Python と FaceNet による顔認識
このガイドでは、facenet-pytorch を使用した顔の類似性検出ツールを説明します。このツールは、FaceNet モデルの高品質の顔埋め込みを利用して、ターゲット画像を複数の候補と比較して、最も近いものを特定します。 実装を見てみましょう。
必須のツールとライブラリ
- PyTorch: 深層学習操作の基盤。
- FaceNet-PyTorch: 顔検出と埋め込み生成用の事前トレーニング済みモデルを提供します。
- 枕 (PIL): 画像操作タスクを処理します。
- Matplotlib: 結果の視覚化に使用されます。
2 つのコア モデルが採用されています:
- MTCNN: 画像内の顔を検出します。
- InceptionResnetV1: 顔の埋め込みを抽出します。
初期化
import torch from facenet_pytorch import MTCNN, InceptionResnetV1 from PIL import Image import requests from io import BytesIO import matplotlib.pyplot as plt # Initialize face detection (MTCNN) and embedding extraction (InceptionResnetV1) modules. mtcnn = MTCNN(image_size=160, keep_all=True) resnet = InceptionResnetV1(pretrained='vggface2').eval()
関数の定義
1.画像の読み込みと埋め込み抽出:
この関数は、URL から画像を取得し、顔を検出し、埋め込みを計算します。
def get_embedding_and_face(image_path): """Loads an image, detects faces, and returns the embedding and detected face.""" try: response = requests.get(image_path) response.raise_for_status() content_type = response.headers.get('Content-Type') if 'image' not in content_type: raise ValueError(f"Invalid image URL: {content_type}") image = Image.open(BytesIO(response.content)).convert("RGB") except Exception as e: print(f"Image loading error from {image_path}: {e}") return None, None faces, probs = mtcnn(image, return_prob=True) if faces is None or len(faces) == 0: return None, None embedding = resnet(faces[0].unsqueeze(0)) return embedding, faces[0]
2.テンソルから画像への変換:
表示用にテンソルを準備します。
def tensor_to_image(tensor): """Converts a normalized tensor to a displayable image array.""" image = tensor.permute(1, 2, 0).detach().numpy() image = (image - image.min()) / (image.max() - image.min()) image = (image * 255).astype('uint8') return image
3.最も似ている顔の識別:
ターゲット画像の埋め込みを候補の埋め込みと比較します。
def find_most_similar(target_image_path, candidate_image_paths): """Identifies the most similar image to the target from a list of candidates.""" target_emb, target_face = get_embedding_and_face(target_image_path) if target_emb is None: raise ValueError("No face detected in the target image.") highest_similarity = float('-inf') most_similar_face = None most_similar_image_path = None candidate_faces = [] similarities = [] for candidate_image_path in candidate_image_paths: candidate_emb, candidate_face = get_embedding_and_face(candidate_image_path) if candidate_emb is None: similarities.append(None) candidate_faces.append(None) continue similarity = torch.nn.functional.cosine_similarity(target_emb, candidate_emb).item() similarities.append(similarity) candidate_faces.append(candidate_face) if similarity > highest_similarity: highest_similarity = similarity most_similar_face = candidate_face most_similar_image_path = candidate_image_path # Visualization plt.figure(figsize=(12, 8)) # Display target image plt.subplot(2, len(candidate_image_paths) + 1, 1) plt.imshow(tensor_to_image(target_face)) plt.title("Target Image") plt.axis("off") # Display most similar image if most_similar_face is not None: plt.subplot(2, len(candidate_image_paths) + 1, 2) plt.imshow(tensor_to_image(most_similar_face)) plt.title("Most Similar") plt.axis("off") # Display all candidates with similarity scores for idx, (candidate_face, similarity) in enumerate(zip(candidate_faces, similarities)): plt.subplot(2, len(candidate_image_paths) + 1, idx + len(candidate_image_paths) + 2) if candidate_face is not None: plt.imshow(tensor_to_image(candidate_face)) plt.title(f"Score: {similarity * 100:.2f}%") else: plt.title("No Face Detected") plt.axis("off") plt.tight_layout() plt.show() if most_similar_image_path is None: raise ValueError("No faces detected in candidate images.") return most_similar_image_path, highest_similarity
使用法
比較用の画像 URL:
image_url_target = 'https://d1mnxluw9mpf9w.cloudfront.net/media/7588/4x3/1200.jpg' candidate_image_urls = [ 'https://beyondthesinglestory.wordpress.com/wp-content/uploads/2021/04/elon_musk_royal_society_crop1.jpg', 'https://cdn.britannica.com/56/199056-050-CCC44482/Jeff-Bezos-2017.jpg', 'https://cdn.britannica.com/45/188745-050-7B822E21/Richard-Branson-2003.jpg' ] most_similar_image, similarity_score = find_most_similar(image_url_target, candidate_image_urls) print(f"Most similar image: {most_similar_image}") print(f"Similarity score: {similarity_score * 100:.2f}%")
結果
結論
この例では、facenet-pytorch の顔認識機能を紹介します。 顔検出と埋め込み生成を組み合わせることで、本人確認やコンテンツ フィルタリングなど、さまざまなアプリケーション用のツールを作成できます。
以上がPython と FaceNet による顔認識の詳細内容です。詳細については、PHP 中国語 Web サイトの他の関連記事を参照してください。

ホットAIツール

Undresser.AI Undress
リアルなヌード写真を作成する AI 搭載アプリ

AI Clothes Remover
写真から衣服を削除するオンライン AI ツール。

Undress AI Tool
脱衣画像を無料で

Clothoff.io
AI衣類リムーバー

Video Face Swap
完全無料の AI 顔交換ツールを使用して、あらゆるビデオの顔を簡単に交換できます。

人気の記事

ホットツール

メモ帳++7.3.1
使いやすく無料のコードエディター

SublimeText3 中国語版
中国語版、とても使いやすい

ゼンドスタジオ 13.0.1
強力な PHP 統合開発環境

ドリームウィーバー CS6
ビジュアル Web 開発ツール

SublimeText3 Mac版
神レベルのコード編集ソフト(SublimeText3)

ホットトピック










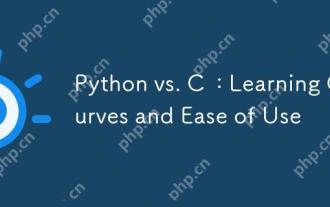
Pythonは学習と使用が簡単ですが、Cはより強力ですが複雑です。 1。Python構文は簡潔で初心者に適しています。動的なタイピングと自動メモリ管理により、使いやすくなりますが、ランタイムエラーを引き起こす可能性があります。 2.Cは、高性能アプリケーションに適した低レベルの制御と高度な機能を提供しますが、学習しきい値が高く、手動メモリとタイプの安全管理が必要です。
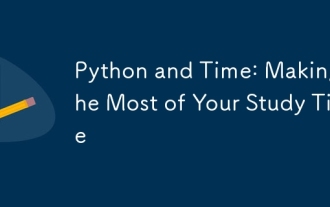
限られた時間でPythonの学習効率を最大化するには、PythonのDateTime、時間、およびスケジュールモジュールを使用できます。 1. DateTimeモジュールは、学習時間を記録および計画するために使用されます。 2。時間モジュールは、勉強と休息の時間を設定するのに役立ちます。 3.スケジュールモジュールは、毎週の学習タスクを自動的に配置します。
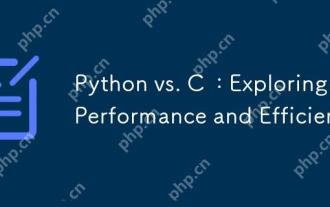
Pythonは開発効率でCよりも優れていますが、Cは実行パフォーマンスが高くなっています。 1。Pythonの簡潔な構文とリッチライブラリは、開発効率を向上させます。 2.Cのコンピレーションタイプの特性とハードウェア制御により、実行パフォーマンスが向上します。選択を行うときは、プロジェクトのニーズに基づいて開発速度と実行効率を比較検討する必要があります。
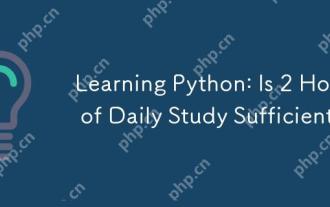
Pythonを1日2時間学ぶだけで十分ですか?それはあなたの目標と学習方法に依存します。 1)明確な学習計画を策定し、2)適切な学習リソースと方法を選択します。3)実践的な実践とレビューとレビューと統合を練習および統合し、統合すると、この期間中にPythonの基本的な知識と高度な機能を徐々に習得できます。
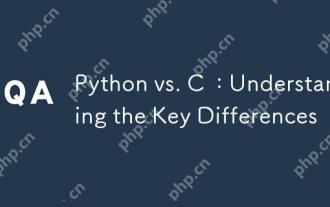
PythonとCにはそれぞれ独自の利点があり、選択はプロジェクトの要件に基づいている必要があります。 1)Pythonは、簡潔な構文と動的タイピングのため、迅速な開発とデータ処理に適しています。 2)Cは、静的なタイピングと手動メモリ管理により、高性能およびシステムプログラミングに適しています。
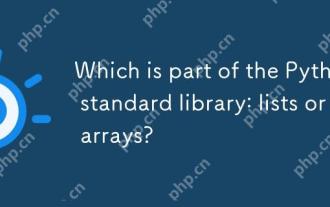
PythonListSarePartOfThestAndardarenot.liestareBuilting-in、versatile、forStoringCollectionsのpythonlistarepart。
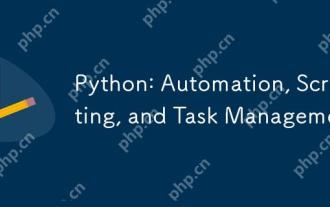
Pythonは、自動化、スクリプト、およびタスク管理に優れています。 1)自動化:OSやShutilなどの標準ライブラリを介してファイルバックアップが実現されます。 2)スクリプトの書き込み:Psutilライブラリを使用してシステムリソースを監視します。 3)タスク管理:スケジュールライブラリを使用してタスクをスケジュールします。 Pythonの使いやすさと豊富なライブラリサポートにより、これらの分野で優先ツールになります。
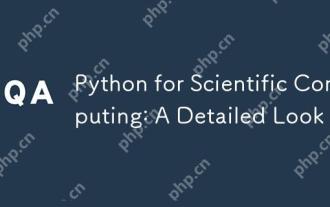
科学コンピューティングにおけるPythonのアプリケーションには、データ分析、機械学習、数値シミュレーション、視覚化が含まれます。 1.numpyは、効率的な多次元配列と数学的関数を提供します。 2。ScipyはNumpy機能を拡張し、最適化と線形代数ツールを提供します。 3. Pandasは、データ処理と分析に使用されます。 4.matplotlibは、さまざまなグラフと視覚的な結果を生成するために使用されます。
