使用 Regex 和 spaCy 屏蔽提示中的机密数据
人们对 OpenAI、Gemini、Claude 等流行的法学硕士存在隐私问题。除非它是开源模型,否则我们真的不知道屏幕后面发生了什么。所以,我们必须要小心。
第一件事是处理我们传递给法学硕士的信息。专家建议避免在提示中包含机密信息或个人标识符。听起来更容易,但随着法学硕士上下文大小的增加,我们可以将大文本传递给模型。因此,它可能会变得严格审查并掩盖所有标识符。
因此,我尝试创建 python 脚本来检测和屏蔽标识符和机密信息。正则表达式很神奇,可以识别不同的机密信息并用掩码替换它。还使用 spacy 库来检测常见标识符,例如名称、地点等,
注意:目前,这适用于印度语境,但仍然可以检测到通用标识符。
那么让我们看看实现(我已经在LLM的帮助下实现了)
如果你想跳过解释。
这是代码库的链接:aditykris/prompt-masker-Indian-context
导入必要的模块/库
import re from typing import Dict, List, Tuple import spacy nlp = spacy.load("en_core_web_sm")
您必须使用以下代码段手动安装“en_core_web_sm”
python -m spacy download en_core_web_sm
设置印度共同机密信息。
class IndianIdentifier: '''Regex for common Indian identifiers''' PAN = r'[A-Z]{5}[0-9]{4}[A-Z]{1}' AADHAR = r'[2-9]{1}[0-9]{3}\s[0-9]{4}\s[0-9]{4}' INDIAN_PASSPORT = r'[A-PR-WYa-pr-wy][1-9]\d\s?\d{4}[1-9]' DRIVING_LICENSE = r'(([A-Z]{2}[0-9]{2})( )|([A-Z]{2}-[0-9]{2}))((19|20)[0-9][0-9])[0-9]{7}' UPI_ID = r'[\.\-a-z0-9]+@[a-z]+' INDIAN_BANK_ACCOUNT = r'\d{9,18}' IFSC_CODE = r'[A-Z]{4}0[A-Z0-9]{6}' INDIAN_PHONE_NUMBER = r'(\+91|\+91\-|0)?[789]\d{9}' EMAIL = r'[\w\.-]+@[\w\.-]+\.\w+' @classmethod def get_all_patterns(cls) -> Dict[str, str]: """Returns all regex patterns defined in the class""" return { name: pattern for name, pattern in vars(cls).items() if isinstance(pattern, str) and not name.startswith('_') }
所以,我正在修改 python 类和方法,因此在这里实现它。
我从 DebugPointer 中找到了这些标识符的正则表达式,非常有帮助。
现在介绍检测功能。简单的 re.finditer() 用于循环不同的模式以查找匹配项。匹配项存储在列表中。
def find_matches(text: str, pattern: str) -> List[Tuple[int, int, str]]: """ Find all matches of a pattern in text and return their positions and matched text """ matches = [] for match in re.finditer(pattern, text): matches.append((match.start(), match.end(), match.group())) return matches
使用简单的字典来存储替换文本。将其包装在一个函数中以返回替换文本。
def get_replacement_text(identifier_type: str) -> str: """ Returns appropriate replacement text based on the type of identifier """ replacements = { 'PAN': '[PAN_NUMBER]', 'AADHAR': '[AADHAR_NUMBER]', 'INDIAN_PASSPORT': '[PASSPORT_NUMBER]', 'DRIVING_LICENSE': '[DL_NUMBER]', 'UPI_ID': '[UPI_ID]', 'INDIAN_BANK_ACCOUNT': '[BANK_ACCOUNT]', 'IFSC_CODE': '[IFSC_CODE]', 'INDIAN_PHONE_NUMBER': '[PHONE_NUMBER]', 'EMAIL': '[EMAIL_ADDRESS]', 'PERSON': '[PERSON_NAME]', 'ORG': '[ORGANIZATION]', 'GPE': '[LOCATION]' } return replacements.get(identifier_type, '[MASKED]')
啊!主要部分开始。
def analyze_identifiers(text: str) -> Tuple[str, Dict[str, List[str]]]: """ Function to identify and hide sensitive information. Returns: - masked_text: Text with all sensitive information masked - found_identifiers: Dictionary containing all identified sensitive information """ # Initialize variables masked_text = text found_identifiers = {} positions_to_mask = [] # First, find all regex matches for identifier_name, pattern in IndianIdentifier.get_all_patterns().items(): matches = find_matches(text, pattern) if matches: found_identifiers[identifier_name] = [match[2] for match in matches] positions_to_mask.extend( (start, end, identifier_name) for start, end, _ in matches ) # Then, process named entities using spaCy doc = nlp(text) for ent in doc.ents: if ent.label_ in ["PERSON", "ORG", "GPE"]: positions_to_mask.append((ent.start_char, ent.end_char, ent.label_)) if ent.label_ not in found_identifiers: found_identifiers[ent.label_] = [] found_identifiers[ent.label_].append(ent.text) # Sort positions by start index in reverse order to handle overlapping matches positions_to_mask.sort(key=lambda x: x[0], reverse=True) # Apply masking for start, end, identifier_type in positions_to_mask: replacement = get_replacement_text(identifier_type) masked_text = masked_text[:start] + replacement + masked_text[end:] return masked_text, found_identifiers
此函数将提示作为输入,并将屏蔽的提示与识别的元素一起作为字典返回。
让我一一解释一下。
以下循环通过不同标识符的正则表达式来查找提示中的匹配项。如果找到,那么它将:
1. 将识别的信息存储在字典中,以标识符类型作为键来跟踪。
2. 记下位置并将其存储在positions_to_mask中,以便我们稍后可以应用遮罩。
import re from typing import Dict, List, Tuple import spacy nlp = spacy.load("en_core_web_sm")
现在是空闲时间。它是一个很棒的自然语言处理 (nlp) 任务库。我们可以使用 nlp 模块从文本中提取标识符。
目前,我已经习惯了它检测姓名、组织和位置。
这与上面的循环相同,用于识别和存储位置。
class IndianIdentifier: '''Regex for common Indian identifiers''' PAN = r'[A-Z]{5}[0-9]{4}[A-Z]{1}' AADHAR = r'[2-9]{1}[0-9]{3}\s[0-9]{4}\s[0-9]{4}' INDIAN_PASSPORT = r'[A-PR-WYa-pr-wy][1-9]\d\s?\d{4}[1-9]' DRIVING_LICENSE = r'(([A-Z]{2}[0-9]{2})( )|([A-Z]{2}-[0-9]{2}))((19|20)[0-9][0-9])[0-9]{7}' UPI_ID = r'[\.\-a-z0-9]+@[a-z]+' INDIAN_BANK_ACCOUNT = r'\d{9,18}' IFSC_CODE = r'[A-Z]{4}0[A-Z0-9]{6}' INDIAN_PHONE_NUMBER = r'(\+91|\+91\-|0)?[789]\d{9}' EMAIL = r'[\w\.-]+@[\w\.-]+\.\w+' @classmethod def get_all_patterns(cls) -> Dict[str, str]: """Returns all regex patterns defined in the class""" return { name: pattern for name, pattern in vars(cls).items() if isinstance(pattern, str) and not name.startswith('_') }
在一些测试用例中,我注意到一些掩码丢失了,这主要是由于标识符重叠造成的。所以,逆序排序有助于解决这个问题。
def find_matches(text: str, pattern: str) -> List[Tuple[int, int, str]]: """ Find all matches of a pattern in text and return their positions and matched text """ matches = [] for match in re.finditer(pattern, text): matches.append((match.start(), match.end(), match.group())) return matches
最后,我们使用来自found_identifiers和positions_to_mask的数据来屏蔽发生。
def get_replacement_text(identifier_type: str) -> str: """ Returns appropriate replacement text based on the type of identifier """ replacements = { 'PAN': '[PAN_NUMBER]', 'AADHAR': '[AADHAR_NUMBER]', 'INDIAN_PASSPORT': '[PASSPORT_NUMBER]', 'DRIVING_LICENSE': '[DL_NUMBER]', 'UPI_ID': '[UPI_ID]', 'INDIAN_BANK_ACCOUNT': '[BANK_ACCOUNT]', 'IFSC_CODE': '[IFSC_CODE]', 'INDIAN_PHONE_NUMBER': '[PHONE_NUMBER]', 'EMAIL': '[EMAIL_ADDRESS]', 'PERSON': '[PERSON_NAME]', 'ORG': '[ORGANIZATION]', 'GPE': '[LOCATION]' } return replacements.get(identifier_type, '[MASKED]')
该程序的示例输入为:
输入:
def analyze_identifiers(text: str) -> Tuple[str, Dict[str, List[str]]]: """ Function to identify and hide sensitive information. Returns: - masked_text: Text with all sensitive information masked - found_identifiers: Dictionary containing all identified sensitive information """ # Initialize variables masked_text = text found_identifiers = {} positions_to_mask = [] # First, find all regex matches for identifier_name, pattern in IndianIdentifier.get_all_patterns().items(): matches = find_matches(text, pattern) if matches: found_identifiers[identifier_name] = [match[2] for match in matches] positions_to_mask.extend( (start, end, identifier_name) for start, end, _ in matches ) # Then, process named entities using spaCy doc = nlp(text) for ent in doc.ents: if ent.label_ in ["PERSON", "ORG", "GPE"]: positions_to_mask.append((ent.start_char, ent.end_char, ent.label_)) if ent.label_ not in found_identifiers: found_identifiers[ent.label_] = [] found_identifiers[ent.label_].append(ent.text) # Sort positions by start index in reverse order to handle overlapping matches positions_to_mask.sort(key=lambda x: x[0], reverse=True) # Apply masking for start, end, identifier_type in positions_to_mask: replacement = get_replacement_text(identifier_type) masked_text = masked_text[:start] + replacement + masked_text[end:] return masked_text, found_identifiers
输出:
蒙版文本:
for identifier_name, pattern in IndianIdentifier.get_all_patterns().items(): matches = find_matches(text, pattern) if matches: found_identifiers[identifier_name] = [match[2] for match in matches] positions_to_mask.extend( (start, end, identifier_name) for start, end, _ in matches )
以上是使用 Regex 和 spaCy 屏蔽提示中的机密数据的详细内容。更多信息请关注PHP中文网其他相关文章!

热AI工具

Undresser.AI Undress
人工智能驱动的应用程序,用于创建逼真的裸体照片

AI Clothes Remover
用于从照片中去除衣服的在线人工智能工具。

Undress AI Tool
免费脱衣服图片

Clothoff.io
AI脱衣机

Video Face Swap
使用我们完全免费的人工智能换脸工具轻松在任何视频中换脸!

热门文章

热工具

记事本++7.3.1
好用且免费的代码编辑器

SublimeText3汉化版
中文版,非常好用

禅工作室 13.0.1
功能强大的PHP集成开发环境

Dreamweaver CS6
视觉化网页开发工具

SublimeText3 Mac版
神级代码编辑软件(SublimeText3)
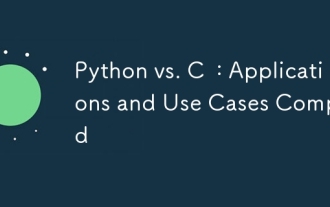
Python适合数据科学、Web开发和自动化任务,而C 适用于系统编程、游戏开发和嵌入式系统。 Python以简洁和强大的生态系统着称,C 则以高性能和底层控制能力闻名。
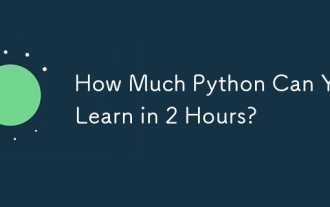
两小时内可以学到Python的基础知识。1.学习变量和数据类型,2.掌握控制结构如if语句和循环,3.了解函数的定义和使用。这些将帮助你开始编写简单的Python程序。
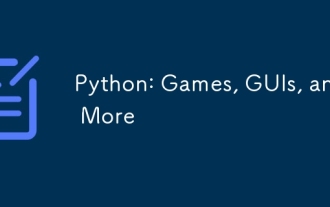
Python在游戏和GUI开发中表现出色。1)游戏开发使用Pygame,提供绘图、音频等功能,适合创建2D游戏。2)GUI开发可选择Tkinter或PyQt,Tkinter简单易用,PyQt功能丰富,适合专业开发。
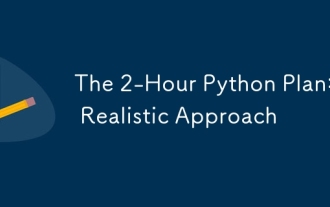
2小时内可以学会Python的基本编程概念和技能。1.学习变量和数据类型,2.掌握控制流(条件语句和循环),3.理解函数的定义和使用,4.通过简单示例和代码片段快速上手Python编程。
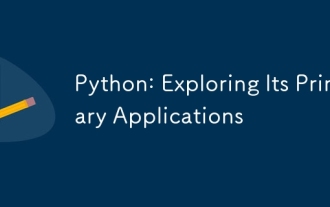
Python在web开发、数据科学、机器学习、自动化和脚本编写等领域有广泛应用。1)在web开发中,Django和Flask框架简化了开发过程。2)数据科学和机器学习领域,NumPy、Pandas、Scikit-learn和TensorFlow库提供了强大支持。3)自动化和脚本编写方面,Python适用于自动化测试和系统管理等任务。
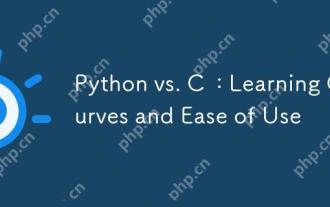
Python更易学且易用,C 则更强大但复杂。1.Python语法简洁,适合初学者,动态类型和自动内存管理使其易用,但可能导致运行时错误。2.C 提供低级控制和高级特性,适合高性能应用,但学习门槛高,需手动管理内存和类型安全。
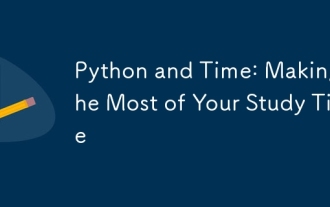
要在有限的时间内最大化学习Python的效率,可以使用Python的datetime、time和schedule模块。1.datetime模块用于记录和规划学习时间。2.time模块帮助设置学习和休息时间。3.schedule模块自动化安排每周学习任务。
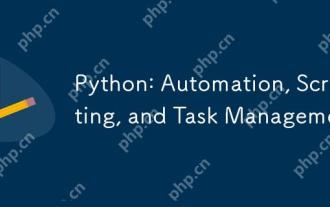
Python在自动化、脚本编写和任务管理中表现出色。1)自动化:通过标准库如os、shutil实现文件备份。2)脚本编写:使用psutil库监控系统资源。3)任务管理:利用schedule库调度任务。Python的易用性和丰富库支持使其在这些领域中成为首选工具。
