


California-based ChainOpera bridges AI and web3 with a new decentralized platform and app marketplace for devs
ChainOpera AI, a California-based startup, has officially launched its decentralized AI platform and generative AI app ecosystem. The primary motive of the company is to create an open and trustworthy AI economy. They do this by linking people who need AI tools with a network of shared computer power, quality data, and the skills of developers. This way, anyone can help create and use AI, making it more accessible and trustworthy.
CEO Salman Avestimehr specifically emphasized the need for personalized AI tools that are easy to train, and grow with the user base. ChainOpera’s platform is designed to bridge the gap between AI developers and decentralized resources, enabling the creation, training, and deployment of advanced AI models. Unlike other web3 AI projects that focus solely on infrastructure (like SingularityNET and Fetch.ai), ChainOpera claims to deliver a complete, user-friendly platform, much like a decentralized version of AWS Bedrock.
Alongside the platform, ChainOpera has also launched a decentralized AI app marketplace, allowing developers to launch generative AI applications utilizing community-driven models and resources. The report further states that users can contribute data and feedback towards these apps, earning rewards in the process.
ChainOpera’s founders, Salman Avestimehr and Aiden He, have deep roots in decentralized AI. In 2020, they developed FedML, an open-source framework for collaborative, decentralized machine learning, which quickly gained traction among global developers. Building on that success, they launched TensorOpera in 2022, providing enterprise-grade AI services through partnerships, such as with Qualcomm, to deploy AI across multi-cloud environments. By merging both AI and web3, ChainOpera is trying to set the stage for a more open and collaborative AI future, where everyone can contribute, build, and benefit from Artificial Intelligence.
以上是California-based ChainOpera bridges AI and web3 with a new decentralized platform and app marketplace for devs的详细内容。更多信息请关注PHP中文网其他相关文章!

热AI工具

Undresser.AI Undress
人工智能驱动的应用程序,用于创建逼真的裸体照片

AI Clothes Remover
用于从照片中去除衣服的在线人工智能工具。

Undress AI Tool
免费脱衣服图片

Clothoff.io
AI脱衣机

Video Face Swap
使用我们完全免费的人工智能换脸工具轻松在任何视频中换脸!

热门文章

热工具

记事本++7.3.1
好用且免费的代码编辑器

SublimeText3汉化版
中文版,非常好用

禅工作室 13.0.1
功能强大的PHP集成开发环境

Dreamweaver CS6
视觉化网页开发工具

SublimeText3 Mac版
神级代码编辑软件(SublimeText3)
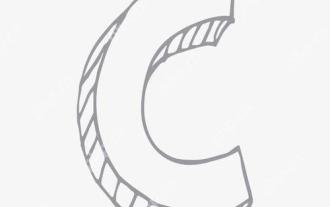
DMA在C 中是指DirectMemoryAccess,直接内存访问技术,允许硬件设备直接与内存进行数据传输,不需要CPU干预。1)DMA操作高度依赖于硬件设备和驱动程序,实现方式因系统而异。2)直接访问内存可能带来安全风险,需确保代码的正确性和安全性。3)DMA可提高性能,但使用不当可能导致系统性能下降。通过实践和学习,可以掌握DMA的使用技巧,在高速数据传输和实时信号处理等场景中发挥其最大效能。
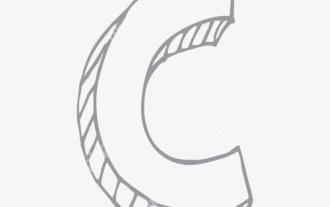
使用C 中的chrono库可以让你更加精确地控制时间和时间间隔,让我们来探讨一下这个库的魅力所在吧。C 的chrono库是标准库的一部分,它提供了一种现代化的方式来处理时间和时间间隔。对于那些曾经饱受time.h和ctime折磨的程序员来说,chrono无疑是一个福音。它不仅提高了代码的可读性和可维护性,还提供了更高的精度和灵活性。让我们从基础开始,chrono库主要包括以下几个关键组件:std::chrono::system_clock:表示系统时钟,用于获取当前时间。std::chron
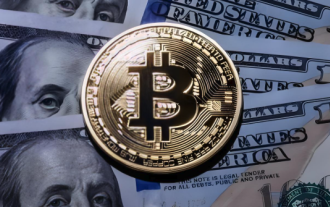
交易所内置量化工具包括:1. Binance(币安):提供Binance Futures量化模块,低手续费,支持AI辅助交易。2. OKX(欧易):支持多账户管理和智能订单路由,提供机构级风控。独立量化策略平台有:3. 3Commas:拖拽式策略生成器,适用于多平台对冲套利。4. Quadency:专业级算法策略库,支持自定义风险阈值。5. Pionex:内置16 预设策略,低交易手续费。垂直领域工具包括:6. Cryptohopper:云端量化平台,支持150 技术指标。7. Bitsgap:
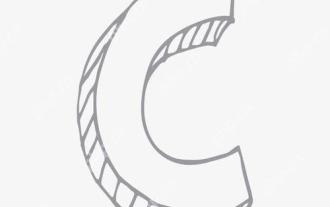
在C 中处理高DPI显示可以通过以下步骤实现:1)理解DPI和缩放,使用操作系统API获取DPI信息并调整图形输出;2)处理跨平台兼容性,使用如SDL或Qt的跨平台图形库;3)进行性能优化,通过缓存、硬件加速和动态调整细节级别来提升性能;4)解决常见问题,如模糊文本和界面元素过小,通过正确应用DPI缩放来解决。
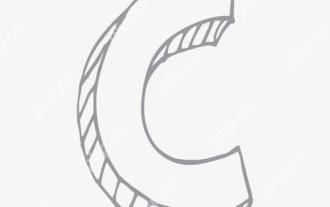
C 在实时操作系统(RTOS)编程中表现出色,提供了高效的执行效率和精确的时间管理。1)C 通过直接操作硬件资源和高效的内存管理满足RTOS的需求。2)利用面向对象特性,C 可以设计灵活的任务调度系统。3)C 支持高效的中断处理,但需避免动态内存分配和异常处理以保证实时性。4)模板编程和内联函数有助于性能优化。5)实际应用中,C 可用于实现高效的日志系统。
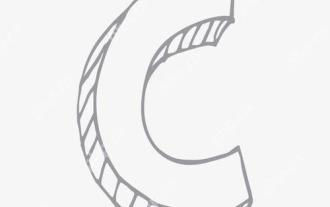
在C 中测量线程性能可以使用标准库中的计时工具、性能分析工具和自定义计时器。1.使用库测量执行时间。2.使用gprof进行性能分析,步骤包括编译时添加-pg选项、运行程序生成gmon.out文件、生成性能报告。3.使用Valgrind的Callgrind模块进行更详细的分析,步骤包括运行程序生成callgrind.out文件、使用kcachegrind查看结果。4.自定义计时器可灵活测量特定代码段的执行时间。这些方法帮助全面了解线程性能,并优化代码。
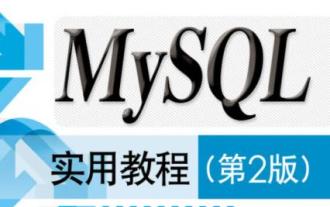
在MySQL中,添加字段使用ALTERTABLEtable_nameADDCOLUMNnew_columnVARCHAR(255)AFTERexisting_column,删除字段使用ALTERTABLEtable_nameDROPCOLUMNcolumn_to_drop。添加字段时,需指定位置以优化查询性能和数据结构;删除字段前需确认操作不可逆;使用在线DDL、备份数据、测试环境和低负载时间段修改表结构是性能优化和最佳实践。
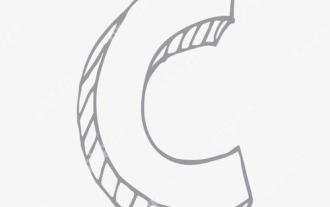
C 中使用字符串流的主要步骤和注意事项如下:1.创建输出字符串流并转换数据,如将整数转换为字符串。2.应用于复杂数据结构的序列化,如将vector转换为字符串。3.注意性能问题,避免在处理大量数据时频繁使用字符串流,可考虑使用std::string的append方法。4.注意内存管理,避免频繁创建和销毁字符串流对象,可以重用或使用std::stringstream。
