如何使用 Z 分数识别并删除 Pandas DataFrame 中的异常值?
识别和排除 pandas DataFrame 中的异常值
在具有多个列的 pandas DataFrame 中,根据特定列值识别和排除异常值可以提高数据的准确性和可靠性。离群值或显着偏离大多数数据的极值可能会扭曲分析结果并导致错误的结论。
要有效过滤离群值,一种稳健的方法是依靠统计技术。一种方法涉及使用 Z 分数,它衡量一个值与平均值的标准差有多少。 Z 分数超过预定义阈值的行可被视为异常值。
使用 sciPy.stats.zscore
sciPy 库提供 zscore() 函数来计算 Z -DataFrame 中每列的分数。这是一个检测和排除异常值的优雅解决方案:
import pandas as pd import numpy as np from scipy import stats df = pd.DataFrame({'Vol': [1200, 1220, 1215, 4000, 1210]}) outlier_threshold = 3 # Compute Z-scores for the 'Vol' column zscores = np.abs(stats.zscore(df['Vol'])) # Create a mask to identify rows with outliers outlier_mask = zscores > outlier_threshold # Exclude rows with outliers df_without_outliers = df[~outlier_mask]
这种方法可以有效识别异常值行并将其从 DataFrame 中删除。
处理多列
如果有多列,异常值检测可以应用于特定列或所有列同时:
# Outliers in at least one column outlier_mask = (np.abs(stats.zscore(df)) < outlier_threshold).all(axis=1) # Remove rows with outliers in any column df_without_outliers = df[~outlier_mask]
# Outliers in a specific column ('Vol') zscores = np.abs(stats.zscore(df['Vol'])) outlier_mask = zscores > outlier_threshold # Remove rows with outliers in the 'Vol' column df_without_outliers = df[~outlier_mask]
通过采用Z-score计算等统计方法,可以有效地检测和排除pandas DataFrame中的异常值,确保分析数据更干净、更可靠。
以上是如何使用 Z 分数识别并删除 Pandas DataFrame 中的异常值?的详细内容。更多信息请关注PHP中文网其他相关文章!

热AI工具

Undresser.AI Undress
人工智能驱动的应用程序,用于创建逼真的裸体照片

AI Clothes Remover
用于从照片中去除衣服的在线人工智能工具。

Undress AI Tool
免费脱衣服图片

Clothoff.io
AI脱衣机

Video Face Swap
使用我们完全免费的人工智能换脸工具轻松在任何视频中换脸!

热门文章

热工具

记事本++7.3.1
好用且免费的代码编辑器

SublimeText3汉化版
中文版,非常好用

禅工作室 13.0.1
功能强大的PHP集成开发环境

Dreamweaver CS6
视觉化网页开发工具

SublimeText3 Mac版
神级代码编辑软件(SublimeText3)
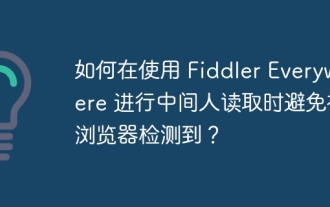
使用FiddlerEverywhere进行中间人读取时如何避免被检测到当你使用FiddlerEverywhere...
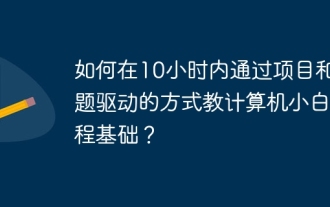
如何在10小时内教计算机小白编程基础?如果你只有10个小时来教计算机小白一些编程知识,你会选择教些什么�...
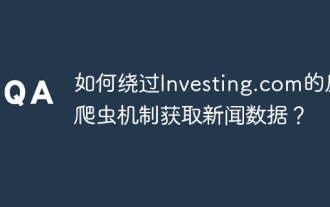
攻克Investing.com的反爬虫策略许多人尝试爬取Investing.com(https://cn.investing.com/news/latest-news)的新闻数据时,常常�...
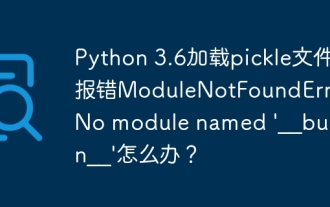
Python3.6环境下加载pickle文件报错:ModuleNotFoundError:Nomodulenamed...
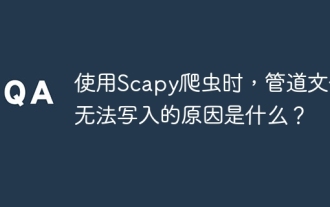
使用Scapy爬虫时管道文件无法写入的原因探讨在学习和使用Scapy爬虫进行数据持久化存储时,可能会遇到管道文�...
