使用 AWS Bedrock 部署 AI 交通拥堵预测器:完整概述
我们都喜欢交通,对吧?这是我唯一一次思考我是如何完全搞砸了我的演示(想太多是一种痛苦)。
抛开所有笑话,我想创建一个项目,可以作为 PoC 实时查找流量,以便将来进一步增强它。了解交通拥堵预测器。
我将逐步介绍如何使用 AWS Bedrock 部署交通拥堵预测器。 AWS Bedrock 为基础模型提供完全托管的服务,使其非常适合部署 AI 应用程序。我们将涵盖从初始设置到最终部署和测试的所有内容。
现在,先决条件
- 具有适当权限的AWS帐户(必须使用我的借记卡进行验证,因为我认为它在一定限制内可以免费使用。痛苦)。
- Python 3.8
- 交通拥堵预测器代码(来自之前的开发)
- 已安装并配置 AWS CLI
- Python 和 AWS 服务的基本知识就足够了。
第 1 步:准备环境
首先,设置您的开发环境:
# Create a new virtual environment python -m venv bedrock-env source bedrock-env/bin/activate # On Windows use: bedrock-env\Scripts\activate # Install required packages pip install boto3 pandas numpy scikit-learn streamlit plotly
第 2 步:AWS Bedrock 设置
导航到 AWS 控制台并启用 AWS Bedrock
在基岩中创建新模型:
- 转到 AWS Bedrock 控制台
- 选择“模型访问权限”
- 请求访问克劳德模型家族
- 等待批准(通常是即时的,但任何事情都可能发生)
步骤 3:修改 Bedrock 集成代码
创建一个新文件“bedrock_integration.py”:
import boto3 import json import numpy as np import pandas as pd from typing import Dict, Any class TrafficPredictor: def __init__(self): self.bedrock = boto3.client( service_name='bedrock-runtime', region_name='us-east-1' # Change to your region ) def prepare_features(self, input_data: Dict[str, Any]) -> pd.DataFrame: # Convert input data to model features hour = input_data['hour'] day = input_data['day'] features = pd.DataFrame({ 'hour_sin': [np.sin(2 * np.pi * hour/24)], 'hour_cos': [np.cos(2 * np.pi * hour/24)], 'day_sin': [np.sin(2 * np.pi * day/7)], 'day_cos': [np.cos(2 * np.pi * day/7)], 'temperature': [input_data['temperature']], 'precipitation': [input_data['precipitation']], 'special_event': [input_data['special_event']], 'road_work': [input_data['road_work']], 'vehicle_count': [input_data['vehicle_count']] }) return features def predict(self, input_data: Dict[str, Any]) -> float: features = self.prepare_features(input_data) # Prepare prompt for Claude prompt = f""" Based on the following traffic conditions, predict the congestion level (0-10): - Time: {input_data['hour']}:00 - Day of week: {input_data['day']} - Temperature: {input_data['temperature']}°C - Precipitation: {input_data['precipitation']}mm - Special event: {'Yes' if input_data['special_event'] else 'No'} - Road work: {'Yes' if input_data['road_work'] else 'No'} - Vehicle count: {input_data['vehicle_count']} Return only the numerical prediction. """ # Call Bedrock response = self.bedrock.invoke_model( modelId='anthropic.claude-v2', body=json.dumps({ "prompt": prompt, "max_tokens": 10, "temperature": 0 }) ) # Parse response response_body = json.loads(response['body'].read()) prediction = float(response_body['completion'].strip()) return np.clip(prediction, 0, 10)
第4步:创建FastAPI后端
创建“api.py:”
from fastapi import FastAPI, HTTPException from pydantic import BaseModel from bedrock_integration import TrafficPredictor from typing import Dict, Any app = FastAPI() predictor = TrafficPredictor() class PredictionInput(BaseModel): hour: int day: int temperature: float precipitation: float special_event: bool road_work: bool vehicle_count: int @app.post("/predict") async def predict_traffic(input_data: PredictionInput) -> Dict[str, float]: try: prediction = predictor.predict(input_data.dict()) return {"congestion_level": prediction} except Exception as e: raise HTTPException(status_code=500, detail=str(e))
第 5 步:创建 AWS 基础设施
创建“基础设施.py”:
import boto3 import json def create_infrastructure(): # Create ECR repository ecr = boto3.client('ecr') try: ecr.create_repository(repositoryName='traffic-predictor') except ecr.exceptions.RepositoryAlreadyExistsException: pass # Create ECS cluster ecs = boto3.client('ecs') ecs.create_cluster(clusterName='traffic-predictor-cluster') # Create task definition task_def = { 'family': 'traffic-predictor', 'containerDefinitions': [{ 'name': 'traffic-predictor', 'image': f'{ecr.describe_repositories()["repositories"][0]["repositoryUri"]}:latest', 'memory': 512, 'cpu': 256, 'essential': True, 'portMappings': [{ 'containerPort': 8000, 'hostPort': 8000, 'protocol': 'tcp' }] }], 'requiresCompatibilities': ['FARGATE'], 'networkMode': 'awsvpc', 'cpu': '256', 'memory': '512' } ecs.register_task_definition(**task_def)
第 6 步:将应用程序容器化
创建“Dockerfile:”
FROM python:3.9-slim WORKDIR /app COPY requirements.txt . RUN pip install --no-cache-dir -r requirements.txt COPY . . CMD ["uvicorn", "api:app", "--host", "0.0.0.0", "--port", "8000"]
创建“requirements.txt:”
fastapi uvicorn boto3 pandas numpy scikit-learn
第 7 步:部署到 AWS
运行这些命令:
# Build and push Docker image aws ecr get-login-password --region us-east-1 | docker login --username AWS --password-stdin $AWS_ACCOUNT_ID.dkr.ecr.us-east-1.amazonaws.com docker build -t traffic-predictor . docker tag traffic-predictor:latest $AWS_ACCOUNT_ID.dkr.ecr.us-east-1.amazonaws.com/traffic-predictor:latest docker push $AWS_ACCOUNT_ID.dkr.ecr.us-east-1.amazonaws.com/traffic-predictor:latest # Create infrastructure python infrastructure.py
第 8 步:更新 Streamlit 前端
修改“app.py”以连接到API:
import streamlit as st import requests import plotly.graph_objects as go import plotly.express as px API_ENDPOINT = "your-api-endpoint" def predict_traffic(input_data): response = requests.post(f"{API_ENDPOINT}/predict", json=input_data) return response.json()["congestion_level"] # Rest of the Streamlit code remains the same, but replace direct model calls # with API calls using predict_traffic()
第 9 步:测试和监控
测试 API 端点:
curl -X POST "your-api-endpoint/predict" \ -H "Content-Type: application/json" \ -d '{"hour":12,"day":1,"temperature":25,"precipitation":0,"special_event":false,"road_work":false,"vehicle_count":1000}'
使用 AWS CloudWatch 进行监控:
- 设置 CloudWatch 仪表板
- 创建错误率和延迟警报
- 监控 API 使用情况和成本
如果一切顺利的话。恭喜!您已成功部署交通拥堵预测器。为了那个,垫垫自己的背!确保监控成本和性能、定期更新模型并实施 CI/CD 管道。下一步是添加用户身份验证、增强监控和警报、优化模型性能以及根据用户反馈添加更多功能。
感谢您阅读本文。让我知道任何想法、问题或观察!
以上是使用 AWS Bedrock 部署 AI 交通拥堵预测器:完整概述的详细内容。更多信息请关注PHP中文网其他相关文章!

热AI工具

Undresser.AI Undress
人工智能驱动的应用程序,用于创建逼真的裸体照片

AI Clothes Remover
用于从照片中去除衣服的在线人工智能工具。

Undress AI Tool
免费脱衣服图片

Clothoff.io
AI脱衣机

Video Face Swap
使用我们完全免费的人工智能换脸工具轻松在任何视频中换脸!

热门文章

热工具

记事本++7.3.1
好用且免费的代码编辑器

SublimeText3汉化版
中文版,非常好用

禅工作室 13.0.1
功能强大的PHP集成开发环境

Dreamweaver CS6
视觉化网页开发工具

SublimeText3 Mac版
神级代码编辑软件(SublimeText3)
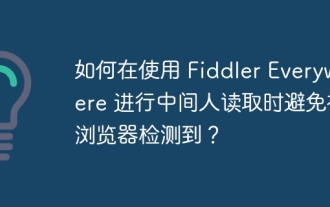
使用FiddlerEverywhere进行中间人读取时如何避免被检测到当你使用FiddlerEverywhere...
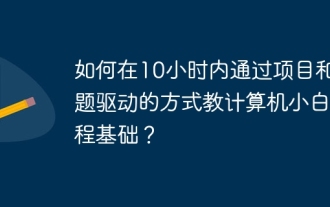
如何在10小时内教计算机小白编程基础?如果你只有10个小时来教计算机小白一些编程知识,你会选择教些什么�...
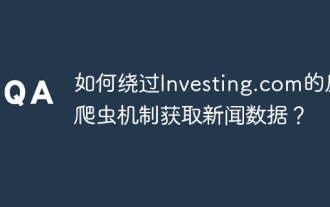
攻克Investing.com的反爬虫策略许多人尝试爬取Investing.com(https://cn.investing.com/news/latest-news)的新闻数据时,常常�...
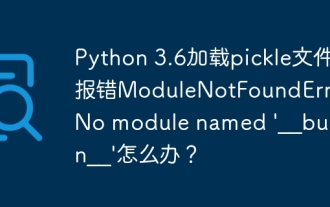
Python3.6环境下加载pickle文件报错:ModuleNotFoundError:Nomodulenamed...
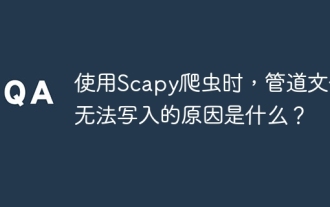
使用Scapy爬虫时管道文件无法写入的原因探讨在学习和使用Scapy爬虫进行数据持久化存储时,可能会遇到管道文�...
