Building RustyNum: a NumPy Alternative with Rust and Python
Hey Dev Community!
I wanted to share a side project I’ve been working on called RustyNum. As someone who uses NumPy daily for data processing and scientific computing, I often wondered how challenging it would be to create a similar library from scratch using Rust and Python. This curiosity sparked the development of RustyNum—a lightweight alternative to NumPy that leverages Rust’s powerful features.
What is RustyNum?
RustyNum combines the speed and memory safety of Rust with the simplicity and flexibility of Python. One of the standout features is that it's using Rust’s portable SIMD (Single Instruction, Multiple Data) feature, which allows RustyNum to optimize computations across different CPU architectures seamlessly. This means you can achieve high-performance array manipulations without leaving the Python ecosystem. I wanted to learn building a library from scratch and as a result RustyNum is not using any 3rd party dependencies.
Why RustyNum?
- Performance Boost: By utilizing Rust’s portable SIMD, RustyNum can handle performance-critical tasks more efficiently than traditional Python libraries.
- Memory Safety: Rust ensures memory safety without a garbage collector, reducing the risk of memory leaks and segmentation faults.
- Learning Experience: This project has been a fantastic way for me to dive deeper into Rust-Python interoperability and explore the intricacies of building numerical libraries.
- Because no external dependencies are used the Python wheels are super small (300kBytes) compared to alternatives such as Numpy (>10MBytes).
When to Consider RustyNum:
If you’re working on data analysis, scientific computing, or small-scale machine learning projects and find NumPy a bit heavy for your needs, RustyNum might be the perfect fit. It’s especially useful when you need optimized performance across various hardware without the complexity of integrating with C-based libraries. However, be aware that the library is pretty much in its early days and only covers basic operations from Numpy as of today.
You can check out RustyNum on GitHub. I’d love to hear your feedback, suggestions, or contributions!
Thanks for reading, and happy coding!
Cheers,
Igor
以上是Building RustyNum: a NumPy Alternative with Rust and Python的详细内容。更多信息请关注PHP中文网其他相关文章!

热AI工具

Undresser.AI Undress
人工智能驱动的应用程序,用于创建逼真的裸体照片

AI Clothes Remover
用于从照片中去除衣服的在线人工智能工具。

Undress AI Tool
免费脱衣服图片

Clothoff.io
AI脱衣机

Video Face Swap
使用我们完全免费的人工智能换脸工具轻松在任何视频中换脸!

热门文章

热工具

记事本++7.3.1
好用且免费的代码编辑器

SublimeText3汉化版
中文版,非常好用

禅工作室 13.0.1
功能强大的PHP集成开发环境

Dreamweaver CS6
视觉化网页开发工具

SublimeText3 Mac版
神级代码编辑软件(SublimeText3)
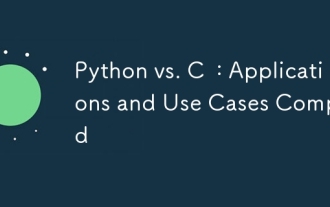
Python适合数据科学、Web开发和自动化任务,而C 适用于系统编程、游戏开发和嵌入式系统。 Python以简洁和强大的生态系统着称,C 则以高性能和底层控制能力闻名。
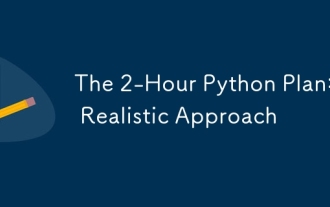
2小时内可以学会Python的基本编程概念和技能。1.学习变量和数据类型,2.掌握控制流(条件语句和循环),3.理解函数的定义和使用,4.通过简单示例和代码片段快速上手Python编程。
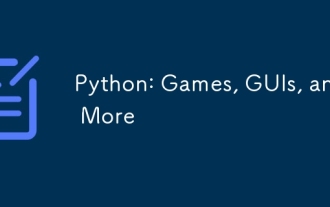
Python在游戏和GUI开发中表现出色。1)游戏开发使用Pygame,提供绘图、音频等功能,适合创建2D游戏。2)GUI开发可选择Tkinter或PyQt,Tkinter简单易用,PyQt功能丰富,适合专业开发。
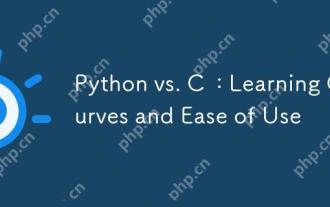
Python更易学且易用,C 则更强大但复杂。1.Python语法简洁,适合初学者,动态类型和自动内存管理使其易用,但可能导致运行时错误。2.C 提供低级控制和高级特性,适合高性能应用,但学习门槛高,需手动管理内存和类型安全。
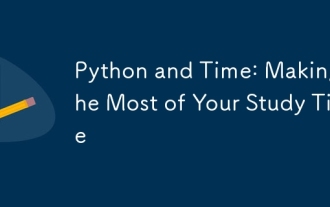
要在有限的时间内最大化学习Python的效率,可以使用Python的datetime、time和schedule模块。1.datetime模块用于记录和规划学习时间。2.time模块帮助设置学习和休息时间。3.schedule模块自动化安排每周学习任务。
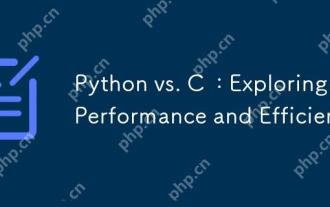
Python在开发效率上优于C ,但C 在执行性能上更高。1.Python的简洁语法和丰富库提高开发效率。2.C 的编译型特性和硬件控制提升执行性能。选择时需根据项目需求权衡开发速度与执行效率。
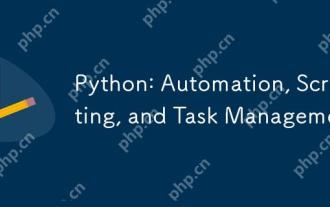
Python在自动化、脚本编写和任务管理中表现出色。1)自动化:通过标准库如os、shutil实现文件备份。2)脚本编写:使用psutil库监控系统资源。3)任务管理:利用schedule库调度任务。Python的易用性和丰富库支持使其在这些领域中成为首选工具。
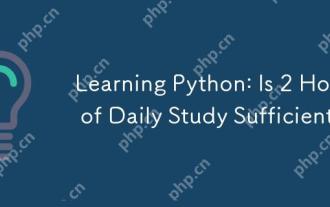
每天学习Python两个小时是否足够?这取决于你的目标和学习方法。1)制定清晰的学习计划,2)选择合适的学习资源和方法,3)动手实践和复习巩固,可以在这段时间内逐步掌握Python的基本知识和高级功能。
