter-wave radar is increasingly regarded as a key enhancement of perception and positioning capabilities in autonomous driving, especially in challenging edge scenes such as rain, snow, and fog. As its name suggests, 4D millimeter wave radar can measure four dimensions of target information: range, azimuth, altitude and Doppler velocity, providing a comprehensive sensing solution.
However, the quality of 4D millimeter wave radar point clouds lags significantly behind lidar point clouds. First of all, 4D millimeter wave radar point clouds have low resolution problems, especially in angle measurement. This limitation is mainly due to the antenna configuration and direction of arrival (DOA) estimation of mmWave radar [2]. Secondly, 4D millimeter wave radar point clouds are much sparser than lidar point clouds. Third, due to multipath effects, signal interference and ground reflection, 4D millimeter wave radar point clouds often contain a large number of clutter points. All these shortcomings hinder the application of 4D millimeter wave radar in autonomous driving.
The quality of 4D millimeter wave radar point clouds is not only limited by hardware, but also by signal processing algorithms [3]. In particular, detecting real targets from raw radargrams or tensors to generate point clouds can greatly impact quality. Traditionally, the False Alarm Rate (CFAR) detector and its variants [4], [5] are widely used in the detection of millimeter wave radar point clouds. However, as a probability-based algorithm, CFAR-type detectors may encounter problems when detecting objects of different sizes because these objects are not independent and identically distributed [6], which often occurs in autonomous driving scenarios.
In order to solve the point cloud quality issues related to 4D millimeter wave radar, this paper proposes a learning-based 4D millimeter wave radar point cloud detector, which is composed of dense real-life data generated from lidar point clouds. Information supervision. Initially, this paper stitches multiple frames of pre-processed LiDAR point clouds to generate dense 3D occupancy ground truth. This article then introduces the DenserRadar network, which extracts the features of the original 4D millimeter wave radar tensor and generates a denser and more accurate 4D millimeter wave radar point cloud. The network employs a weighted hybrid loss function along with other novel design elements to capture multi-resolution features and generate point clouds with better resolution than traditional techniques. Comparative experiments conducted on the K-Radar data set [7] prove the effectiveness of this method.
The algorithm of this article is shown in Figure 1. First, this paper designs a ground truth generation process to obtain dense 3D occupied space ground truth as supervision information by splicing multi-frame lidar point cloud data, and then establishes the DenserRadar network, which is tasked with generating data from the original 4D millimeter wave Detection of millimeter wave radar point clouds in radar tensor data.
Figure 1. Overview of the entire algorithm.
Figure 2. Truth value generation flow chart.
Experimental results:
Figure 4. Qualitative point cloud comparison between the DenserRadar algorithm and the CA-CFAR algorithm in this article, attached Images and dense 3D space-occupying ground truth point clouds are used as reference. Each arrow in the diagram represents a length of 10 meters.
Summary:
This article introduces DenserRadar, a novel 4D millimeter wave radar point Cloud detection networks, and an innovative process for generating dense ground truth. Experimental results and ablation studies demonstrate the effectiveness of our network architecture and ground truth generation methodology. This research has the potential to improve the perception and localization capabilities of autonomous driving systems, especially in challenging edge-case scenarios.
The above is the detailed content of DenserRadar: 4D millimeter wave radar point cloud detector based on dense LiDAR point cloud. For more information, please follow other related articles on the PHP Chinese website!

Hot AI Tools

Undresser.AI Undress
AI-powered app for creating realistic nude photos

AI Clothes Remover
Online AI tool for removing clothes from photos.

Undress AI Tool
Undress images for free

Clothoff.io
AI clothes remover

Video Face Swap
Swap faces in any video effortlessly with our completely free AI face swap tool!

Hot Article

Hot Tools

Notepad++7.3.1
Easy-to-use and free code editor

SublimeText3 Chinese version
Chinese version, very easy to use

Zend Studio 13.0.1
Powerful PHP integrated development environment

Dreamweaver CS6
Visual web development tools

SublimeText3 Mac version
God-level code editing software (SublimeText3)

Hot Topics










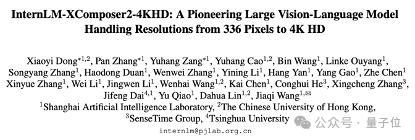
A large model that can automatically analyze the content of PDFs, web pages, posters, and Excel charts is not too convenient for workers. The InternLM-XComposer2-4KHD (abbreviated as IXC2-4KHD) model proposed by Shanghai AILab, the Chinese University of Hong Kong and other research institutions makes this a reality. Compared with other multi-modal large models that have a resolution limit of no more than 1500x1500, this work increases the maximum input image of multi-modal large models to more than 4K (3840x1600) resolution, and supports any aspect ratio and 336 pixels to 4K Dynamic resolution changes. Three days after its release, the model topped the HuggingFace visual question answering model popularity list. Easy to handle
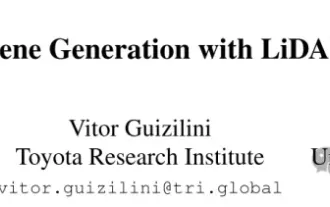
Original title: TowardsRealisticSceneGenerationwithLiDARDiffusionModels Paper link: https://hancyran.github.io/assets/paper/lidar_diffusion.pdf Code link: https://lidar-diffusion.github.io Author affiliation: CMU Toyota Research Institute University of Southern California Paper ideas : Diffusion models (DMs) excel at photorealistic image synthesis, but adapting them to lidar scene generation presents significant challenges. This is mainly because DMs operating in point space have difficulty
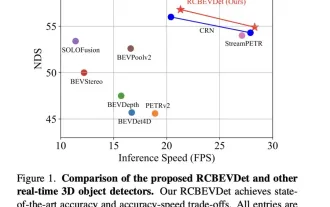
Written above & the author’s personal understanding is that the main issue this discussion paper focuses on is the application of 3D target detection technology in the process of autonomous driving. Although the development of environmental vision camera technology provides high-resolution semantic information for 3D object detection, this method is limited by issues such as the inability to accurately capture depth information and poor performance in bad weather or low-light conditions. In response to this problem, the discussion proposed a new multi-mode 3D target detection method-RCBEVDet that combines surround-view cameras and economical millimeter-wave radar sensors. This method provides richer semantic information and a solution to problems such as poor performance in bad weather or low-light conditions by comprehensively using information from multiple sensors. To address this issue, the discussion proposed a method that combines surround-view cameras
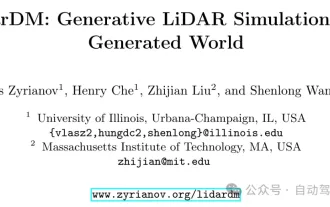
Original title: LidarDM: GenerativeLiDARSimulationinaGeneratedWorld Paper link: https://arxiv.org/pdf/2404.02903.pdf Code link: https://github.com/vzyrianov/lidardm Author affiliation: University of Illinois, Massachusetts Institute of Technology Paper idea: Introduction to this article LidarDM, a novel lidar generation model capable of producing realistic, layout-aware, physically believable, and temporally coherent lidar videos. LidarDM has two unprecedented capabilities in lidar generative modeling: (1)
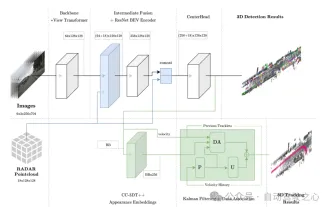
Written above & the author’s personal understanding This article introduces a camera-millimeter wave radar fusion method (CR3DT) for 3D target detection and multi-target tracking. The lidar-based method has set a high standard for this field, but its high computing power and high cost have restricted the development of this solution in the field of autonomous driving; camera-based 3D target detection and tracking solutions are due to their high cost It is relatively low and has attracted the attention of many scholars, but due to its poor results. Therefore, the fusion of cameras and millimeter wave radar is becoming a promising solution. Under the existing camera framework BEVDet, the author fuses the spatial and velocity information of millimeter wave radar and combines it with the CC-3DT++ tracking head to significantly improve the accuracy of 3D target detection and tracking.
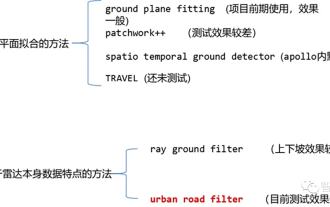
Currently, there are two common laser point cloud segmentation algorithms: methods based on plane fitting and methods based on the characteristics of laser point cloud data. The details are as follows: Point Cloud Ground Segmentation Algorithm 01 Method based on plane fitting - GroundPlaneFitting algorithm idea: A simple processing method is to divide the space into several sub-planes along the x direction (the direction of the car head), and then use The Ground Plane Fitting Algorithm (GPF) results in a ground segmentation method that can handle steep slopes. This method is to fit a global plane in a single frame point cloud. It works better when the number of point clouds is large. When the point cloud is sparse, it is easy to cause missed detections and false detections, such as 16-line lidar. Algorithm pseudocode: The pseudocode algorithm process is the final result of segmentation for a given point cloud P.
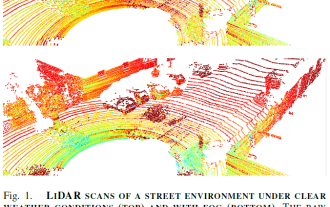
01Abstract Self-driving cars rely on various sensors to collect information about the surrounding environment. The vehicle's behavior is planned based on environmental awareness, so its reliability is crucial for safety reasons. Active lidar sensors are capable of creating accurate 3D representations of scenes, making them a valuable addition to autonomous vehicles’ environmental awareness. LiDAR performance changes in adverse weather conditions such as fog, snow, or rain due to light scattering and occlusion. This limitation has recently spurred considerable research on methods to mitigate perceptual performance degradation. This paper collects, analyzes and discusses different aspects of LiDAR-based environmental sensing to cope with adverse weather conditions. and discusses topics such as the availability of appropriate data, raw point cloud processing and denoising, robust perception algorithms, and sensor fusion to alleviate
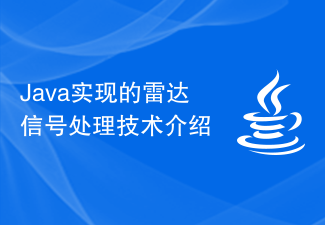
Introduction: With the continuous development of modern science and technology, radar signal processing technology has been increasingly widely used. As one of the most popular programming languages at present, Java is widely used in the implementation of radar signal processing algorithms. This article will introduce the radar signal processing technology implemented in Java. 1. Introduction to radar signal processing technology Radar signal processing technology can be said to be the core and soul of the development of radar systems, and is the key technology to realize the automation and digitization of radar systems. Radar signal processing technology includes waveform processing, filtering, pulse compression, and adaptive beam shaping.
