Python性能优化的20条建议
优化算法时间复杂度
算法的时间复杂度对程序的执行效率影响最大,在Python中可以通过选择合适的数据结构来优化时间复杂度,如list和set查找某一个元素的时间复杂度分别是O(n)和O(1)。不同的场景有不同的优化方式,总得来说,一般有分治,分支界限,贪心,动态规划等思想。
减少冗余数据
如用上三角或下三角的方式去保存一个大的对称矩阵。在0元素占大多数的矩阵里使用稀疏矩阵表示。
合理使用copy与deepcopy
对于dict和list等数据结构的对象,直接赋值使用的是引用的方式。而有些情况下需要复制整个对象,这时可以使用copy包里的copy和deepcopy,这两个函数的不同之处在于后者是递归复制的。效率也不一样:(以下程序在ipython中运行)
import copy a = range(100000) %timeit -n 10 copy.copy(a) # 运行10次 copy.copy(a) %timeit -n 10 copy.deepcopy(a) 10 loops, best of 3: 1.55 ms per loop 10 loops, best of 3: 151 ms per loop
timeit后面的-n表示运行的次数,后两行对应的是两个timeit的输出,下同。由此可见后者慢一个数量级。
使用dict或set查找元素
python dict和set都是使用hash表来实现(类似c++11标准库中unordered_map),查找元素的时间复杂度是O(1)
a = range(1000) s = set(a) d = dict((i,1) for i in a) %timeit -n 10000 100 in d %timeit -n 10000 100 in s 10000 loops, best of 3: 43.5 ns per loop 10000 loops, best of 3: 49.6 ns per loop
dict的效率略高(占用的空间也多一些)。
合理使用生成器(generator)和yield
%timeit -n 100 a = (i for i in range(100000)) %timeit -n 100 b = [i for i in range(100000)] 100 loops, best of 3: 1.54 ms per loop 100 loops, best of 3: 4.56 ms per loop
使用()得到的是一个generator对象,所需要的内存空间与列表的大小无关,所以效率会高一些。在具体应用上,比如set(i for i in range(100000))会比set([i for i in range(100000)])快。
但是对于需要循环遍历的情况:
%timeit -n 10 for x in (i for i in range(100000)): pass %timeit -n 10 for x in [i for i in range(100000)]: pass 10 loops, best of 3: 6.51 ms per loop 10 loops, best of 3: 5.54 ms per loop
后者的效率反而更高,但是如果循环里有break,用generator的好处是显而易见的。yield也是用于创建generator:
def yield_func(ls): for i in ls: yield i+1 def not_yield_func(ls): return [i+1 for i in ls] ls = range(1000000) %timeit -n 10 for i in yield_func(ls):pass %timeit -n 10 for i in not_yield_func(ls):pass 10 loops, best of 3: 63.8 ms per loop 10 loops, best of 3: 62.9 ms per loop
对于内存不是非常大的list,可以直接返回一个list,但是可读性yield更佳(人个喜好)。
python2.x内置generator功能的有xrange函数、itertools包等。
优化循环
循环之外能做的事不要放在循环内,比如下面的优化可以快一倍:
a = range(10000) size_a = len(a) %timeit -n 1000 for i in a: k = len(a) %timeit -n 1000 for i in a: k = size_a 1000 loops, best of 3: 569 µs per loop 1000 loops, best of 3: 256 µs per loop
优化包含多个判断表达式的顺序
对于and,应该把满足条件少的放在前面,对于or,把满足条件多的放在前面。如:
a = range(2000) %timeit -n 100 [i for i in a if 10 < i < 20 or 1000 < i < 2000] %timeit -n 100 [i for i in a if 1000 < i < 2000 or 100 < i < 20] %timeit -n 100 [i for i in a if i % 2 == 0 and i > 1900] %timeit -n 100 [i for i in a if i > 1900 and i % 2 == 0] 100 loops, best of 3: 287 µs per loop 100 loops, best of 3: 214 µs per loop 100 loops, best of 3: 128 µs per loop 100 loops, best of 3: 56.1 µs per loop
使用join合并迭代器中的字符串
In [1]: %%timeit ...: s = '' ...: for i in a: ...: s += i ...: 10000 loops, best of 3: 59.8 µs per loop In [2]: %%timeit s = ''.join(a) ...: 100000 loops, best of 3: 11.8 µs per loop
join对于累加的方式,有大约5倍的提升。
选择合适的格式化字符方式
s1, s2 = 'ax', 'bx' %timeit -n 100000 'abc%s%s' % (s1, s2) %timeit -n 100000 'abc{0}{1}'.format(s1, s2) %timeit -n 100000 'abc' + s1 + s2 100000 loops, best of 3: 183 ns per loop 100000 loops, best of 3: 169 ns per loop 100000 loops, best of 3: 103 ns per loop
三种情况中,%的方式是最慢的,但是三者的差距并不大(都非常快)。(个人觉得%的可读性最好)
不借助中间变量交换两个变量的值
In [3]: %%timeit -n 10000 a,b=1,2 ....: c=a;a=b;b=c; ....: 10000 loops, best of 3: 172 ns per loop In [4]: %%timeit -n 10000 a,b=1,2 a,b=b,a ....: 10000 loops, best of 3: 86 ns per loop
使用a,b=b,a而不是c=a;a=b;b=c;来交换a,b的值,可以快1倍以上。
使用if is
a = range(10000) %timeit -n 100 [i for i in a if i == True] %timeit -n 100 [i for i in a if i is True] 100 loops, best of 3: 531 µs per loop 100 loops, best of 3: 362 µs per loop
使用 if is True 比 if == True 将近快一倍。
使用级联比较x < y < z
x, y, z = 1,2,3 %timeit -n 1000000 if x < y < z:pass %timeit -n 1000000 if x < y and y < z:pass 1000000 loops, best of 3: 101 ns per loop 1000000 loops, best of 3: 121 ns per loop
x < y < z效率略高,而且可读性更好。
while 1 比 while True 更快
def while_1(): n = 100000 while 1: n -= 1 if n <= 0: break def while_true(): n = 100000 while True: n -= 1 if n <= 0: break m, n = 1000000, 1000000 %timeit -n 100 while_1() %timeit -n 100 while_true() 100 loops, best of 3: 3.69 ms per loop 100 loops, best of 3: 5.61 ms per loop
while 1 比 while true快很多,原因是在python2.x中,True是一个全局变量,而非关键字。
使用**而不是pow
%timeit -n 10000 c = pow(2,20) %timeit -n 10000 c = 2**20 10000 loops, best of 3: 284 ns per loop 10000 loops, best of 3: 16.9 ns per loop
**就是快10倍以上!
使用 cProfile, cStringIO 和 cPickle等用c实现相同功能(分别对应profile, StringIO, pickle)的包
import cPickle import pickle a = range(10000) %timeit -n 100 x = cPickle.dumps(a) %timeit -n 100 x = pickle.dumps(a) 100 loops, best of 3: 1.58 ms per loop 100 loops, best of 3: 17 ms per loop
由c实现的包,速度快10倍以上!
使用最佳的反序列化方式
下面比较了eval, cPickle, json方式三种对相应字符串反序列化的效率:
import json import cPickle a = range(10000) s1 = str(a) s2 = cPickle.dumps(a) s3 = json.dumps(a) %timeit -n 100 x = eval(s1) %timeit -n 100 x = cPickle.loads(s2) %timeit -n 100 x = json.loads(s3) 100 loops, best of 3: 16.8 ms per loop 100 loops, best of 3: 2.02 ms per loop 100 loops, best of 3: 798 µs per loop
可见json比cPickle快近3倍,比eval快20多倍。
使用C扩展(Extension)
目前主要有CPython(python最常见的实现的方式)原生API, ctypes,Cython,cffi三种方式,它们的作用是使得Python程序可以调用由C编译成的动态链接库,其特点分别是:
CPython原生API: 通过引入Python.h头文件,对应的C程序中可以直接使用Python的数据结构。实现过程相对繁琐,但是有比较大的适用范围。
ctypes: 通常用于封装(wrap)C程序,让纯Python程序调用动态链接库(Windows中的dll或Unix中的so文件)中的函数。如果想要在python中使用已经有C类库,使用ctypes是很好的选择,有一些基准测试下,python2+ctypes是性能最好的方式。
Cython: Cython是CPython的超集,用于简化编写C扩展的过程。Cython的优点是语法简洁,可以很好地兼容numpy等包含大量C扩展的库。Cython的使得场景一般是针对项目中某个算法或过程的优化。在某些测试中,可以有几百倍的性能提升。
cffi: cffi的就是ctypes在pypy(详见下文)中的实现,同进也兼容CPython。cffi提供了在python使用C类库的方式,可以直接在python代码中编写C代码,同时支持链接到已有的C类库。
使用这些优化方式一般是针对已有项目性能瓶颈模块的优化,可以在少量改动原有项目的情况下大幅度地提高整个程序的运行效率。
并行编程
因为GIL的存在,Python很难充分利用多核CPU的优势。但是,可以通过内置的模块multiprocessing实现下面几种并行模式:
多进程:对于CPU密集型的程序,可以使用multiprocessing的Process,Pool等封装好的类,通过多进程的方式实现并行计算。但是因为进程中的通信成本比较大,对于进程之间需要大量数据交互的程序效率未必有大的提高。
多线程:对于IO密集型的程序,multiprocessing.dummy模块使用multiprocessing的接口封装threading,使得多线程编程也变得非常轻松(比如可以使用Pool的map接口,简洁高效)。
分布式:multiprocessing中的Managers类提供了可以在不同进程之共享数据的方式,可以在此基础上开发出分布式的程序。
不同的业务场景可以选择其中的一种或几种的组合实现程序性能的优化。
终级大杀器:PyPy
PyPy是用RPython(CPython的子集)实现的Python,根据官网的基准测试数据,它比CPython实现的Python要快6倍以上。快的原因是使用了Just-in-Time(JIT)编译器,即动态编译器,与静态编译器(如gcc,javac等)不同,它是利用程序运行的过程的数据进行优化。由于历史原因,目前pypy中还保留着GIL,不过正在进行的STM项目试图将PyPy变成没有GIL的Python。
如果python程序中含有C扩展(非cffi的方式),JIT的优化效果会大打折扣,甚至比CPython慢(比Numpy)。所以在PyPy中最好用纯Python或使用cffi扩展。
随着STM,Numpy等项目的完善,相信PyPy将会替代CPython。
使用性能分析工具
除了上面在ipython使用到的timeit模块,还有cProfile。cProfile的使用方式也非常简单: python -m cProfile filename.py,filename.py 是要运行程序的文件名,可以在标准输出中看到每一个函数被调用的次数和运行的时间,从而找到程序的性能瓶颈,然后可以有针对性地优化。
参考
[1] http://www.ibm.com/developerworks/cn/linux/l-cn-python-optim/
[2] http://maxburstein.com/blog/speeding-up-your-python-code/
原文:http://segmentfault.com/blog/defool/1190000000666603

Hot AI Tools

Undresser.AI Undress
AI-powered app for creating realistic nude photos

AI Clothes Remover
Online AI tool for removing clothes from photos.

Undress AI Tool
Undress images for free

Clothoff.io
AI clothes remover

Video Face Swap
Swap faces in any video effortlessly with our completely free AI face swap tool!

Hot Article

Hot Tools

Notepad++7.3.1
Easy-to-use and free code editor

SublimeText3 Chinese version
Chinese version, very easy to use

Zend Studio 13.0.1
Powerful PHP integrated development environment

Dreamweaver CS6
Visual web development tools

SublimeText3 Mac version
God-level code editing software (SublimeText3)

Hot Topics
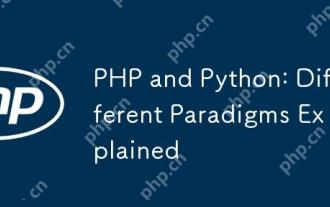
PHP is mainly procedural programming, but also supports object-oriented programming (OOP); Python supports a variety of paradigms, including OOP, functional and procedural programming. PHP is suitable for web development, and Python is suitable for a variety of applications such as data analysis and machine learning.
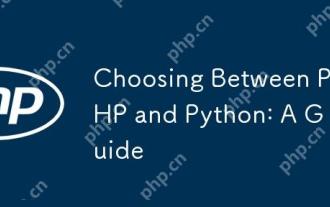
PHP is suitable for web development and rapid prototyping, and Python is suitable for data science and machine learning. 1.PHP is used for dynamic web development, with simple syntax and suitable for rapid development. 2. Python has concise syntax, is suitable for multiple fields, and has a strong library ecosystem.
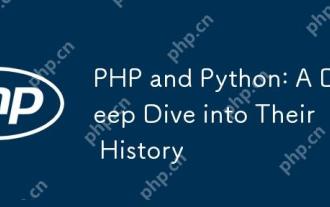
PHP originated in 1994 and was developed by RasmusLerdorf. It was originally used to track website visitors and gradually evolved into a server-side scripting language and was widely used in web development. Python was developed by Guidovan Rossum in the late 1980s and was first released in 1991. It emphasizes code readability and simplicity, and is suitable for scientific computing, data analysis and other fields.
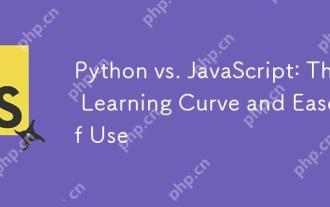
Python is more suitable for beginners, with a smooth learning curve and concise syntax; JavaScript is suitable for front-end development, with a steep learning curve and flexible syntax. 1. Python syntax is intuitive and suitable for data science and back-end development. 2. JavaScript is flexible and widely used in front-end and server-side programming.
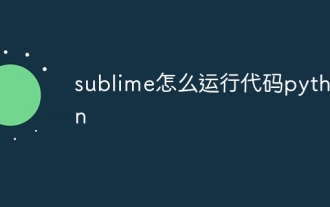
To run Python code in Sublime Text, you need to install the Python plug-in first, then create a .py file and write the code, and finally press Ctrl B to run the code, and the output will be displayed in the console.
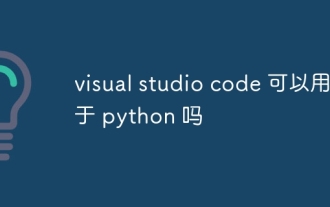
VS Code can be used to write Python and provides many features that make it an ideal tool for developing Python applications. It allows users to: install Python extensions to get functions such as code completion, syntax highlighting, and debugging. Use the debugger to track code step by step, find and fix errors. Integrate Git for version control. Use code formatting tools to maintain code consistency. Use the Linting tool to spot potential problems ahead of time.
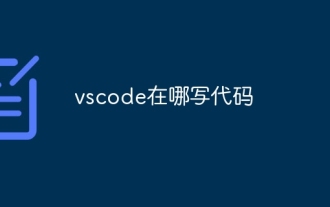
Writing code in Visual Studio Code (VSCode) is simple and easy to use. Just install VSCode, create a project, select a language, create a file, write code, save and run it. The advantages of VSCode include cross-platform, free and open source, powerful features, rich extensions, and lightweight and fast.
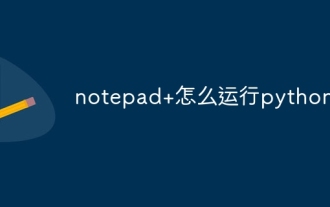
Running Python code in Notepad requires the Python executable and NppExec plug-in to be installed. After installing Python and adding PATH to it, configure the command "python" and the parameter "{CURRENT_DIRECTORY}{FILE_NAME}" in the NppExec plug-in to run Python code in Notepad through the shortcut key "F6".
