使用Autogen中的代码执行人完成复杂的任务
AI agents are designed to act autonomously, solving problems and executing tasks in dynamic environments. A key feature in Autogen, enabling their adaptability is AutoGen’s code executors. This feature along with LLMs enables AI agents to generate, evaluate, and execute code in real-time. This capability bridges the gap between static AI models and actionable intelligence. By automating workflows, performing data analysis, and debugging complex systems, it transforms agents from mere thinkers into effective doers. In this article, we will learn more about code executors in AutoGen and how to implement them.
Table of Contents
- Types of Code Executors in AutoGen
- How to Build AI Agents with Code Executors in AutoGen?
- Pre-requisites
- Building an AI Agent Using Command Line Executor
- Building an ML Model Using Jupyter Code Executor
- Building an AI Agent Using Custom Executor
- Conclusion
- Frequently Asked Questions
Types of Code Executors in AutoGen
AutoGen has three kinds of code executors that can be used for different purposes.
- Command Line Executor: It allows AI agents to run the code in the command line. It will save each code block to a separate file and execute that file. This executor is ideal for automating tasks like file management, script execution, or handling external tools. It provides flexibility and low-level control in a workflow.
- Jupyter Code Executor: It enables agents to execute Python code within a Jupyter-like environment. Here, you can define variables in one code block and reuse them in subsequent blocks. One advantage of this setup is that when an error occurs, only the specific block of code with the error needs to be re-executed, rather than the entire script.
- Custom Code Executor: It gives developers the ability to create specialized code execution logic. For example, the custom code executor can access variables defined in the environment without explicitly providing them to the LLM.
These Code Executors can be run on both the host machine (local) as well as the Docker containers.
Also Read: 4 Steps to Build Multi-Agent Nested Chats with AutoGen
How to Build AI Agents with Code Executors in AutoGen?
Now let’s learn how you can use these different code executors in AutoGen:
Pre-requisites
Before building AI agents, ensure you have the necessary API keys for the required LLMs.
Load the .env file with the API keys needed.
from dotenv import load_dotenv load_dotenv(./env)
Key Libraries Required
autogen-agentchat – 0.2.38
jupyter_kernel_gateway-3.0.1
Building an AI Agent Using Command Line Executor
Let’s build an AI agent to know the offers and discounts available on an e-commerce website using the command line executor. Here are the steps to follow.
1. Import the necessary libraries.
from autogen import ConversableAgent, AssistantAgent, UserProxyAgent from autogen.coding import LocalCommandLineCodeExecutor, DockerCommandLineCodeExecutor
2. Define the agents.
user_proxy = UserProxyAgent( name="User", llm_config=False, is_termination_msg=lambda msg: msg.get("content") is not None and "TERMINATE" in msg["content"], human_input_mode="TERMINATE", code_execution_config=False ) code_writer_agent = ConversableAgent( name="CodeWriter", system_message="""You are a Python developer. You use your coding skill to solve problems. Once the task is done, returns 'TERMINATE'.""", llm_config={"config_list": [{"model": "gpt-4o-mini"}]}, ) local_executor = LocalCommandLineCodeExecutor( timeout=15, work_dir='./code files') local_executor_agent = ConversableAgent( "local_executor_agent", llm_config=False, code_execution_config={"executor": local_executor}, human_input_mode="ALWAYS", )
We are using the ‘local_executor’ in the code_execution_config of the local_executor_agent.
3. Define the messages which are used to initialize the chat.
messages = ["""To check whether there are any offers or discounts available on a given e-commerce website - https://www.flipkart.com/ Follow these steps, 1. download the html page of the given URL 2. we only need html content, so remove any CSS, JavaScript, and Image tags content 3. save the remaining html content. """ , "read the text and list all the offers and discounts available"] # Intialize the chat chat_result = local_executor_agent.initiate_chat( code_writer_agent, message=messages[0], )
It will ask for human input after each message from the codeWriter agent. You just need to press the ‘Enter’ key to execute the code written by the agent. We can also any further instructions if there is any problem with the code.
Here are the questions we have asked and the output at the end.
As we can see, with the mentioned questions, we can get a list of offers and discounts from an e-commerce website.
Also Read: Hands-on Guide to Building Multi-Agent Chatbots with AutoGen
Building an ML Model Using Jupyter Code Executor
By using this, we can access the variables defined in one code block from another code block, unlike the command line executor.
Now, let’s try to build an ML model using this.
1. Import the additional methods.
from autogen.coding.jupyter import LocalJupyterServer, DockerJupyterServer, JupyterCodeExecutor from pathlib import Path
2. Initialize the jupyter server and output directory.
server = LocalJupyterServer() output_dir = Path("coding") output_dir.mkdir()
Note that LocalJupyterServer may not function on Windows due to a bug. In this case, you can use the DockerJupyterServer instead or use the EmbeddedIPythonCodeExecutor.
3. Define the executor agent and writer agent with a custom system message.
jupyter_executor_agent = ConversableAgent( name="jupyter_executor_agent", llm_config=False, code_execution_config={ "executor": JupyterCodeExecutor(server, output_dir=output_dir), }, human_input_mode="ALWAYS", ) code_writer_system_message = """ You have been given coding capability to solve tasks using Python code in a stateful IPython kernel. You are responsible for writing the code, and the user is responsible for executing the code. When you write Python code, put the code in a markdown code block with the language set to Python. For example: ```python x = 3 ``` You can use the variable `x` in subsequent code blocks. ```python print(x) ``` Always use print statements for the output of the code. Write code incrementally and leverage the statefulness of the kernel to avoid repeating code. Import libraries in a separate code block. Define a function or a class in a separate code block. Run code that produces output in a separate code block. Run code that involves expensive operations like download, upload, and call external APIs in a separate code block. When your code produces an output, the output will be returned to you. Because you have limited conversation memory, if your code creates an image, the output will be a path to the image instead of the image itself.""" code_writer_agent = ConversableAgent( "code_writer", system_message=code_writer_system_message, llm_config={"config_list": [{"model": "gpt-4o"}]}, human_input_mode="TERMINATE", )
4. Define the initial message and initialize the chat
message = "read the datasets/user_behavior_dataset.csv and print what the data is about" chat_result = jupyter_executor_agent.initiate_chat( code_writer_agent, message=message, ) # Once the chat is completed we can stop the server. server.stop()
5. Once the chat is completed we can stop the server.
We can print the messages as follows
for chat in chat_result.chat_history[:]: if chat['name'] == 'code_writer' and 'TERMINATE' not in chat['content']: print("--------agent-----------") print(chat['content']) if chat['name'] == 'jupyter_executor_agent' and 'exitcode' not in chat['content']: print("--------user------------") print(chat['content'])
Here’s the sample
As we can see, we can get the code generated by the agent and also the results after executing the code.
Also Read: Building Agentic Chatbots Using AutoGen
Building an AI Agent Using Custom Executor
Now, let’s try to create a custom executor that can run the code in the same jupyter notebook where we are creating this executor. So, we can read a CSV file, and then ask an agent to build an ML model on the already imported file.
Here’s how we’ll do it.
1. Import the necessary libraries.
import pandas as pd from typing import List from IPython import get_ipython from autogen.coding import CodeBlock, CodeExecutor, CodeExtractor, CodeResult, MarkdownCodeExtractor
2. Define the executor that can extract and run the code from jupyter cells.
class NotebookExecutor(CodeExecutor): @property def code_extractor(self) -> CodeExtractor: # Extact code from markdown blocks. return MarkdownCodeExtractor() def __init__(self) -> None: # Get the current IPython instance running in this notebook. self._ipython = get_ipython() def execute_code_blocks(self, code_blocks: List[CodeBlock]) -> CodeResult: log = "" for code_block in code_blocks: result = self._ipython.run_cell("%%capture --no-display cap\n" + code_block.code) log += self._ipython.ev("cap.stdout") log += self._ipython.ev("cap.stderr") if result.result is not None: log += str(result.result) exitcode = 0 if result.success else 1 if result.error_before_exec is not None: log += f"\n{result.error_before_exec}" exitcode = 1 if result.error_in_exec is not None: log += f"\n{result.error_in_exec}" exitcode = 1 if exitcode != 0: break return CodeResult(exit_code=exitcode, output=log)
3. Define the agents.
code_writer_agent = ConversableAgent( name="CodeWriter", system_message="You are a helpful AI assistant.\n" "You use your coding skill to solve problems.\n" "You have access to a IPython kernel to execute Python code.\n" "You can suggest Python code in Markdown blocks, each block is a cell.\n" "The code blocks will be executed in the IPython kernel in the order you suggest them.\n" "All necessary libraries have already been installed.\n" "Add return or print statements to the code to get the output\n" "Once the task is done, returns 'TERMINATE'.", llm_config={"config_list": [{"model": "gpt-4o-mini"}]}, ) code_executor_agent = ConversableAgent( name="CodeExecutor", llm_config=False, code_execution_config={"executor": NotebookExecutor()}, is_termination_msg=lambda msg: "TERMINATE" in msg.get("content", "").strip().upper(), human_input_mode="ALWAYS" )
4. Read the file and initiate the chat with the file.
df = pd.read_csv('datasets/mountains_vs_beaches_preferences.csv') chat_result = code_executor_agent.initiate_chat( code_writer_agent, message="What are the column names in the dataframe defined above as df?", )
5. We can print the chat history as follows:
for chat in chat_result.chat_history[:]: if chat['name'] == 'CodeWriter' and 'TERMINATE' not in chat['content']: print("--------agent-----------") print(chat['content']) if chat['name'] == 'CodeExecutor' and 'exitcode' not in chat['content']: print("--------user------------") print(chat['content'])
As we can see again, we can get the code generated by the agent and also the results after executing the code.
Conclusion
AutoGen’s code executors provide flexibility and functionality for AI agents to perform real-world tasks. The command line executor enables script execution, while the Jupyter code executor supports iterative development. Custom executors, on the other hand, allow developers to create tailored workflows.
These tools empower AI agents to transition from problem solvers to solution implementers. Developers can use these features to build intelligent systems that deliver actionable insights and automate complex processes.
Frequently Asked Questions
Q1. What is the primary purpose of Code Executors in AutoGen?A. Code Executors in AutoGen allow AI agents to generate, execute, and evaluate code in real time. This enables agents to automate tasks, perform data analysis, debug systems, and implement dynamic workflows.
Q2. What are the differences between Command Line and Jupyter Code Executors?A. The Command Line Executor saves and executes code as separate files, ideal for tasks like file management and script execution. The Jupyter Code Executor operates in a stateful environment, allowing reuse of variables and selective re-execution of code blocks, making it more suitable for iterative coding tasks like building ML models.
Q3. Can Code Executors be used with Docker containers?A. Yes, both the Command Line Executor and Jupyter Code Executor can be configured to run on Docker containers, providing a flexible environment for execution.
Q4. What is the advantage of using a Custom Code Executor?A. Custom Code Executors allow developers to define specialized execution logic, such as running code within the same Jupyter notebook. This is useful for tasks requiring a high level of integration or customization.
Q5. What are the prerequisites for using Code Executors in AutoGen?A. Before using Code Executors, ensure you have the necessary API keys for your preferred LLMs. You should also have the required libraries, such as `autogen-agentchat` and `jupyter_kernel_gateway`, installed in your environment.
以上是使用Autogen中的代码执行人完成复杂的任务的详细内容。更多信息请关注PHP中文网其他相关文章!

热AI工具

Undresser.AI Undress
人工智能驱动的应用程序,用于创建逼真的裸体照片

AI Clothes Remover
用于从照片中去除衣服的在线人工智能工具。

Undress AI Tool
免费脱衣服图片

Clothoff.io
AI脱衣机

Video Face Swap
使用我们完全免费的人工智能换脸工具轻松在任何视频中换脸!

热门文章

热工具

记事本++7.3.1
好用且免费的代码编辑器

SublimeText3汉化版
中文版,非常好用

禅工作室 13.0.1
功能强大的PHP集成开发环境

Dreamweaver CS6
视觉化网页开发工具

SublimeText3 Mac版
神级代码编辑软件(SublimeText3)
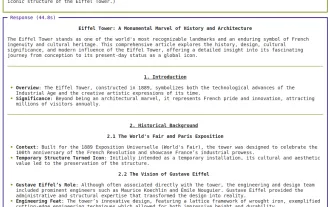
在从事代理AI时,开发人员经常发现自己在速度,灵活性和资源效率之间进行权衡。我一直在探索代理AI框架,并遇到了Agno(以前是Phi-
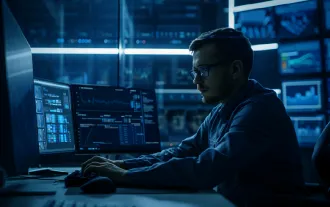
SQL的Alter表语句:动态地将列添加到数据库 在数据管理中,SQL的适应性至关重要。 需要即时调整数据库结构吗? Alter表语句是您的解决方案。本指南的详细信息添加了Colu
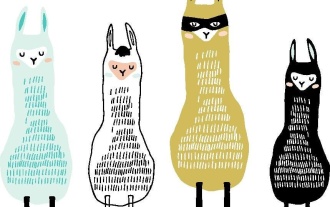
陷入困境的基准:骆驼案例研究 2025年4月上旬,梅塔(Meta)揭开了Llama 4套件的模特套件,具有令人印象深刻的性能指标,使他们对GPT-4O和Claude 3.5 Sonnet等竞争对手有利地定位。伦斯的中心
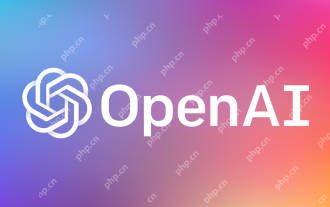
该版本包括三种不同的型号,GPT-4.1,GPT-4.1 MINI和GPT-4.1 NANO,标志着向大语言模型景观内的特定任务优化迈进。这些模型并未立即替换诸如
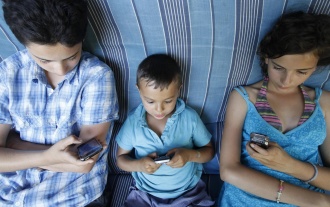
视频游戏可以缓解焦虑,建立焦点或支持多动症的孩子吗? 随着医疗保健在全球范围内挑战,尤其是在青年中的挑战,创新者正在转向一种不太可能的工具:视频游戏。现在是世界上最大的娱乐印度河之一
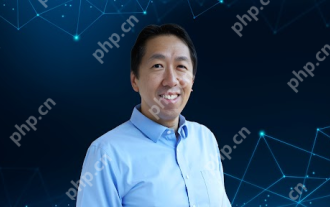
解锁嵌入模型的力量:深入研究安德鲁·NG的新课程 想象一个未来,机器可以完全准确地理解和回答您的问题。 这不是科幻小说;多亏了AI的进步,它已成为R
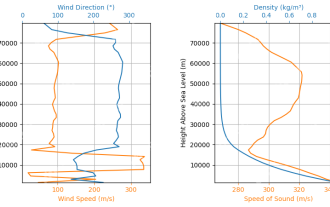
模拟火箭发射的火箭发射:综合指南 本文指导您使用强大的Python库Rocketpy模拟高功率火箭发射。 我们将介绍从定义火箭组件到分析模拟的所有内容
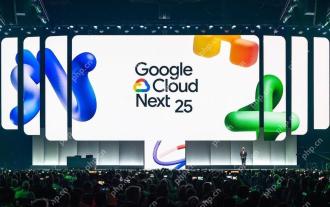
双子座是Google AI策略的基础 双子座是Google AI代理策略的基石,它利用其先进的多模式功能来处理和生成跨文本,图像,音频,视频和代码的响应。由DeepM开发
